PCDR-DFF: multi-modal 3D object detection based on point cloud diversity representation and dual feature fusion
Neural Computing and Applications(2024)
摘要
Recently, multi-modal 3D object detection techniques based on point clouds and images have received increasing attention. However, existing methods for multi-modal feature fusion are often relatively singular, and single point cloud representation methods also have some limitations. For example, voxelization may result in the loss of fine-grained information, while 2D images lack depth information, which can restrict the accuracy of detection. Therefore, in this work, we propose a novel method for multi-modal 3D object detection based on point cloud diversity representation and dual feature fusion, PCDR-DFF, to improve the prediction accuracy of 3D object detection. Firstly, point clouds are projected to the image coordinate system and extract multi-level features of the point cloud corresponding to the image using a 2D backbone network. Then, the point clouds are jointly characterized using graphs and pillars, and the 3D features of the point clouds are extracted using graph neural networks and residual connectivity. Finally, a dual feature fusion method is designed to improve the accuracy of detection with the help of a well-designed multi-point fusion model and multi-feature fusion mechanism embedded with a spare 3D-U Net. Extensive experiments on the KITTI dataset demonstrate the effectiveness and competitiveness of our proposed models in comparison with other methods.
更多查看译文
关键词
3D Object detection,Graph neural networks,Multi-modal,Point cloud
AI 理解论文
溯源树
样例
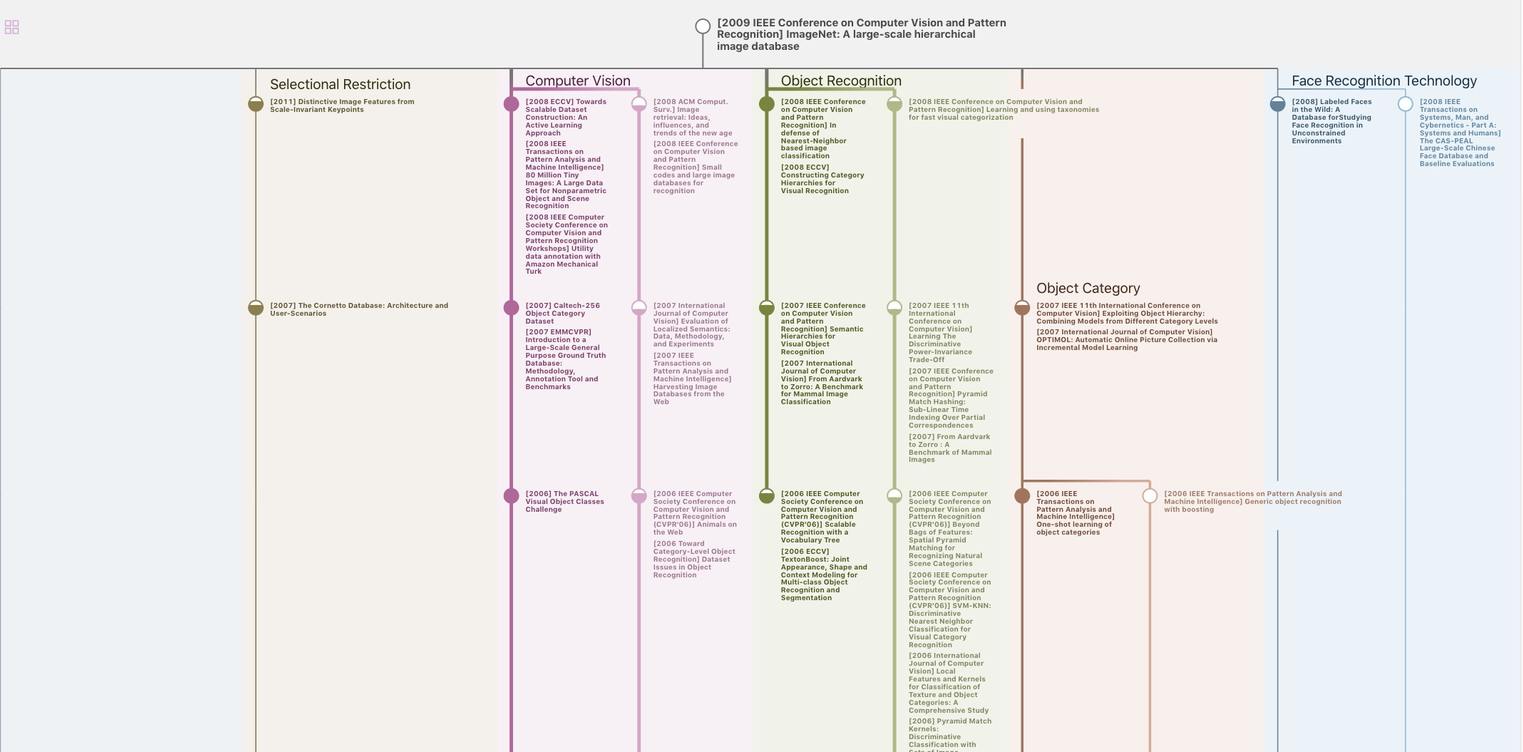
生成溯源树,研究论文发展脉络
Chat Paper
正在生成论文摘要