STFE: A Comprehensive Video-Based Person Re-Identification Network Based on Spatio-Temporal Feature Enhancement
IEEE Transactions on Multimedia(2024)
摘要
Video-based person re-identification (Re-ID) is designed to retrieve target pedestrians in video sequences under non-overlapping cameras. At present, mainstream approaches post-process the feature map extracted by the convolutional neural network backbone to obtain a global representation or a fine-grained local representation for higher accuracy. However, they still suffer from challenges, such as information loss for global-based methods and spatio-temporal feature fragmentation for local-based methods. To alleviate these problems, this paper proposes a Spatio-Temporal Feature Enhancement (STFE) network from a spatio-temporal comprehensive perspective, combining the advantages of the above methods to obtain more comprehensive information from video tracklets. STFE consists of two main modules: Feature Space Projection Module (FSPM) and Global Low-frequency Enhancement Module (GLEM). FSPM mathematically converts continuous video information into a discrete feature space and selectively retains more useful information, thus avoiding spatio-temporal information loss. Meanwhile, FSPM applies global features instead of dividing feature maps spatially, thereby avoiding spatio-temporal feature fragmentation. In addition, GLEM which is based on transformer, acts as a broadband low-pass filter to mine richer global comprehensive information. Finally, by combining FSPM with GLEM, STFE can obtain spatio-temporal comprehensive video representation. Extensive experiments were conducted on two widely-used video Re-ID datasets. The experimental results verify our idea and demonstrate the effectiveness of the proposed STFE with 95.5% Rank-1 accuracy on MARS benchmarks, which surpasses previous
state-of-the-arts
by a large margin of +4%.
更多查看译文
关键词
video-based person re-identification,spatio-temporal information learning,motion feature extraction
AI 理解论文
溯源树
样例
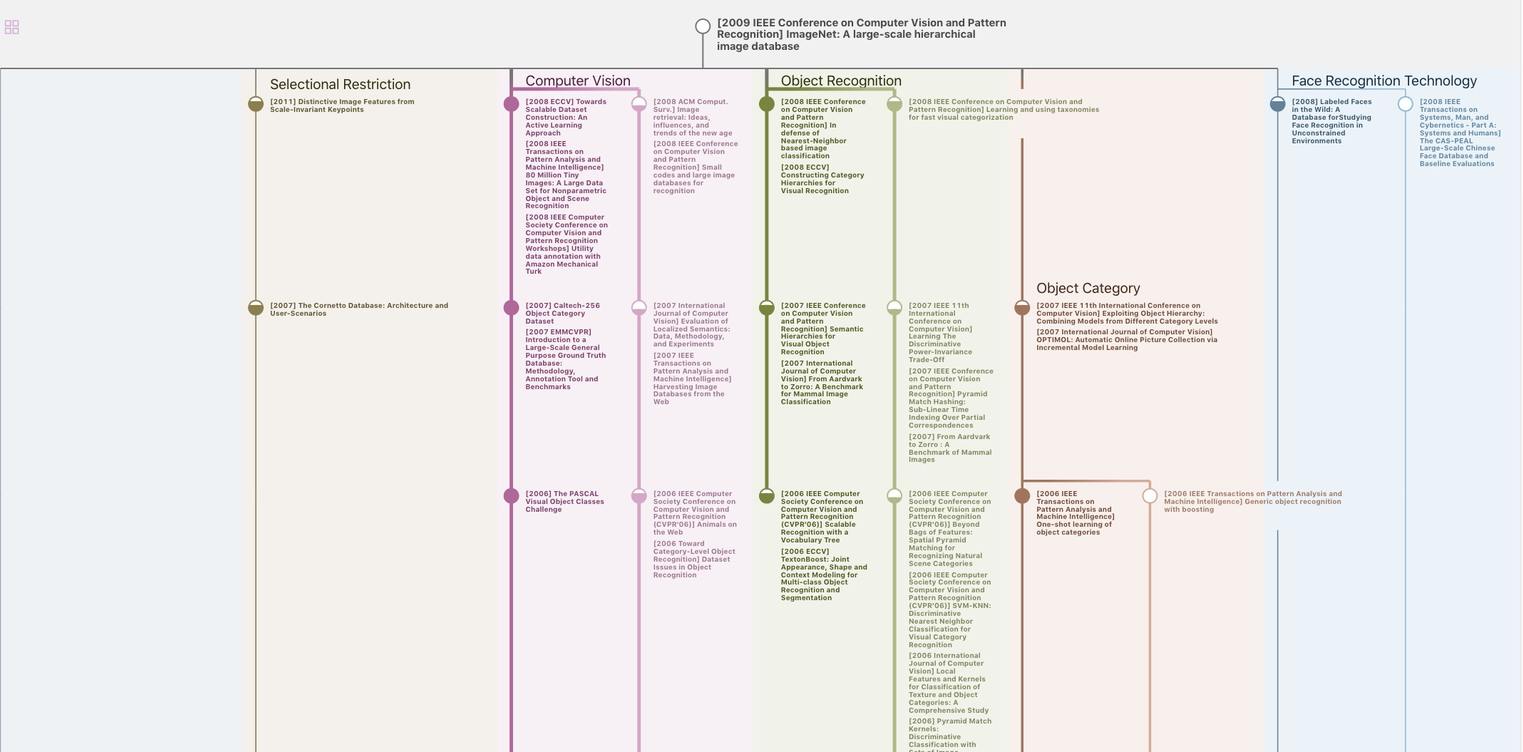
生成溯源树,研究论文发展脉络
Chat Paper
正在生成论文摘要