Coherence-based channel selection and Riemannian geometry features for magnetoencephalography decoding
Cognitive Neurodynamics(2024)
摘要
Magnetoencephalography (MEG) records the extremely weak magnetic fields on the surface of the scalp through highly sensitive sensors. Multi-channel MEG data provide higher spatial and temporal resolution when measuring brain activities, and can be applied for brain-computer interfaces as well. However, a large number of channels leads to high computational complexity and can potentially impact decoding accuracy. To improve the accuracy of MEG decoding, this paper proposes a new coherence-based channel selection method that effectively identifies task-relevant channels, reducing the presence of noisy and redundant information. Riemannian geometry is then used to extract effective features from selected channels of MEG data. Finally, MEG decoding is achieved by training a support vector machine classifier with the Radial Basis Function kernel. Experiments were conducted on two public MEG datasets to validate the effectiveness of the proposed method. The results from Dataset 1 show that Riemannian geometry achieves higher classification accuracy (compared to common spatial patterns and power spectral density) in the single-subject visual decoding task. Moreover, coherence-based channel selection significantly (P = 0.0002) outperforms the use of all channels. Moving on to Dataset 2, the results reveal that coherence-based channel selection is also significantly (P <0.0001) superior to all channels and channels around C3 and C4 in cross-session mental imagery decoding tasks. Additionally, the proposed method outperforms state-of-the-art methods in motor imagery tasks.
更多查看译文
关键词
Magnetoencephalography (MEG),Coherence,Channel selection,Riemannian geometry,Brain-computer Interface (BCI)
AI 理解论文
溯源树
样例
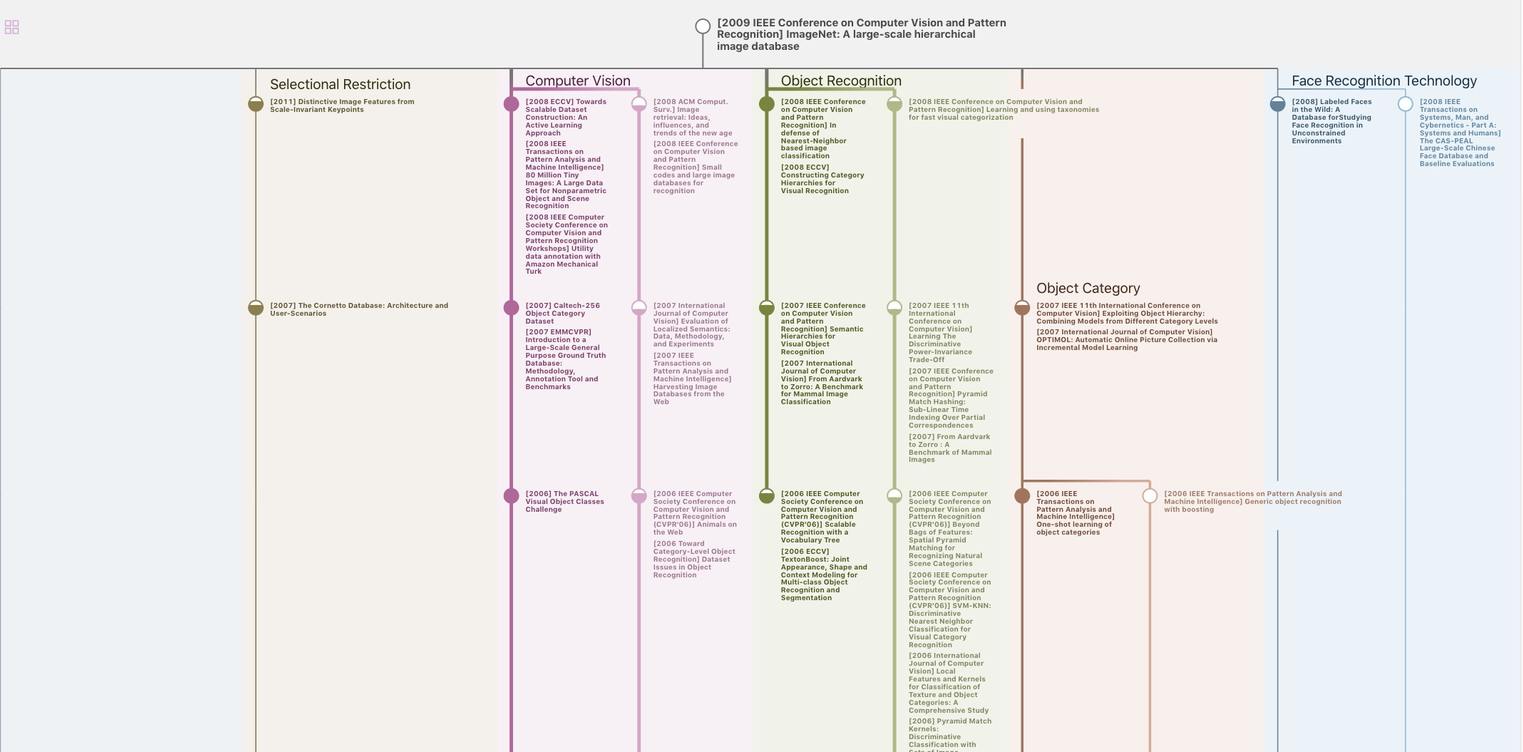
生成溯源树,研究论文发展脉络
Chat Paper
正在生成论文摘要