Differentiable Clustering for Graph Attention
IEEE Transactions on Knowledge and Data Engineering(2024)
摘要
Graph clusters (or communities) represent important graph structural information. In this paper, we present
D
ifferentiable
C
lustering for graph
AT
tention (DCAT). To the best of our knowledge, DCAT is the first solution that incorporates graph clustering into graph attention networks (GAT) to learn cluster-aware attention scores for semi-supervised learning tasks. In DCAT, we propose a novel approach to formunderlineating graph clustering as an auxiliary differentiable objective based on modunderlinearity maximization, which can be optimized together with the learning objective of GAT for a semi-supervised task. Specifically, we propose a solution to relaxing modunderlinearity maximization from a discrete optimization problem to a differentiable objective with theoretical guarantee so that we can learn cluster-aware attention scores by jointly learning from graph clustering and a semi-supervised learning task. To address the computational challenge, we further propose to reformunderlineate the constraint introduced by the clustering objective into a new form. Our analysis shows that DCAT allocates higher attention scores to nodes within the same cluster, allowing them to have a higher influence in node representation learning, and thus DCAT will generate better node representations for downstream applications. The experimental resunderlinets on commonly used datasets show that DCAT outperforms popunderlinear and state-of-the-art graph neural networks.
更多查看译文
关键词
Graph neural networks,graph attention networks,attention mechanism,semi-supervised learning,graph clustering
AI 理解论文
溯源树
样例
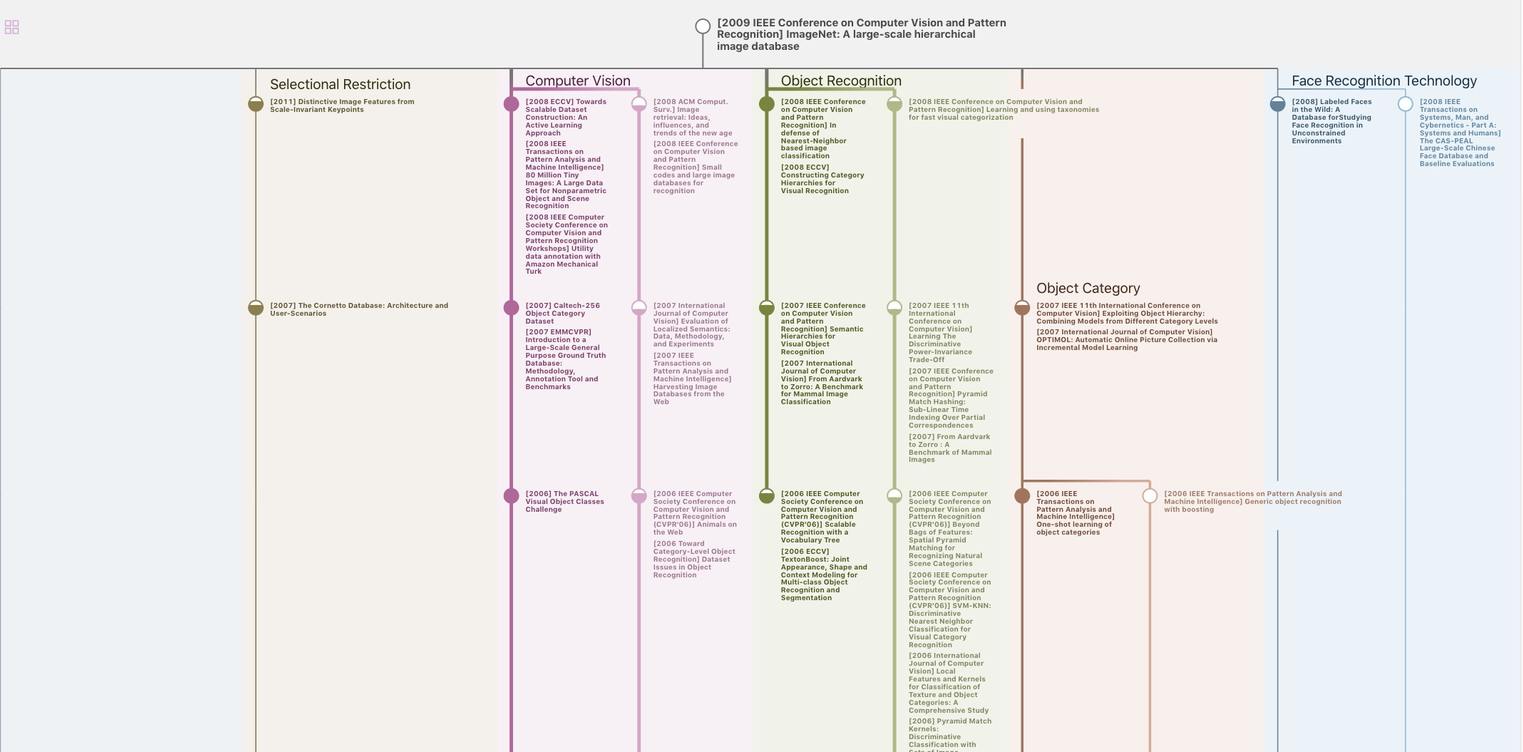
生成溯源树,研究论文发展脉络
Chat Paper
正在生成论文摘要