Explainable multimodal prediction of treatment-resistance in patients with depression leveraging brain morphometry and natural language processing
PSYCHIATRY RESEARCH(2024)
摘要
Although 20 % of patients with depression receiving treatment do not achieve remission, predicting treatmentresistant depression (TRD) remains challenging. In this study, we aimed to develop an explainable multimodal prediction model for TRD using structured electronic medical record data, brain morphometry, and natural language processing. In total, 247 patients with a new depressive episode were included. TRD-predictive models were developed based on the combination of following parameters: selected tabular dataset features, independent components-map weightings from brain T1-weighted magnetic resonance imaging (MRI), and topic probabilities from clinical notes. All models applied the extreme gradient boosting (XGBoost) algorithm via five-fold cross-validation. The model using all data sources showed the highest area under the receiver operating characteristic of 0.794, followed by models that used combined brain MRI and structured data, brain MRI and clinical notes, clinical notes and structured data, brain MRI only, structured data only, and clinical notes only (0.770, 0.762, 0.728, 0.703, 0.684, and 0.569, respectively). Classifications of TRD were driven by several predictors, such as previous exposure to antidepressants and antihypertensive medications, sensorimotor network, default mode network, and somatic symptoms. Our findings suggest that a combination of clinical data with neuroimaging and natural language processing variables improves the prediction of TRD.
更多查看译文
关键词
Treatment-resistant depression,Machine learning,Brain imaging,Natural language processing
AI 理解论文
溯源树
样例
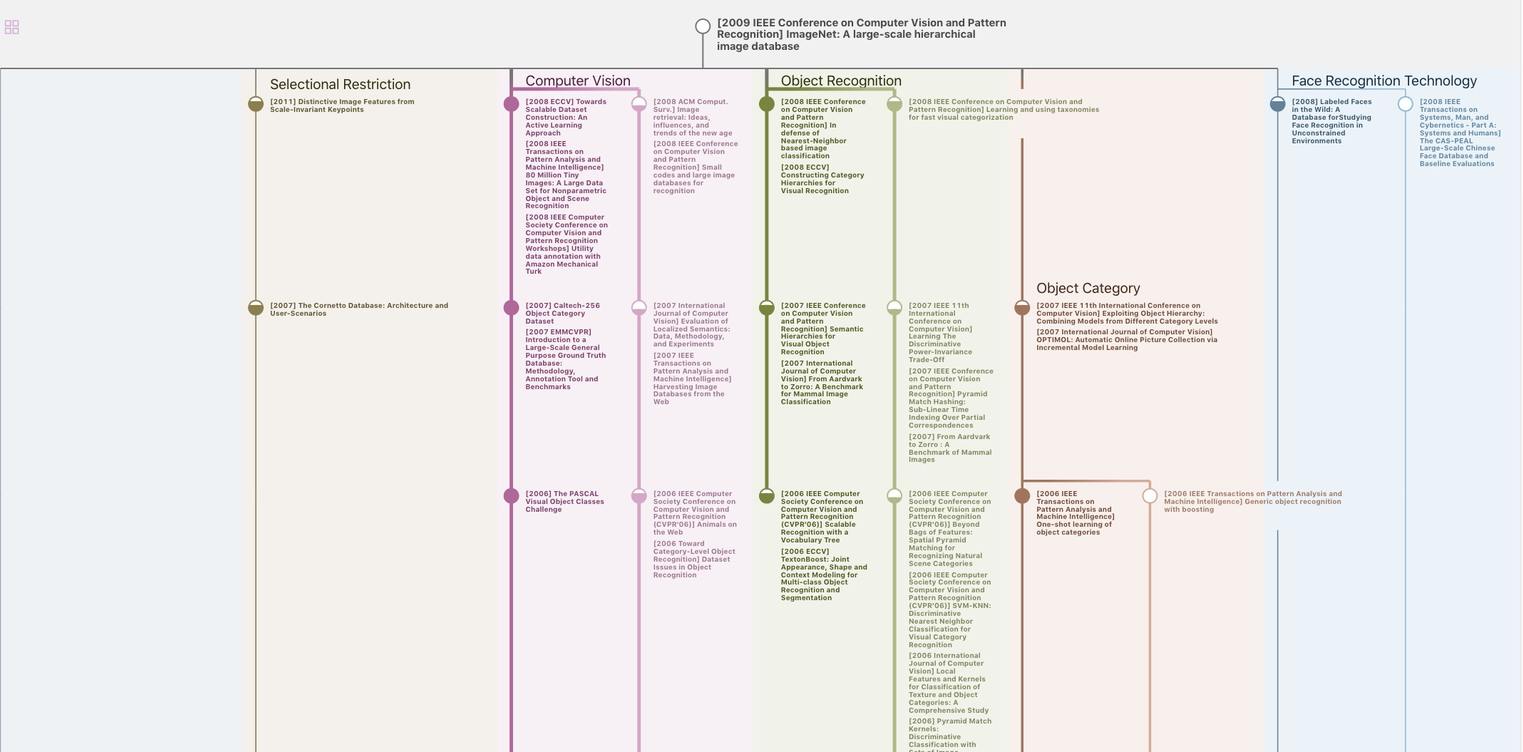
生成溯源树,研究论文发展脉络
Chat Paper
正在生成论文摘要