Convolution Driven Vision Transformer for the Prediction of Mild Cognitive Impairment to Alzheimer's disease Progression.
2024 IEEE International Conference on Consumer Electronics (ICCE)(2024)
摘要
Alzheimer’s disease (AD) poses a significant challenge to global health, affecting over 50 million individuals worldwide with no current cure. Early identification and treatment are vital to mitigate its impact on memory, language, and actions. Recent strides in artificial intelligence (AI) and neural networks, particularly Vision Transformers (ViT), show promise in enhancing early AD diagnosis through advanced medical image analysis. ViT, surpassing conventional Convolutional Neural Networks (CNNs), proves adept at discerning subtle changes in brain structure and function, crucial for early AD detection. However, ViT models face challenges like iterative attention biases hindering effective target class focus. Our proposed solution incorporates an augmenting method and convolution patch embedding, addressing these limitations, and improving patch extraction efficiency while preserving original dimensions. Utilizing the Alzheimer’s Disease Neuroimaging Initiative (ADNI) dataset, our comprehensive study focuses on stable and convertible mild cognitive impairment (MCIs and MCIc) to AD progression. The proposed method achieves a diagnostic accuracy of 91.35%, sensitivity of 91.09%, and specificity of 91.63%, surpassing existing methodologies. This highlights the practical viability and effectiveness of our approach in AD diagnosis. In conclusion, early and accurate AD detection is crucial for patient outcomes. Our innovative methods showcase the potential of ViT in brain image analysis, paving the way for future advancements in medical imaging research.
更多查看译文
关键词
Alzheimer’s disease,CNN,vision transformer,SA,GPSA,sMRI,classification
AI 理解论文
溯源树
样例
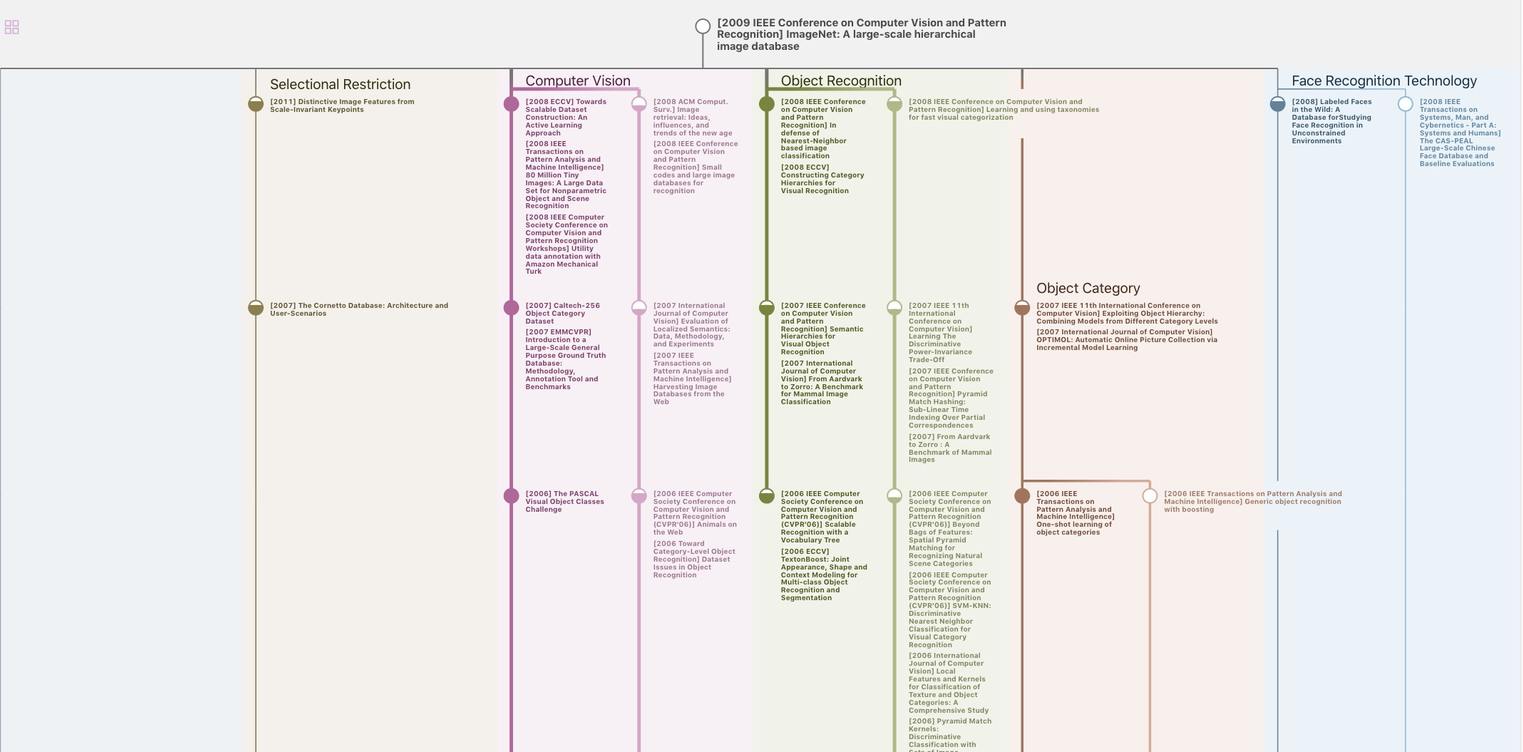
生成溯源树,研究论文发展脉络
Chat Paper
正在生成论文摘要