Transfer Learning Fusion and Stacked Auto-encoders for Viral Lung Disease Classification
New Generation Computing(2024)
摘要
The objective of this research endeavor is to identify an effective model for the classification of multiple viral respiratory diseases, encompassing COVID-19. The feature extraction phase from medical images constitutes a formidable challenge in achieving optimal disease classification outcomes. In this work, a selection of the best models among several popular transfer learning (TL) models is realized. The concatenation of the best models for better features extraction is used; the deep learning (DL) methods for deep features extraction and deep data reduction were applied for an optimal classification. This paper includes two studies, the first was applied to binary classification (COVID-19/Normal) and the second is concerned with multi-classification (COVID-19/Normal/VPneumonia). The validation of the proposed approaches is made on a big datasets: (i) binary classification 4800 COVID-19 and 4803 Normal images for the two cases Chest X-Ray (CXR) and Computed Tomography (CT) scans, and (ii) multi-class classification 3931 COVID-19, 3931 Normal, and 4273 Viral Pneumonia (VP) images for CXR. This study hypothesized that the proposed approach might be able to extract specific graphical features of COVID-19 and provide a clinical diagnosis ahead of the pathogenic test. Experimental results achieved in binary classification a high: Val_accuracy = 99.87
更多查看译文
关键词
Deep learning,Transfer learning,Fusion,Stacked auto-encoders,Classification
AI 理解论文
溯源树
样例
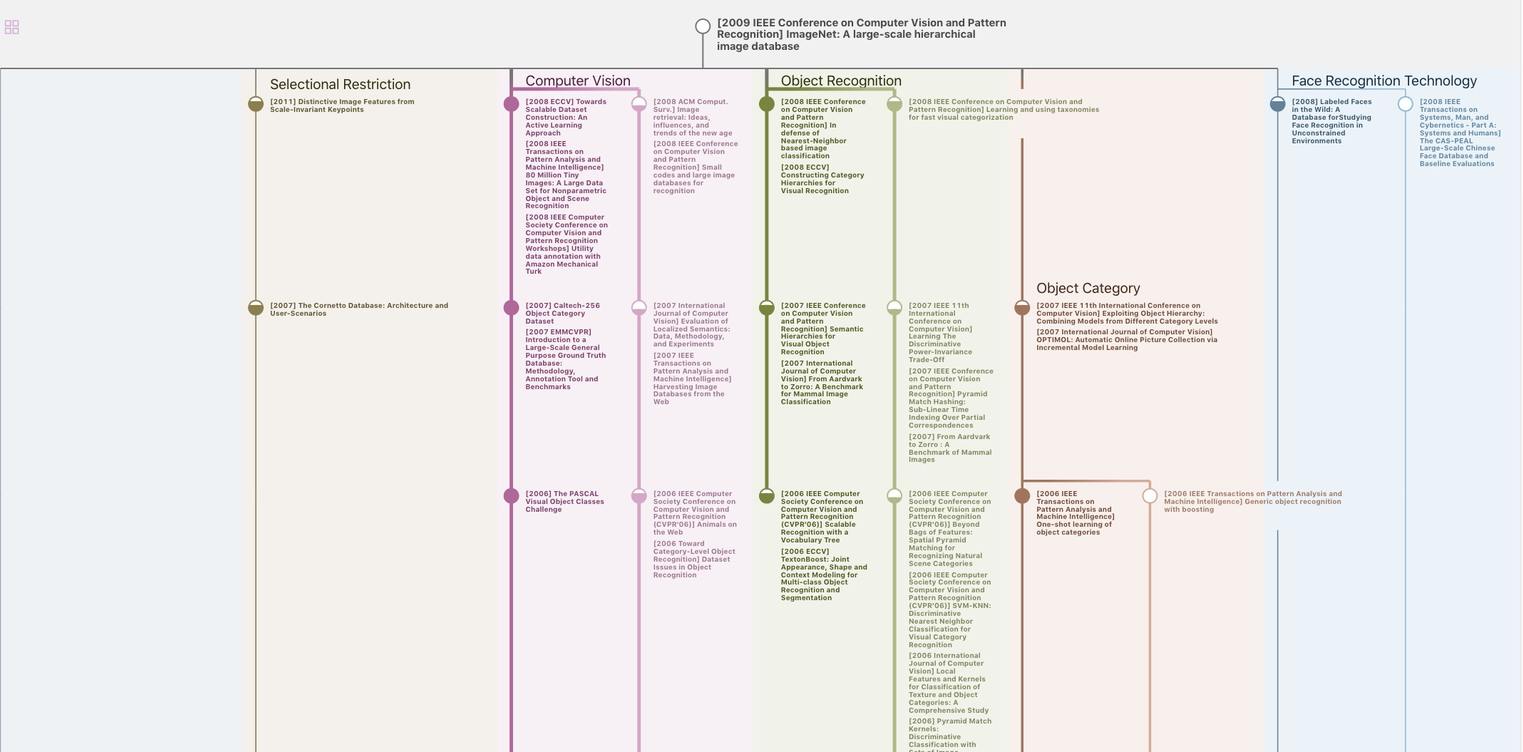
生成溯源树,研究论文发展脉络
Chat Paper
正在生成论文摘要