Federated Domain Separation for Distributed Forecasting of Non-IID Household Loads
IEEE Transactions on Smart Grid(2024)
摘要
Household load forecasting is increasingly essential since it enables various demand-side management applications. The federated learning approach is becoming popular for its advantages in fully using different households’ load data with privacy preservation. However, due to the non-independent and identically distributed (non-IID) characteristic of each household’s local data, the knowledge acquired by local training may have a strong bias. It can introduce contamination and make the global model vulnerable if locally trained models are simply aggregated as traditional FL methods do. To this end, we develop a novel framework that integrates federated domain separation to alleviate the negative effects caused by non-IID data. Specifically, we divide the acquired knowledge into the useful part and potentially contaminating part. By acquiring the former and removing the latter through a well-designed algorithm, a more anti-contamination and more personalized FL model can be expected. Compared to current post-processing personalization methods, the proposed framework can avoid global knowledge forgetting, thus achieving more comprehensive knowledge utilization to give more accurate results. Extensive comparison experiments with benchmarking methods are conducted on a publicly available dataset to validate the superiority of the proposed framework, while a variety of ablation experiments prove the effectiveness of all inner components.
更多查看译文
关键词
Household load forecasting,federated learning,Non-IID data,domain separation,personalization
AI 理解论文
溯源树
样例
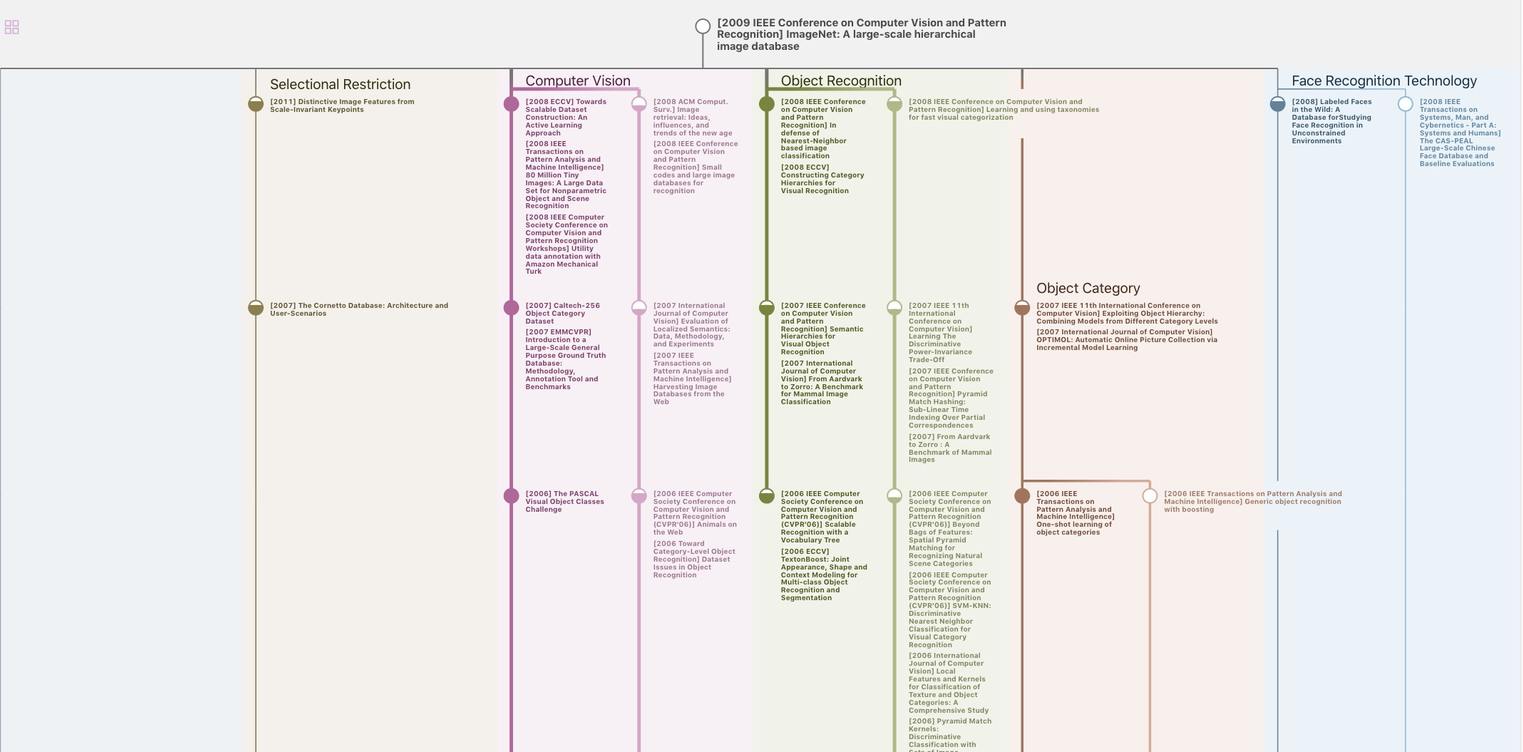
生成溯源树,研究论文发展脉络
Chat Paper
正在生成论文摘要