Kernel-based mapping of reliability in predictions for consensus modelling
Chemometrics and Intelligent Laboratory Systems(2024)
摘要
Approaches of high-level data fusion, also known as consensus, combine predictions of individual models to increase reliability and overcome limitations of single models. Consensus strategies are frequently applied in the framework of Quantitative Structure - Activity Relationships (QSARs) to reduce the uncertainties in the prediction of molecular activities and provide better accuracy of the model outcomes. However, specific regions of the chemical space may systematically be associated with low accuracy and even consensus modelling cannot improve prediction reliability through the multiple outcomes of individual models.In this study, a new heuristic metric to assess the degree of accuracy of consensus predictions in the chemical space is proposed. This metric can assist the mapping of reliability in prediction and enhance the delineation of a safe zone, where consensus predictions are expected to have better accuracy. The new metric is calculated by kernel-based potential functions and it can be used in the framework of both classification and regression consensus modelling. Four case studies, including extensive datasets for consensus modelling, were used to test the proposed approach.Results demonstrated that a potential can be associated with regions of the chemical space as a function of accuracy of consensus modelling and it can be used to enable the mapping of reliability in prediction and the definition of specific regions where predictions are expected to be more reliable.
更多查看译文
AI 理解论文
溯源树
样例
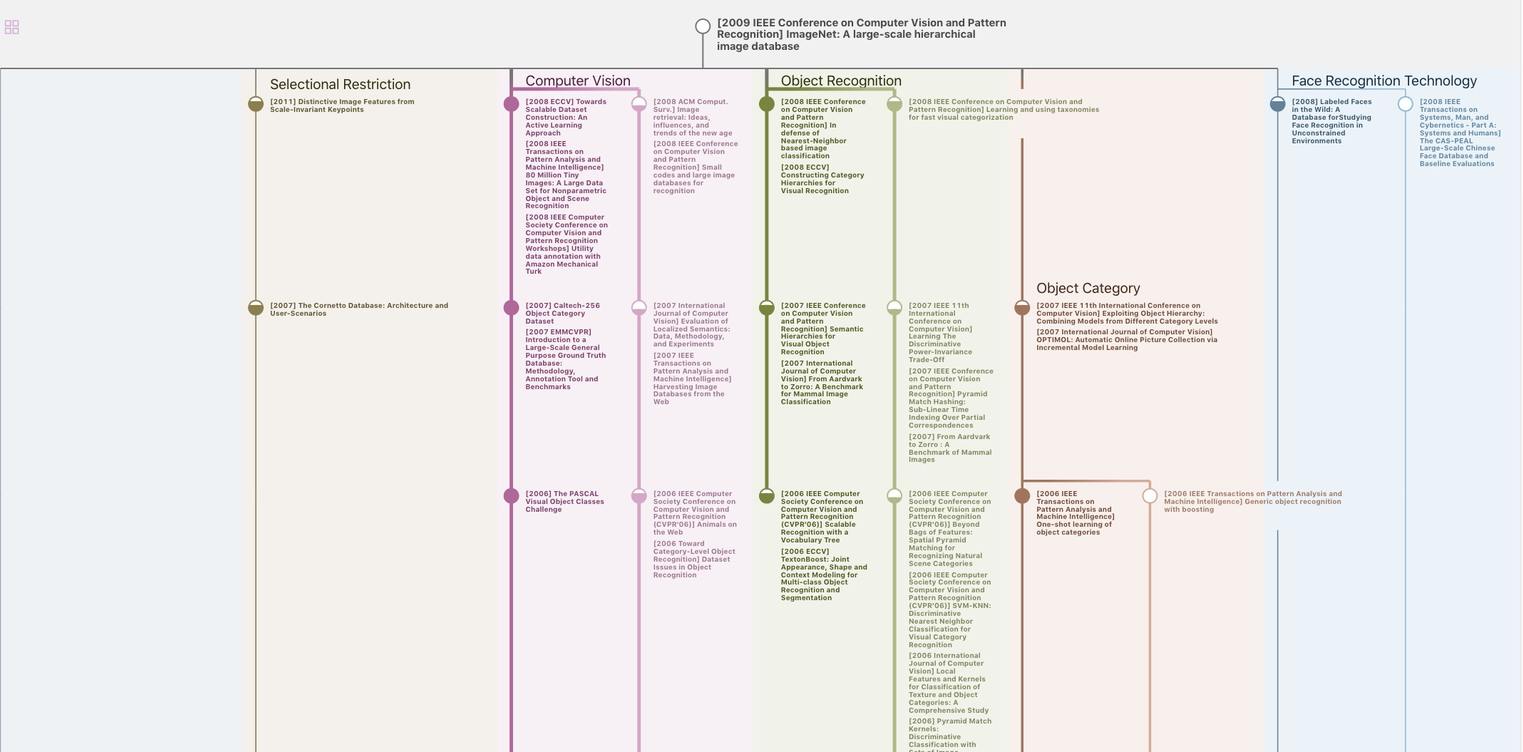
生成溯源树,研究论文发展脉络
Chat Paper
正在生成论文摘要