Model-agnostic Adversarial Example Detection via High-Frequency Amplification
Computers & Security(2024)
摘要
Image classification based on Deep Neural Networks (DNNs) is vulnerable to adversarial examples, which make the classifier output incorrect predictions. One approach to defending against this attack is to detect whether the input is an adversarial example. Unfortunately, existing adversarial example detection methods heavily rely on the underlying classifier and may fail when the classifier is upgraded. In this paper, we propose a model-agnostic detection method that leverages high-frequency signals from adversarial noises in adversarial examples and does not need interactions with the underlying classifier. We amplify redundant high-frequency signals brought by adversarial noises and represent object boundaries with these signals in an image. Our key insight is that the boundaries extracted by redundant high-frequency signals have a strong correlation with the boundaries of images in adversarial examples, while this correlation does not exist in clean images. Furthermore, adversarial examples of large images have more high-frequency signals and make adversarial detection easier on large image datasets. Experimental results show that our method has good transferability and can accurately detect various adversarial examples on different datasets.
更多查看译文
关键词
adversarial example,adversarial detection,high-frequency signal,superpixel
AI 理解论文
溯源树
样例
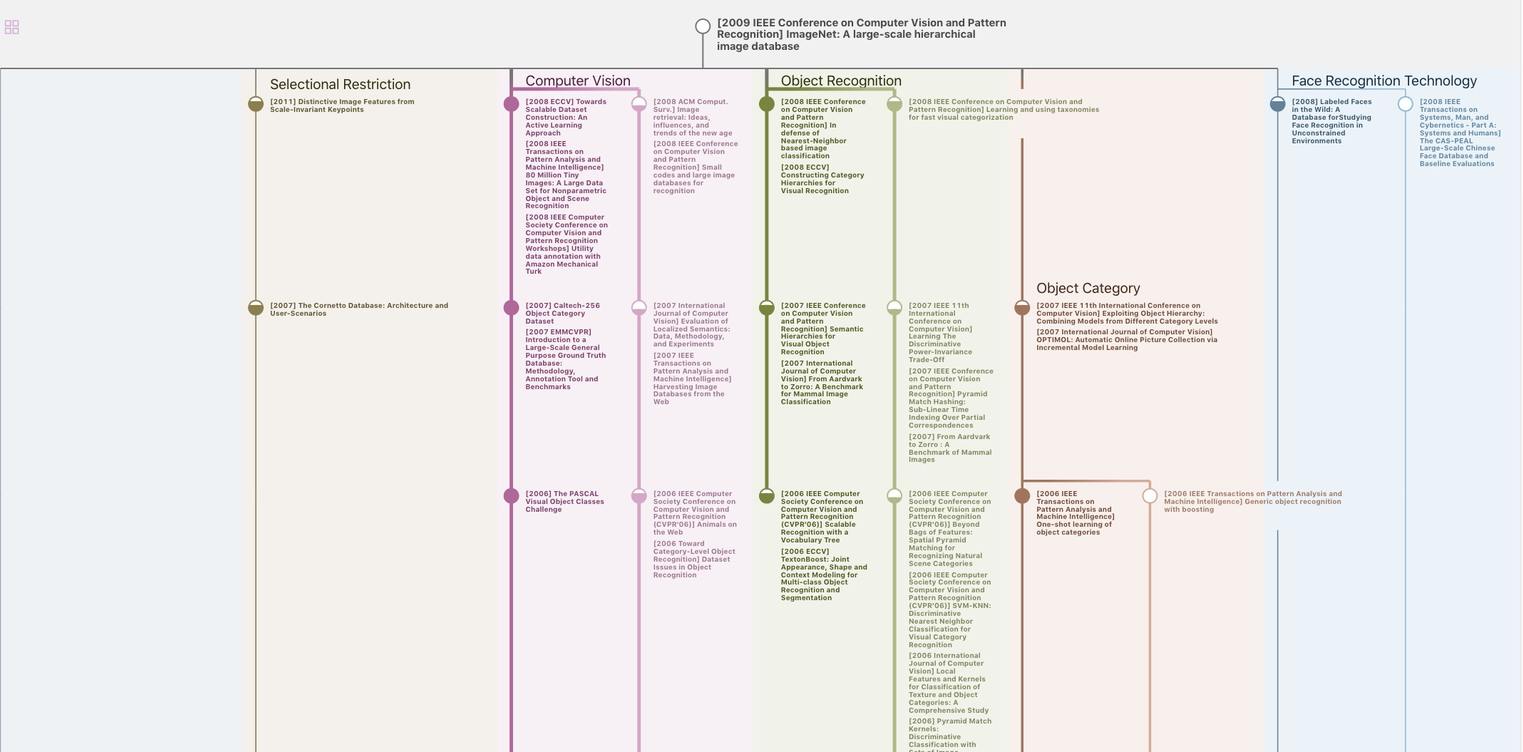
生成溯源树,研究论文发展脉络
Chat Paper
正在生成论文摘要