DECOR: Dynamic Decoupling and Multi-Objective Optimization for Long-tailed Remote Sensing Image Classification
IEEE Transactions on Geoscience and Remote Sensing(2024)
摘要
In the realm of remote sensing, targets of interest span a range of categories. However, their distribution is not always uniform. Certain categories substantially outnumber others, resulting in what’s termed a ‘long-tailed distribution’ in remote sensing imagery. This imbalanced distribution often biases a classifier’s focus toward the more abundant (head) classes, at the detriment of the less-represented (tail) classes. Such biases undermine the classifier’s generalization performance, particularly in the context of remote sensing image classification (RSIC). While existing mitigation approaches such as resampling, reweighting, and transfer learning offer some respite, they often miss out on in-depth knowledge refinement, rendering them less effective for severe long-tailed RSIC scenarios. To counter these challenges, we introduce DECOR, a dynamic decoupling and multi-objective optimization framework. Within DECOR, the feature extractor and classifier are dynamically decoupled, promoting superior feature representation and classifier training. Then, a multi-objective optimization approach is proposed to delve deeper, refining feature representation at the knowledge level using learnable feature centroids coupled with masked world knowledge learning. Moreover, to combat the pronounced effects of sample imbalance on classifier training, we employ a class-balanced re-sampling technique paired with a parameter-efficient adapter, which sharpens the classifier’s decision boundary and bridges the gap between representation and classification. DECOR’s efficacy is validated through comprehensive experiments on several datasets, including the NWPU-RESISC45-LT (NWPU-LT), AID-LT, and our self-built BIT-AFGR50-LT. Experimental results demonstrate DECOR’s marked enhancement in performance on long-tailed datasets. Our source code is available at: https://github.com/ChloeeGrace/DECOR.
更多查看译文
关键词
Long tail,remote sensing scene classification,decouple learning
AI 理解论文
溯源树
样例
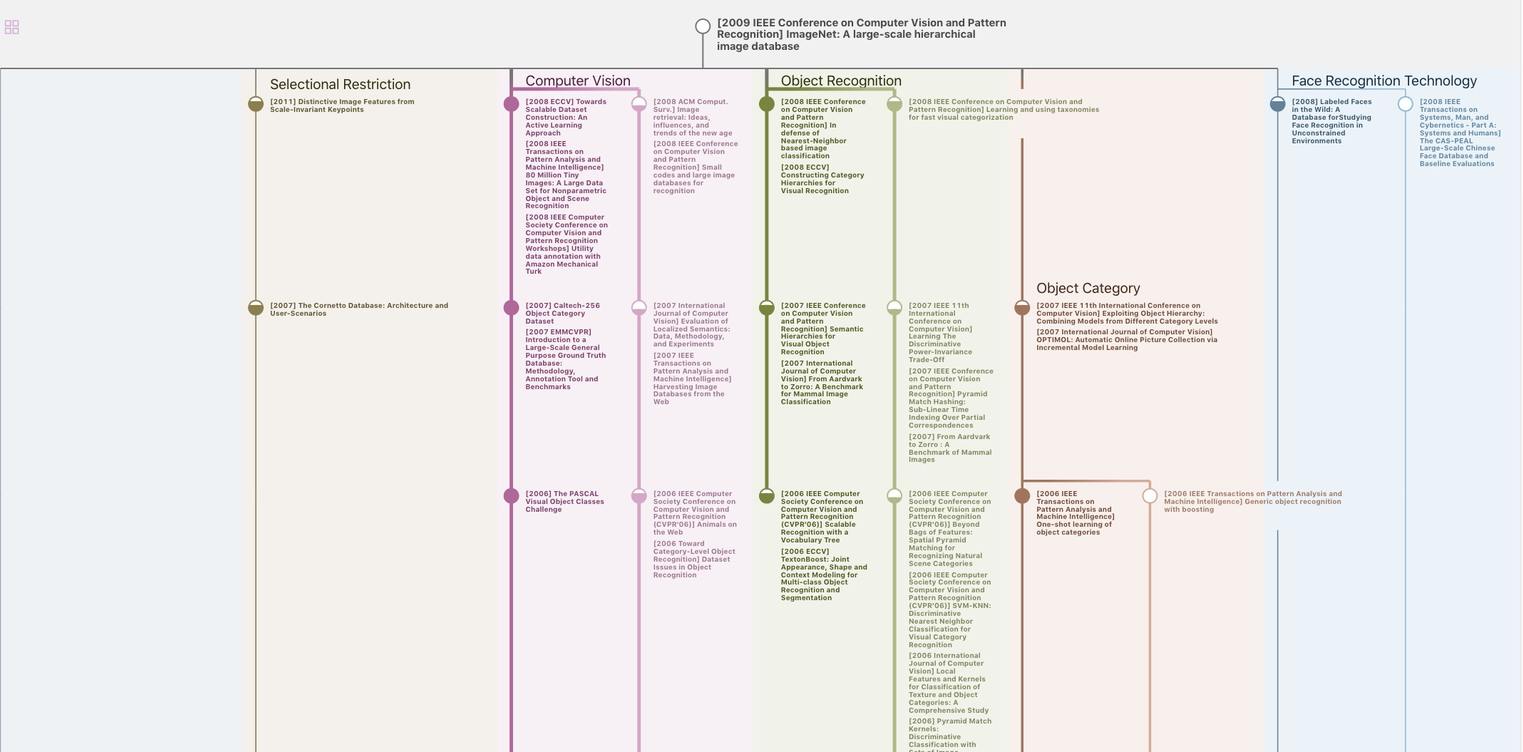
生成溯源树,研究论文发展脉络
Chat Paper
正在生成论文摘要