Machine learning based sinogram interpolation for X-ray computed tomography validated on experimental data
Precision Engineering(2024)
摘要
The data driven industry 4.0 and increasing mass-customization of additive manufacturing products require a flexible and high-throughput integration of a 100% quality inspection. While XCT has shown to be a viable non-destructive testing and measurement technique, the long acquisition times remain a stumbling block for a cost-efficient integration in production environments. To mitigate the reduced reconstruction quality from fast acquisition protocols a new sinogram interpolation algorithm is proposed. First, the method re-samples the acquired sinogram data to a new data representation which is called a spinogram. Second, the alternative data format is used to predict the missing X-ray projections using a deep learning network. We implemented this method for real XCT scans and validated on a dataset of five laser sintered objects. When applying the proposed method before the reconstruction step, less noise is present in the reconstructed and segmented volumes. This improves the feature analysis of the measured objects while preserving a lower acquistion time, hence extending the use of XCT towards fast quality inspections.
更多查看译文
关键词
Additive manufacturing,Quality control,Industrial computed tomography,Sinogram interpolation,Deep learning
AI 理解论文
溯源树
样例
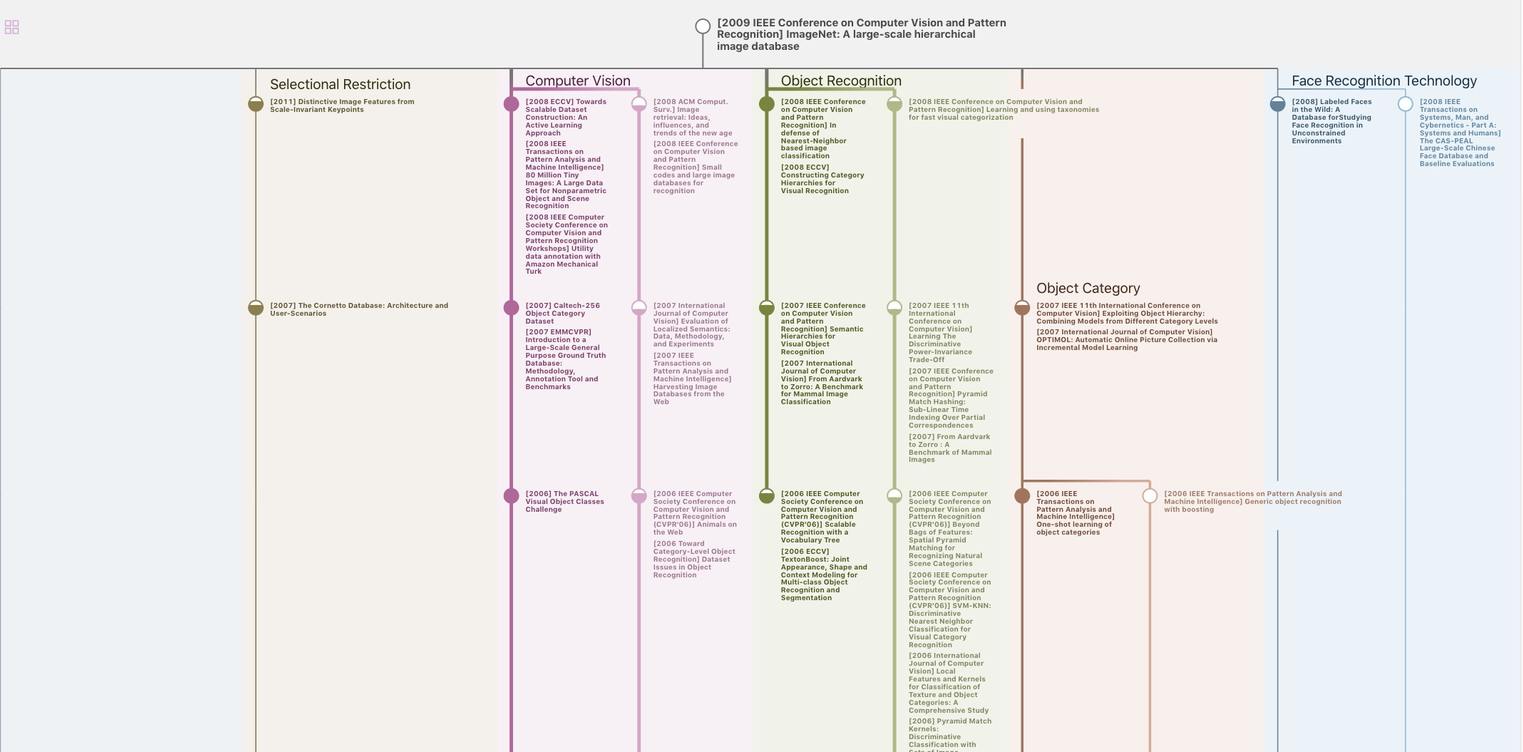
生成溯源树,研究论文发展脉络
Chat Paper
正在生成论文摘要