Cross-Modal Fusion and Progressive Decoding Network for RGB-D Salient Object Detection
International Journal of Computer Vision(2024)
摘要
Most existing RGB-D salient object detection (SOD) methods tend to achieve higher performance by integrating additional modules, such as feature enhancement and edge generation. There is no doubt that these modules will inevitably produce feature redundancy and performance degradation. To this end, we exquisitely design a cross-modal fusion and progressive decoding network (termed CPNet) to achieve RGB-D SOD tasks. The designed network structure only includes three indispensable parts: feature encoding, feature fusion and feature decoding. Specifically, in the feature encoding part, we adopt a two-stream Swin Transformer encoder to extract multi-level and multi-scale features from RGB images and depth images respectively to model global information. In the feature fusion part, we design a cross-modal attention fusion module, which can leverage the attention mechanism to fuse multi-modality and multi-level features. In the feature decoding part, we design a progressive decoder to gradually fuse low-level features and filter noise information to accurately predict salient objects. Extensive experimental results on 6 benchmarks demonstrated that our network surpasses 12 state-of-the-art methods in terms of four metrics. In addition, it is also verified that for the RGB-D SOD task, the addition of the feature enhancement module and the edge generation module is not conducive to improving the detection performance under this framework, which provides new insights into the salient object detection task. Our codes are available at https://github.com/hu-xh/CPNet .
更多查看译文
关键词
Salient object detection,Cross-modality,Multi-scale feature aggregation,Attention mechanism
AI 理解论文
溯源树
样例
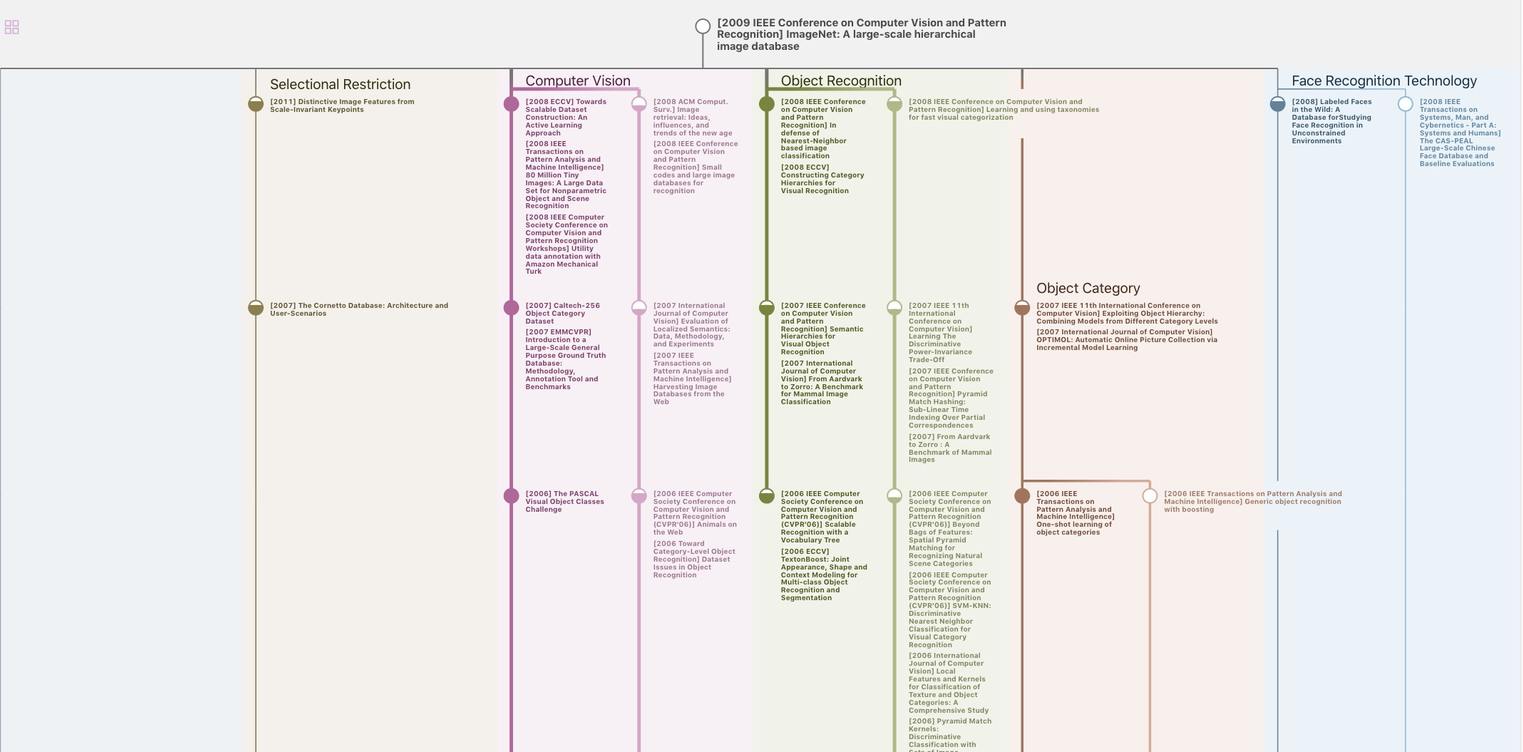
生成溯源树,研究论文发展脉络
Chat Paper
正在生成论文摘要