Evaluation of experimental error in accelerometer monitoring: variation among individual animals versus variation among devices
Smart Agricultural Technology(2024)
摘要
Application of sensors is becoming prevalent in research and production settings. With increasing battery power, improved case and component durability, and consistent data connectivity, precision technologies, such as accelerometers, can help identify changes in livestock behavior. The objective of this study is to identify the variation among individual animals and among different accelerometer devices. A repeated 4×4 Latin-square design was utilized to identify differences between accelerometer, animal, and week. Twelve ewes separated into three age groups were randomly assigned to 4 different accelerometers deployed as an ear tag weekly over the course of 4 weeks. Manual behavior observations were paired to calculated accelerometer metrics and were used for training and validation dataset to predict animal behavior using random forest machine learning. Movement variation had the greatest importance in predicting behaviors. Across the four week study, differences were found for animal and week through each of the calculated metrics. Differences in accelerometers were detected for 80% of the calculated metrics. This study shows the importance to account for variation among individual animals and accelerometer devices in experimental designs.
更多查看译文
关键词
accelerometer,movement intensity,movement variation,random forest
AI 理解论文
溯源树
样例
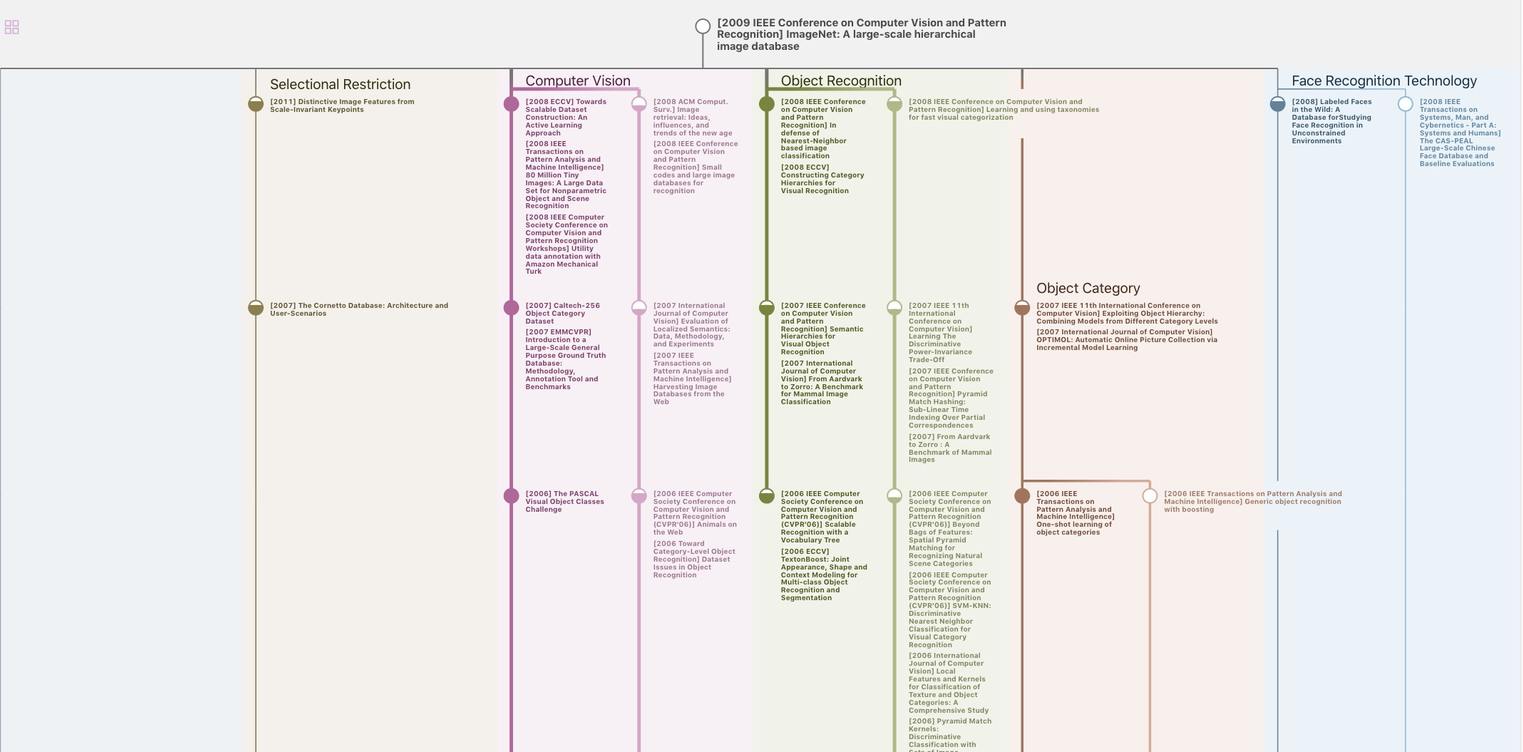
生成溯源树,研究论文发展脉络
Chat Paper
正在生成论文摘要