Data reweighting net for web fine-grained image classification
Multimedia Tools and Applications(2024)
摘要
Fine-grained visual classification (FGVC) necessitates expert knowledge,which is expensive and requires a large training sample size. Consequently, using sample data acquired through the web has emerged as a novel approach for augmenting training samples. However, the web data often includes noisy samples, leading to misclassification of deep learning models. This paper presents a a meta-learning-base method called Data Reweighting Net (DR-Net). It enables the use of small, clean meta set as a guiding mechanism to accurately learn web image datasets that contain noise. More specifically, the DR-Net fully learns from small, clean meta set to discard noisy samples and obtain clean web samples through low similarity properties. DR-Net enables classification networks to adaptively learn training sets through sample weighting, mitigating the impact of noisy labels on classification learning. Our experiments on Web-bird, Web-aircraft, Web-car, CIFAR-10, and CIFAR-100 datasets demonstrate the feasibility of our proposed method.
更多查看译文
关键词
Web images,Fine-grained visual classification,Noisy labels,Data Reweighting
AI 理解论文
溯源树
样例
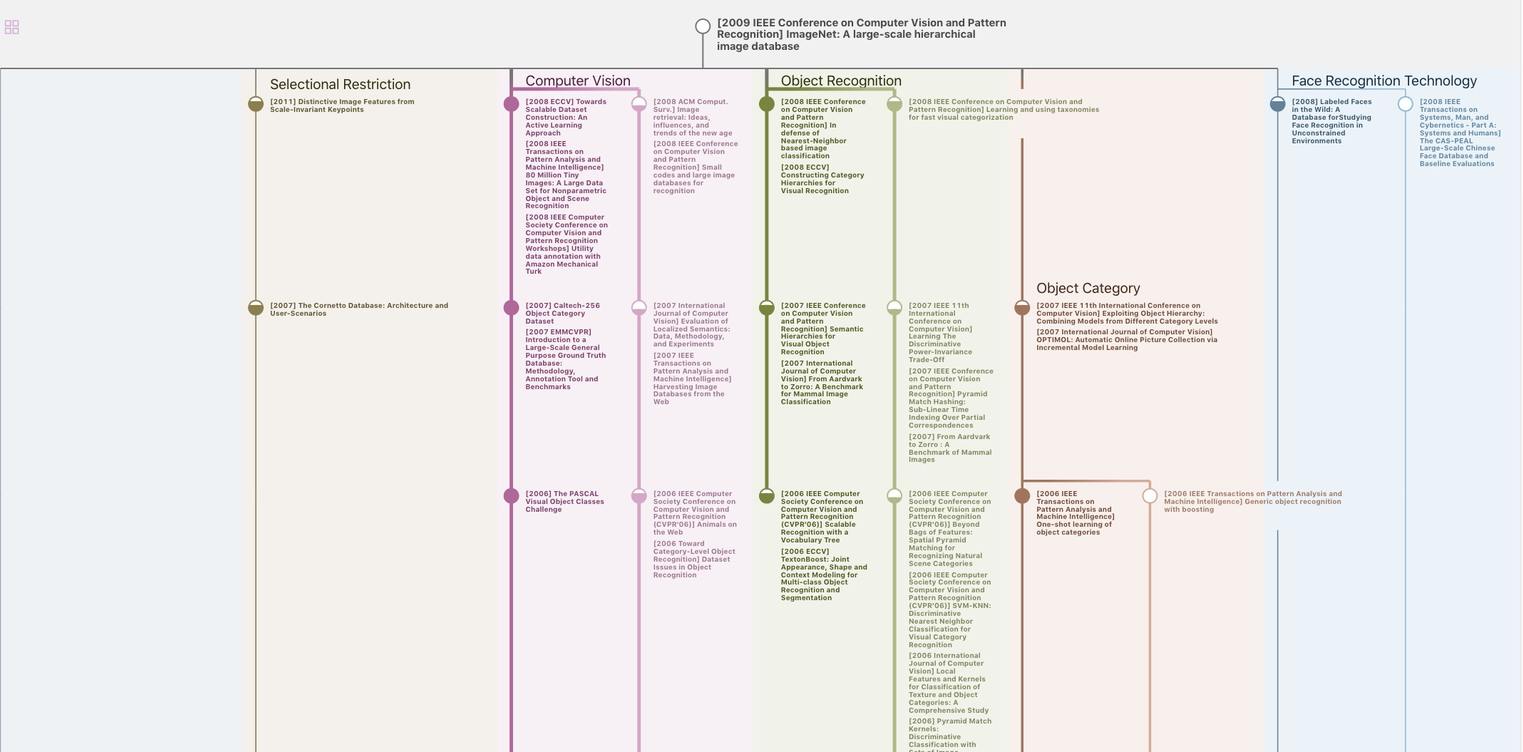
生成溯源树,研究论文发展脉络
Chat Paper
正在生成论文摘要