Estimation of ground motion parameters via multi-task deep neural networks
Natural Hazards(2024)
摘要
Ground motion parameters are crucial characteristics in earthquake warning and earthquake engineering practice. However, the existing methods are time-consuming and labor-intensive. In this study, a multi-task approach (GMP-MT) based on a hard parameter sharing strategy and single station data is proposed to improve the overall estimation accuracy by jointly optimizing the estimation of peak ground acceleration (PGA) and peak ground velocity (PGV). In addition, this study reshapes the mean squared error by adjusting the weight of the loss according to the data distribution to solve the data imbalance. The developed network structure extracts not only the seismic features from various dimensions but also the spatial–temporal correlations from large-dimensional seismic data. The designed model is trained and tested based on the global three-component seismic waveform data recorded in the STanford EArthquake Dataset. Experimental results show that the correlation coefficients of PGA and PGV are above 90
更多查看译文
关键词
Massive tectonic earthquakes,Ground motion parameter,Multi-task,Attention
AI 理解论文
溯源树
样例
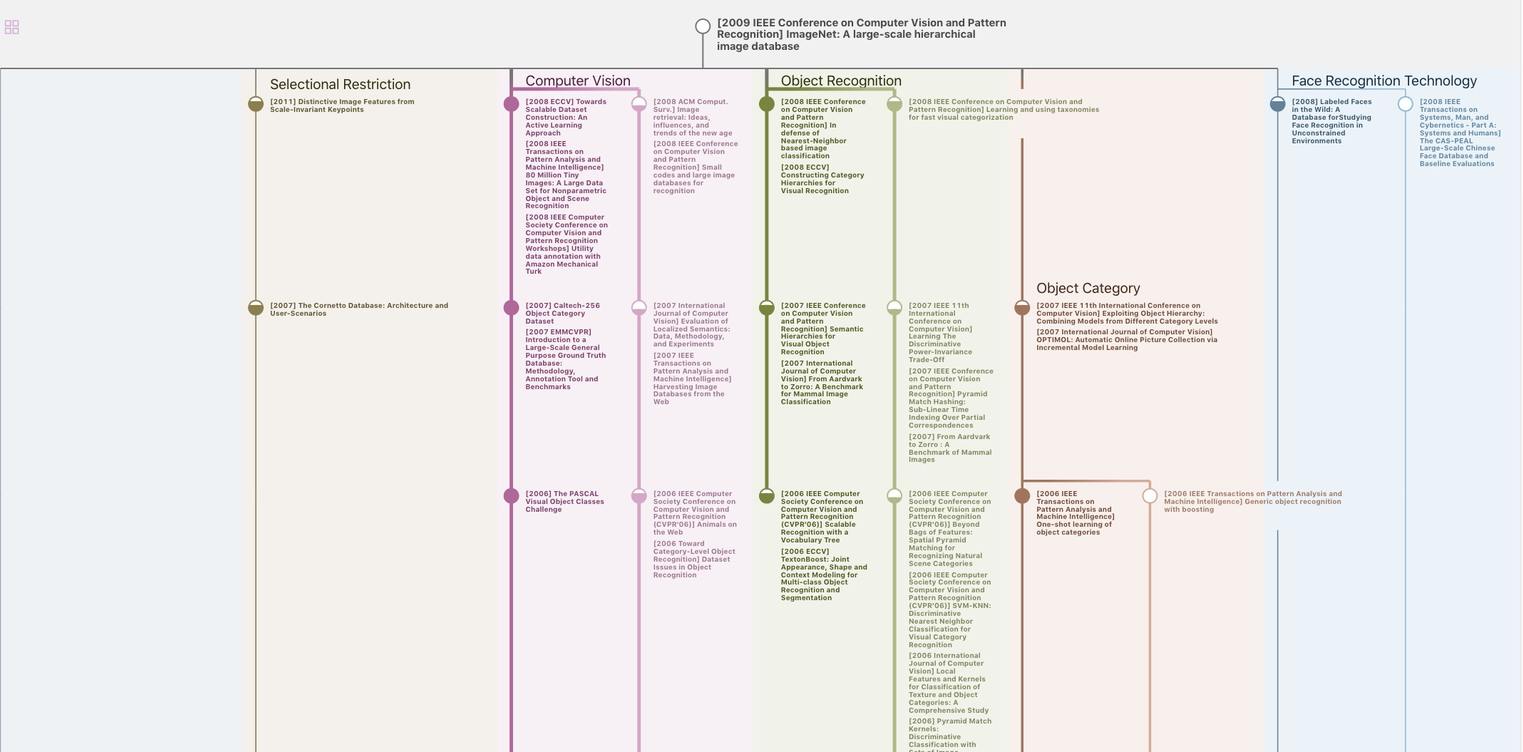
生成溯源树,研究论文发展脉络
Chat Paper
正在生成论文摘要