Gauss–Newton with Preconditioned Conjugate Gradient Magnetotelluric Inversion for 3D Axial Anisotropic Conductivities
IEEE Transactions on Geoscience and Remote Sensing(2024)
摘要
We present a regularized inversion method for three-dimensional (3D) magnetotelluric (MT) data with axial anisotropic conductivities based on the edge-based finite element (FE) method. The Gauss–Newton (GN) approach is used to minimize the inversion objective function, including data misfit and regularization penalties, considering both structural complexity and anisotropic penalties. The most time-intensive task in the 3D MT inversion process is solving the large sparse system of linear equations. To speed up the inversion calculation, a hybrid direct–iterative solver combined with a block-diagonal preconditioner that has not yet been applied in anisotropic inversion is developed to accelerate the solutions for the sparse linear system resulting from forward modeling and sensitivity computations. In each GN iteration, a preconditioned conjugate gradient (PCG) method is adopted to overcome the difficulty of the sensitivity matrix storage for the anisotropic scene and obtain a model update without explicitly calculating and storing the sensitivity matrix. Before the inversion test, we use a model to demonstrate that the hybrid solver is computationally beneficial in terms of memory usage and time spent as compared to the direct solver. The good convergence properties and efficiency of the GN–PCG inversion scheme are demonstrated by two synthetic models and USArray data. The proposed inversion scheme can be an important supplement to existing anisotropic inversion algorithms and provide technical support for MT data interpretation.
更多查看译文
关键词
Magnetotelluric,axial anisotropic,Gauss-Newton inversion
AI 理解论文
溯源树
样例
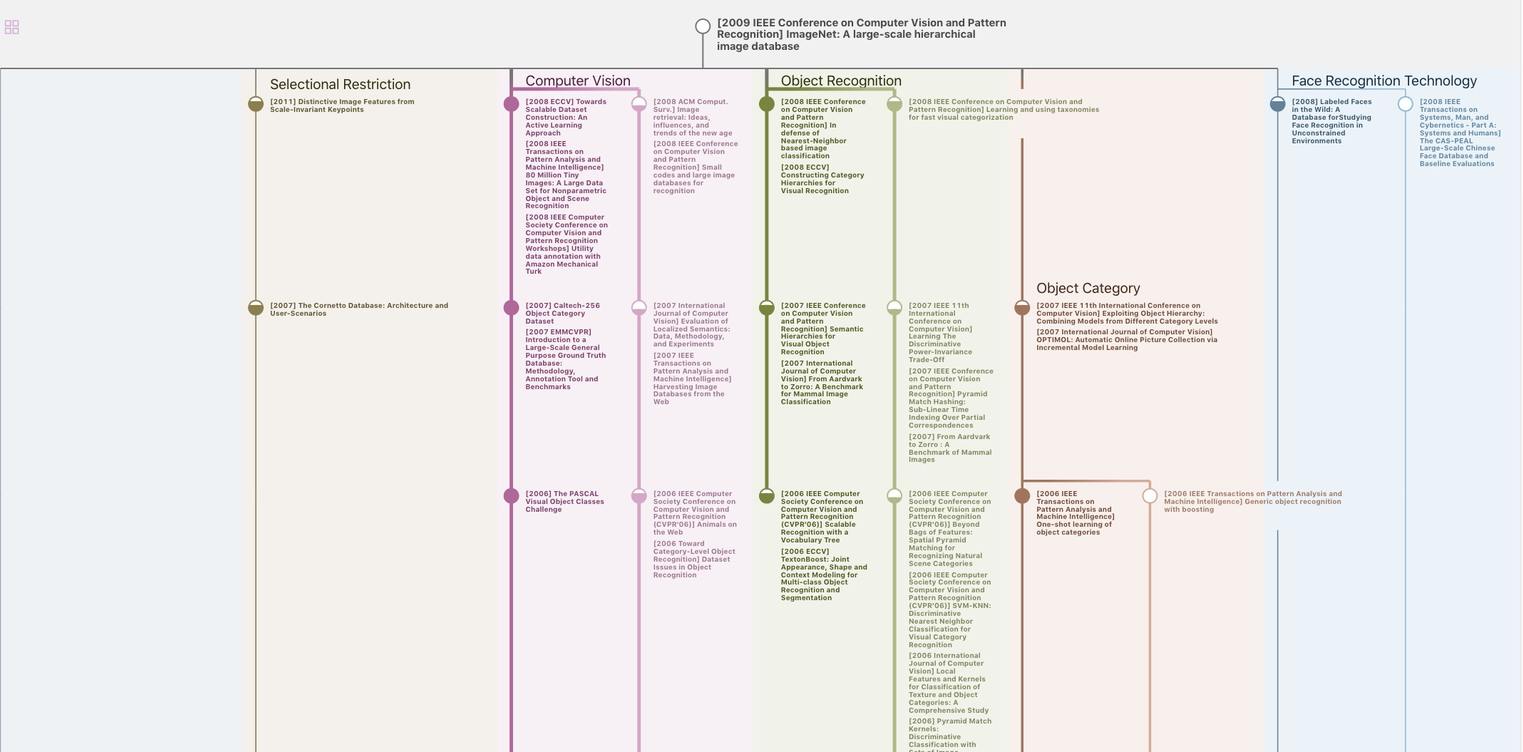
生成溯源树,研究论文发展脉络
Chat Paper
正在生成论文摘要