SeGCN: A Semantic-Aware Graph Convolutional Network for UAV Geo-Localization.
IEEE J. Sel. Top. Appl. Earth Obs. Remote. Sens.(2024)
摘要
Cross-view geo-localization via scene matching is crucial in unmanned aerial vehicle (UAV) systems in global navigation satellite system (GNSS) denial environment. However, images in the same scene may undergo geometric distortion and occlusion due to difference in capture viewpoint, time and platform. The existing methods mainly extract consistent features between images by CNNs, while ignoring the semantic distribution and structural information of the objects. Aiming at addressing this issue, we introduce a semantic-aware graph convolutional network (SeGCN). To improve consistent representation of object features from different viewpoints, potential semantic features are inferred via cross-attention of image context. Then, for exploring the structural information of objects, SeGCN performs graph convolution on graph structures constructed from the same semantic features. Finally, the composite features generated by SeGCN and backbone are utilized for scene matching. Comprehensive experiments conducted on the University-1652 and SUES-200 benchmarks establish that the proposed approach attains the highest levels of accuracy in both localization and navigation tasks. Furthermore, we conducted localization simulation experiments on our real UAV datasets, confirming the effectiveness of SeGCN in real world application scenarios.
更多查看译文
关键词
GCNs,geo-localization,sementic inference,transformer,UAV navigation
AI 理解论文
溯源树
样例
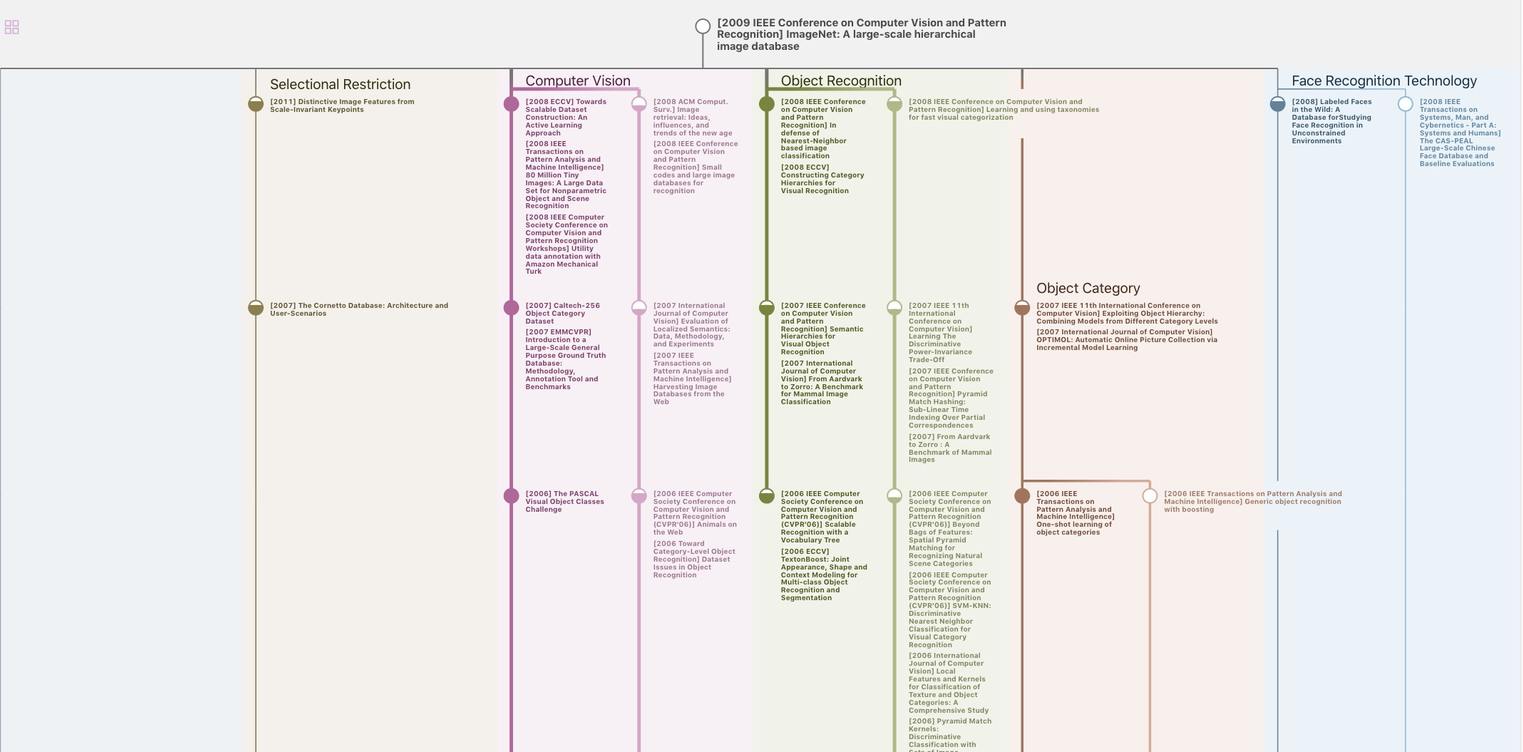
生成溯源树,研究论文发展脉络
Chat Paper
正在生成论文摘要