Direct Edge-to-Edge Attention-Based Multiple Representation Latent Feature Transfer Learning
IEEE Transactions on Automation Science and Engineering(2024)
摘要
Deploying a large number of smart cameras and training their models is a very time-consuming and labor-intensive process. Although there have been studies that utilized direct edge-to-edge (e2e) feature transfer learning without requiring help from centralized servers, they do not make good use of advanced latent features and even require constant adjustment of certain hyperparameters. This not only takes a lot of time for initial model training but also does not meet one of the key characteristics of the Internet of Thing, i.e., reduced human intervention. Therefore, we propose a Lightweight Multi-representation Attention-based Residual (LiMAR) module to reduce the computational load on an edge device via its lightweight architecture, which can extract multi-representation and diverse features to make the best use of the latent features for transfer learning in a direct e2e manner. In addition, we also propose a Lightweight Joint-distribution Autonomous Domain Adaptation (LiJADA) module to further reduce the load of model training and reduce the time required for camera deployment and the necessity of human intervention. The experimental results show that incorporating these two modules with one-to-many transfer can improve the total accuracy by 6.30% compared to a baseline study, yet with 75% less transmission data. In addition, the two modules working with many-to-one transfer learning can reduce the transmission cost by 83.33% and improve the total accuracy by 5.10%.
Note to Practitioners
—This study was motivated by the practical problem of enabling an unmanned application to efficiently learn its initial models. This problem is important for practitioners in unmanned areas who aim to reduce the effort required for system deployment by utilizing direct edge-device to edge-device (or edge-to-edge) model transfer learning. Existing approaches mainly rely on basic feature-level transfer learning, and they often require constant adjustment of hyperparameters to achieve optimal model performance. To tackle these challenges, we propose a novel approach that involves transferring multi-representation features with automatic hyper-parameter adjustment. This approach can improve knowledge reusability across different environments, and it is also important for real-life scenarios. Preliminary evaluations suggest that the approach is feasible and can achieve knowledge reuse while reducing data transmission costs. However, since we still use raw input images for matching the source and target cameras rather than using features for matching, this may limit non-source-free applications. To address this limitation, incorporating model-based transfer in the next-phase implementation can mitigate the drawback.
更多查看译文
关键词
Direct edge-to-edge,many-to-many transfer learning,deep learning,advanced latent features,multi-representation,edge computing,Internet of Things
AI 理解论文
溯源树
样例
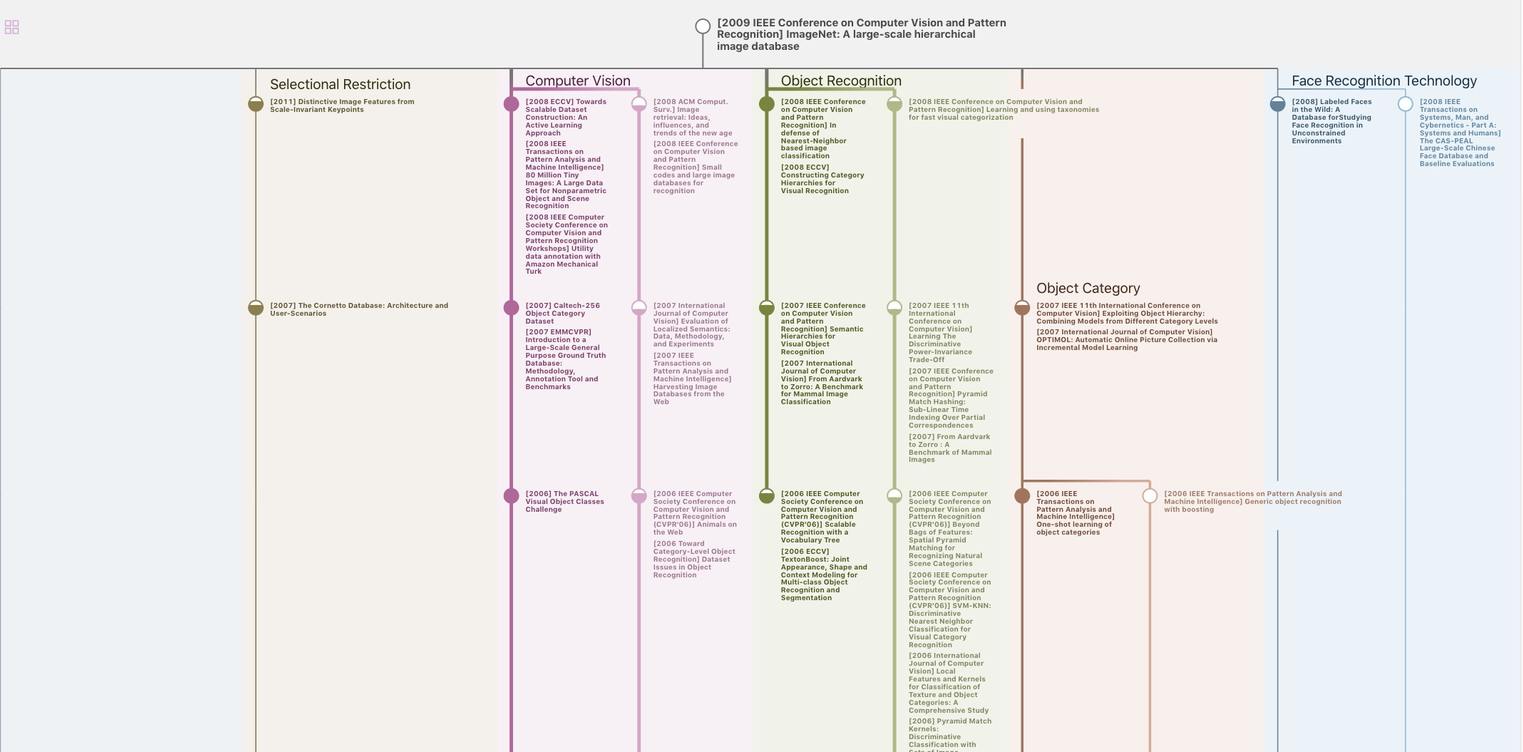
生成溯源树,研究论文发展脉络
Chat Paper
正在生成论文摘要