Temporal-aware structure-semantic-coupled graph network for traffic forecasting
Information Fusion(2024)
摘要
The spatial–temporal graph neural networks have been a critical approach to capturing the complicated spatial–temporal dependencies inherent in traffic series for more accurate forecasting. However, the issue of graph indistinguishability demands further attention, as graphs learned by existing methods tend to converge to implicit and indistinguishable representations, deviating from the genuine distribution. This issue can be attributed to the lack of three primary factors within graphs: the intrinsic graph features, the temporal-distinct features, and the node-distinct features. Aiming to address this problem, we propose a Temporal-Aware Structure-Semantic-Coupled Graph Network (TASSGN) in this paper. Firstly, we design a novel graph learning block to simultaneously learn the structural and semantic aspects of graphs, thereby capturing inherent graph features. Secondly, we propose an innovative Self-Sampling method to sample the relevant history series and present a Temporal-Aware Graphs Encoder to explicitly incorporate temporal information into graph learning and capture temporal-distinct features. Thirdly, sparse graphs are intentionally generated to capture node-distinct features. By combining these three key components together, our method is capable of overcoming the problem of graph indistinguishability and achieving state-of-the-art performances in traffic forecasting.
更多查看译文
关键词
Traffic forecasting,Spatial-temporal graph neural networks,Graph indistinguishability,Temporal-aware graphs
AI 理解论文
溯源树
样例
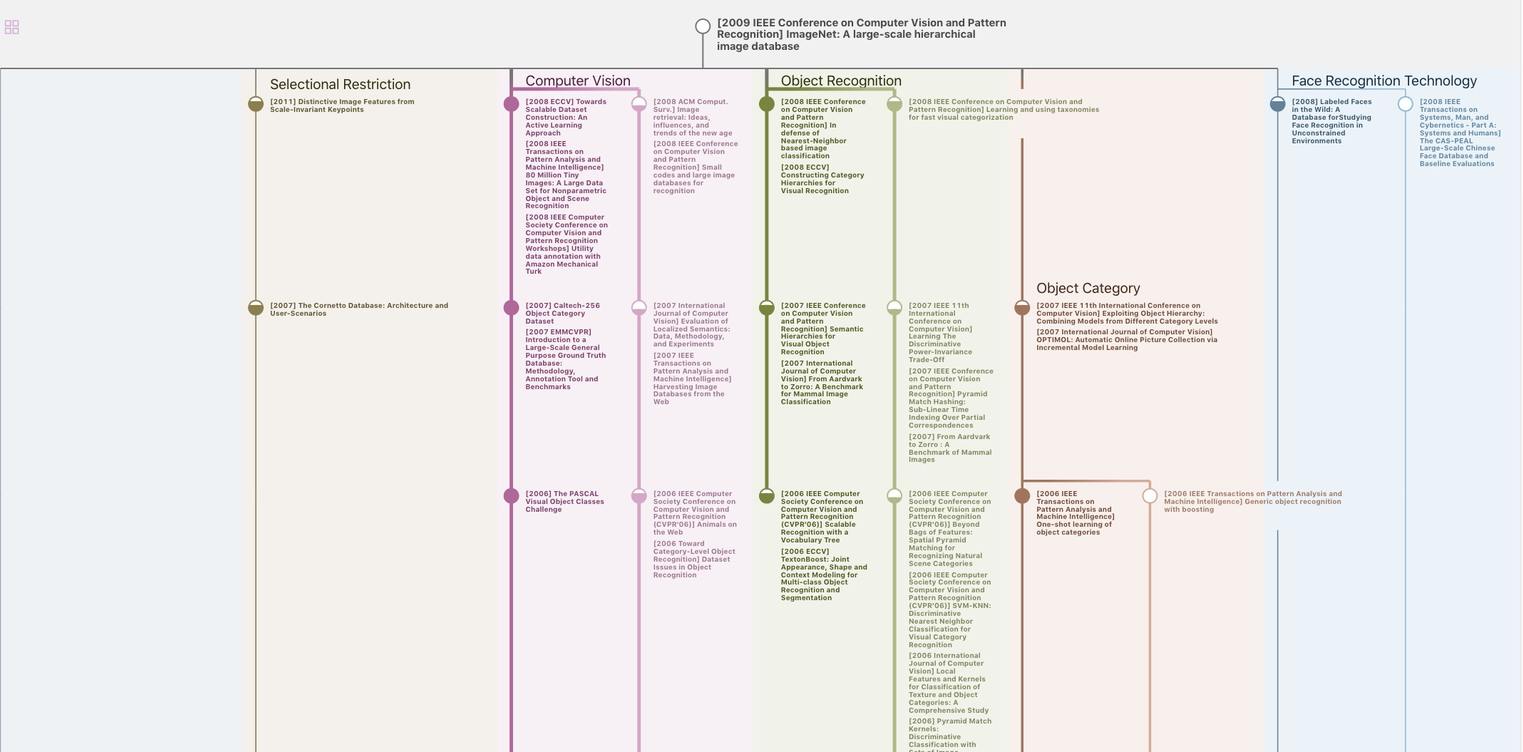
生成溯源树,研究论文发展脉络
Chat Paper
正在生成论文摘要