A novel machine learning method to exploit EBSD and nanoindentation for TRIP steels microstructures analysis
MATERIALS & DESIGN(2024)
摘要
The recognition of phases and microstructures in TRIP-assisted bainitic-ferritic steels is challenging and requires sophisticated techniques to gain insights and reveal mechanical features with nanoscale precision. EBSD and nanoindentation have been employed to assess the surface composition and their properties within a reporting depth of 30 nm. Correlative mechanical microscopy and data science were used to overcome the shortcomings associated with the lack of an inclusive solution that combines the metadata from both techniques. A modular methodology is presented, which involves routines for exploiting structural and mechanical data via reproducible Machine Learning models (code and data are shared). The approach is structured to facilitate reuse by research community for correlating characterization mapping data, not limited to nanoindentation and EBSD. Gaussian mixture models are adopted to extract mechanical phases utilizing the nanomechanical properties. The K-means++ method is used for the first time to mine information from Inverse Polar Figure (IPF) mapping about anisotropy and to extract the knowledge from images for each grain, including grain coordinates and size. Moreover, k-nearest-neighbours regression was used to perform data imputation to fill in the values of descriptors related to missing coordinates relative to those of nanoindentation, grain boundary, EBSD phase, and EBSD anisotropy maps.
更多查看译文
关键词
Machine learning,High-speed nanoindentation mapping,Correlative analysis,Steel,Microstructure
AI 理解论文
溯源树
样例
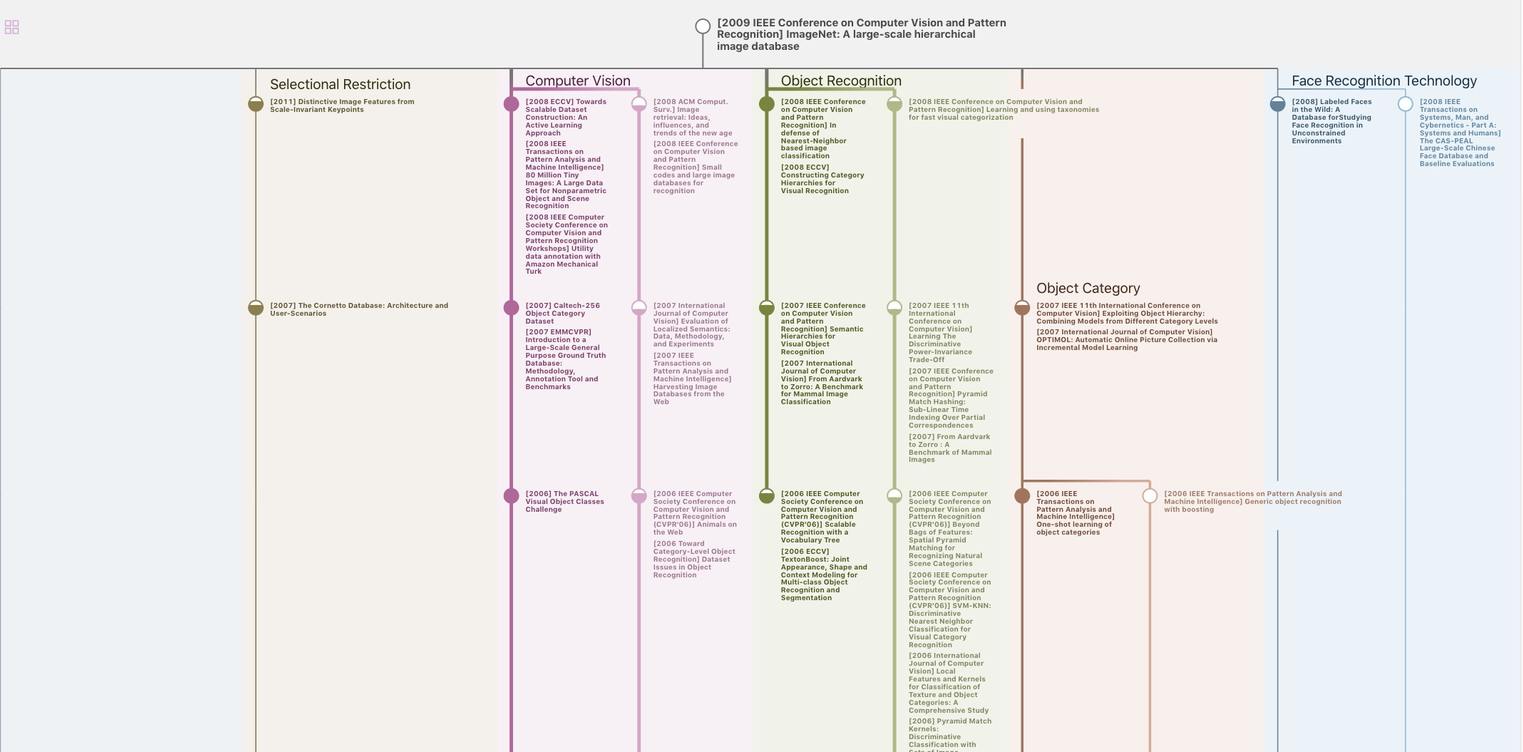
生成溯源树,研究论文发展脉络
Chat Paper
正在生成论文摘要