StableSwap: Stable Face Swapping in a Shared and Controllable Latent Space
IEEE Transactions on Multimedia(2024)
摘要
Person-agnostic face swapping has gained significant attention in recent years, as it offers the potential to enhance various real-world applications by combining high fidelity and identity consistency. However, conventional face swapping methods often rely on intricate adjustments of different loss functions, leading to instability during both the training and inference stages. In this work, we propose a simple yet effective framework named
StableSwap
with a reversible autoencoder to modify the face in a shared latent space. Our approach capitalizes on the information-rich image latent codes to tackle the challenges of complex editing tasks, utilizing the abundant details present in both the source and target faces. To ensure an expressive and robust latent space, we employ a latent alignment approach with perceptual and adversarial losses to optimize the autoencoder. Additionally, we devise a multi-stage identity injection module that samples multiple features with different facial priors and incorporates them to guide the latent image manipulation. By leveraging attention-based blocks, we fuse these futures and update the latent code in a mask-conditioned manner. Both quantitative and qualitative results on the mainstream benchmarks demonstrate that our StableSwap generates competitive identity-consistent swapped faces compared with state-of-the-art methods. Our method outperforms previous approaches in terms of ID Retrieval (98.68) and FID (2.49), while also exhibiting enhanced stability during model training. Beyond this, our model achieves region-controllable face swapping with the capability to perform more fine-grained operations in latent space.
更多查看译文
关键词
Face Swapping,Latent Manipulation,Controllable Editing
AI 理解论文
溯源树
样例
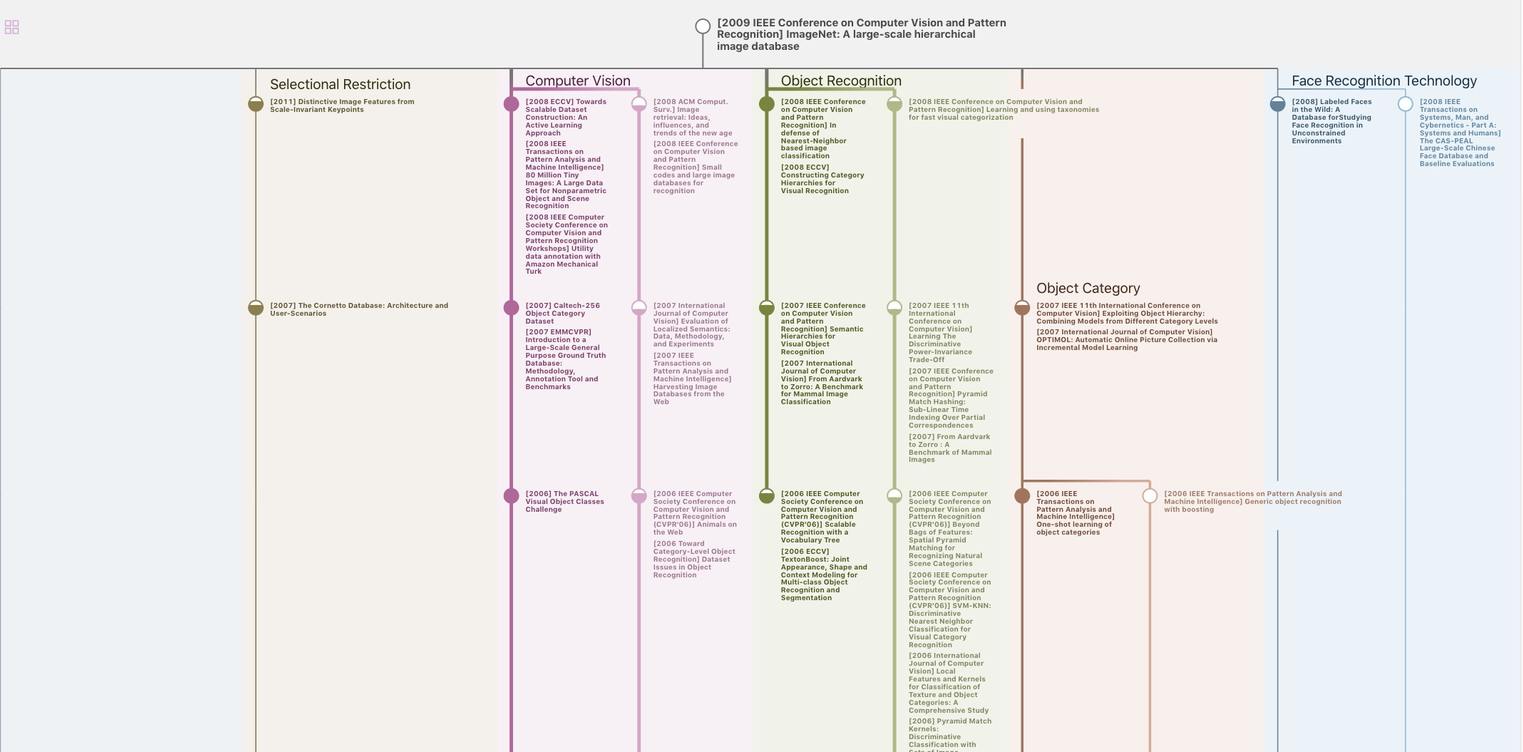
生成溯源树,研究论文发展脉络
Chat Paper
正在生成论文摘要