Comparison of simulation and predictive efficacy for hemorrhagic fever with renal syndrome incidence in mainland China based on five time series models.
Frontiers in public health(2024)
摘要
Background:Hemorrhagic fever with renal syndrome (HFRS) is a zoonotic infectious disease commonly found in Asia and Europe, characterized by fever, hemorrhage, shock, and renal failure. China is the most severely affected region, necessitating an analysis of the temporal incidence patterns in the country.
Methods:We employed Autoregressive Integrated Moving Average (ARIMA), Long Short-Term Memory (LSTM), Convolutional Neural Network (CNN), Nonlinear AutoRegressive with eXogenous inputs (NARX), and a hybrid CNN-LSTM model to model and forecast time series data spanning from January 2009 to November 2023 in the mainland China. By comparing the simulated performance of these models on training and testing sets, we determined the most suitable model.
Results:Overall, the CNN-LSTM model demonstrated optimal fitting performance (with Root Mean Square Error (RMSE), Mean Absolute Percentage Error (MAPE), and Mean Absolute Error (MAE) of 93.77/270.66, 7.59%/38.96%, and 64.37/189.73 for the training and testing sets, respectively, lower than those of individual CNN or LSTM models).
Conclusion:The hybrid CNN-LSTM model seamlessly integrates CNN's data feature extraction and LSTM's recurrent prediction capabilities, rendering it theoretically applicable for simulating diverse distributed time series data. We recommend that the CNN-LSTM model be considered as a valuable time series analysis tool for disease prediction by policy-makers.
更多查看译文
关键词
HFRS,ARIMA,LSTM,CNN,NARX
AI 理解论文
溯源树
样例
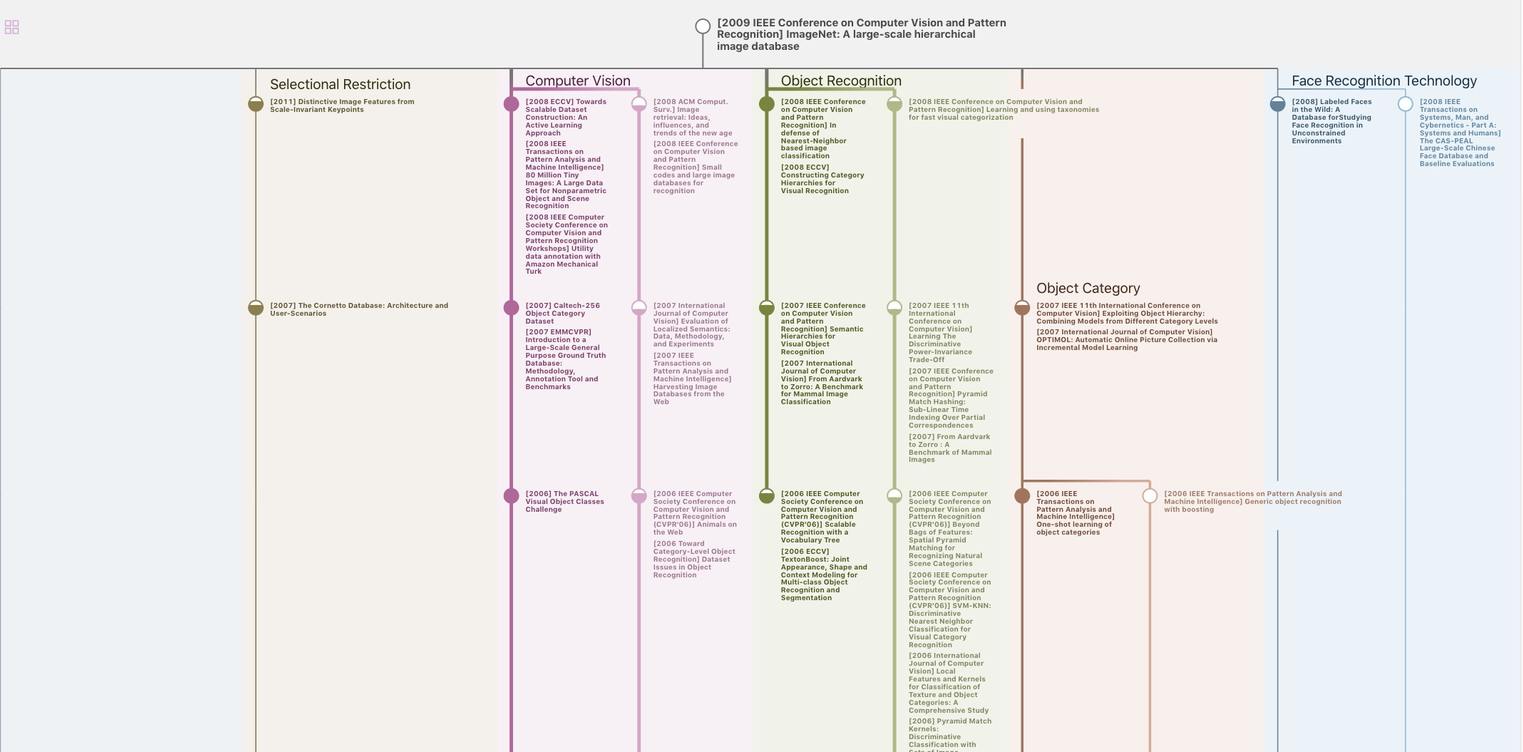
生成溯源树,研究论文发展脉络
Chat Paper
正在生成论文摘要