FedCORE: Federated Learning for Cross-Organization Recommendation Ecosystem
IEEE Transactions on Knowledge and Data Engineering(2024)
摘要
A recommendation system is of vital importance in delivering personalization services, which often brings continuous dual improvement in user experience and organization revenue. However, the data of one single organization may not be enough to build an accurate recommendation model for inactive or new cold-start users. Moreover, due to the recent regulatory restrictions on user privacy and data security, as well as the commercial conflicts, the raw data in different organizations cannot be merged to alleviate the scarcity issue in training a model. In order to learn users’ preferences from such cross-silo data of different organizations and then provide recommendations to the cold-start users, we propose a novel federated learning framework, i.e., federated cross-organization recommendation ecosystem (FedCORE). Specifically, we first focus on the ecosystem problem of cross-organization federated recommendation, including cooperation patterns and privacy protection. For the former, we propose a privacy-aware collaborative training and inference algorithm. For the latter, we define four levels of privacy leakage and propose some methods for protecting the privacy. We then conduct extensive experiments on three real-world datasets and two seminal recommendation models to study the impact of cooperation in our proposed ecosystem and the effectiveness of privacy protection.
更多查看译文
关键词
Federated Learning,Cross-Organization Recommendation
AI 理解论文
溯源树
样例
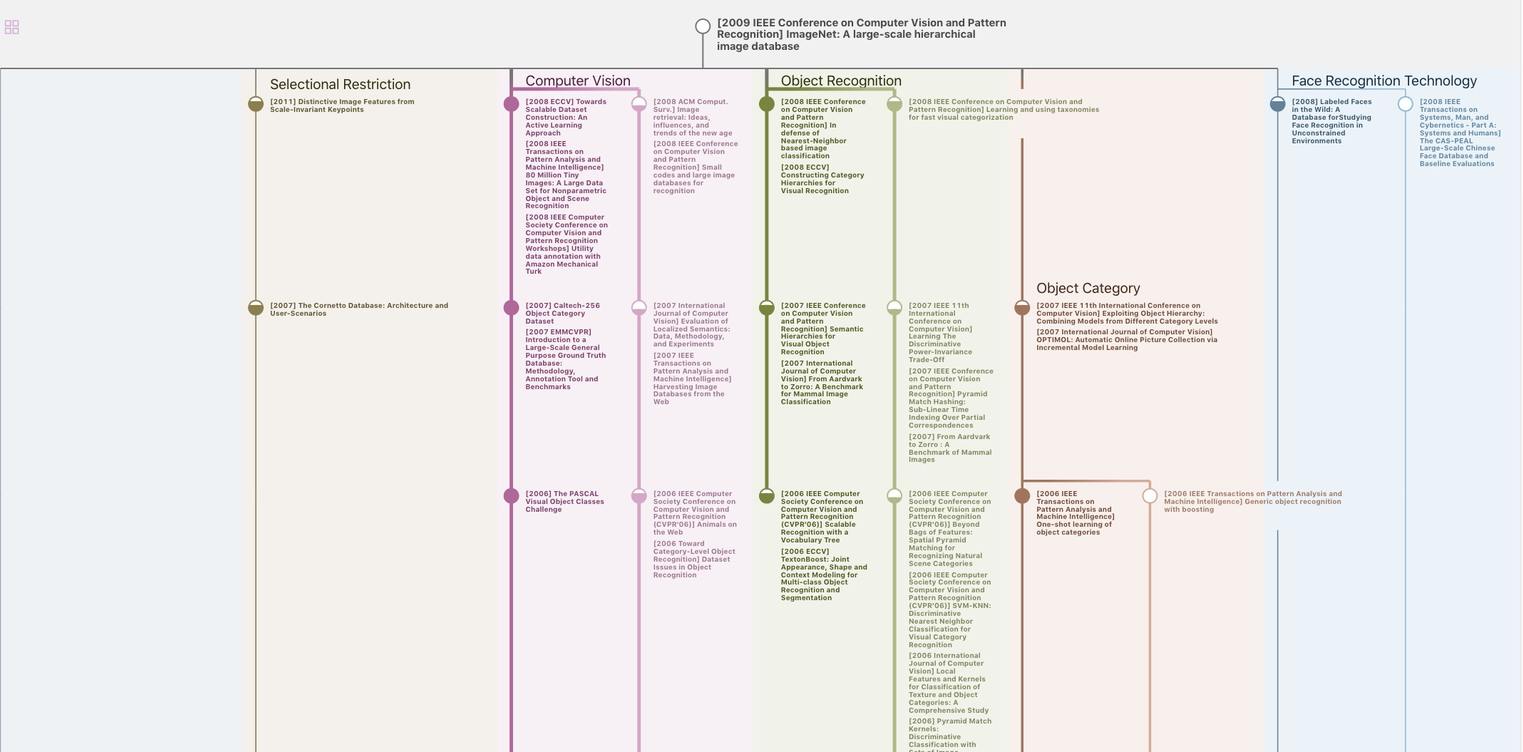
生成溯源树,研究论文发展脉络
Chat Paper
正在生成论文摘要