Choquet-like Integrals with Rough Attribute Fuzzy Measures for Data-driven Decision-making
IEEE Transactions on Fuzzy Systems(2024)
摘要
As nonlinear fuzzy aggregation functions, Choquetlike integrals with fuzzy measures are widely used in decisionmaking, rule-based classification and information fusion. However, the fuzzy measures in the existing Choquet-like integrals are typically provided via human intervention, not driven by data, thereby significantly limiting the automation level of the resulting systems. As an effective data-driven tool, rough set theory has shown its great potential for attribute reduction while dealing with many real-world problems. Nonetheless, different reduction methods generally lead to different outcomes, whilst obtaining all reductions exhaustively is NP-hard. Therefore, it is an interesting challenge to induce fuzzy measures by rough sets, using corresponding Choquet-like integrals to establish a datadriven decision-making method which is applicable for practical problems. To tackle this challenge, Choquet-like integrals based on rough attribute fuzzy measures are introduced here. Also, a novel decision-making model exploiting the resulting Choquetlike integrals, for problems of fault diagnosis and classification. First, a form of data-driven fuzzy measure is introduced through the specificity measures of rough sets, which is named as rough attribute fuzzy measure. Second, for decision information systems, the concept of p-matching degree between two objects is defined over different domain attributes. Third, based on rough attribute fuzzy measures and p-matching degrees, a type of Choquet-like integral is established. Subsequently the new decision-making network model and its associated computational algorithm are provided. The proposed approach is evaluated over both numerical examples and public datasets to demonstrate its efficacy.
更多查看译文
关键词
Rough set,Fuzzy measure,Choquet-like integral,Decision-making,Classification
AI 理解论文
溯源树
样例
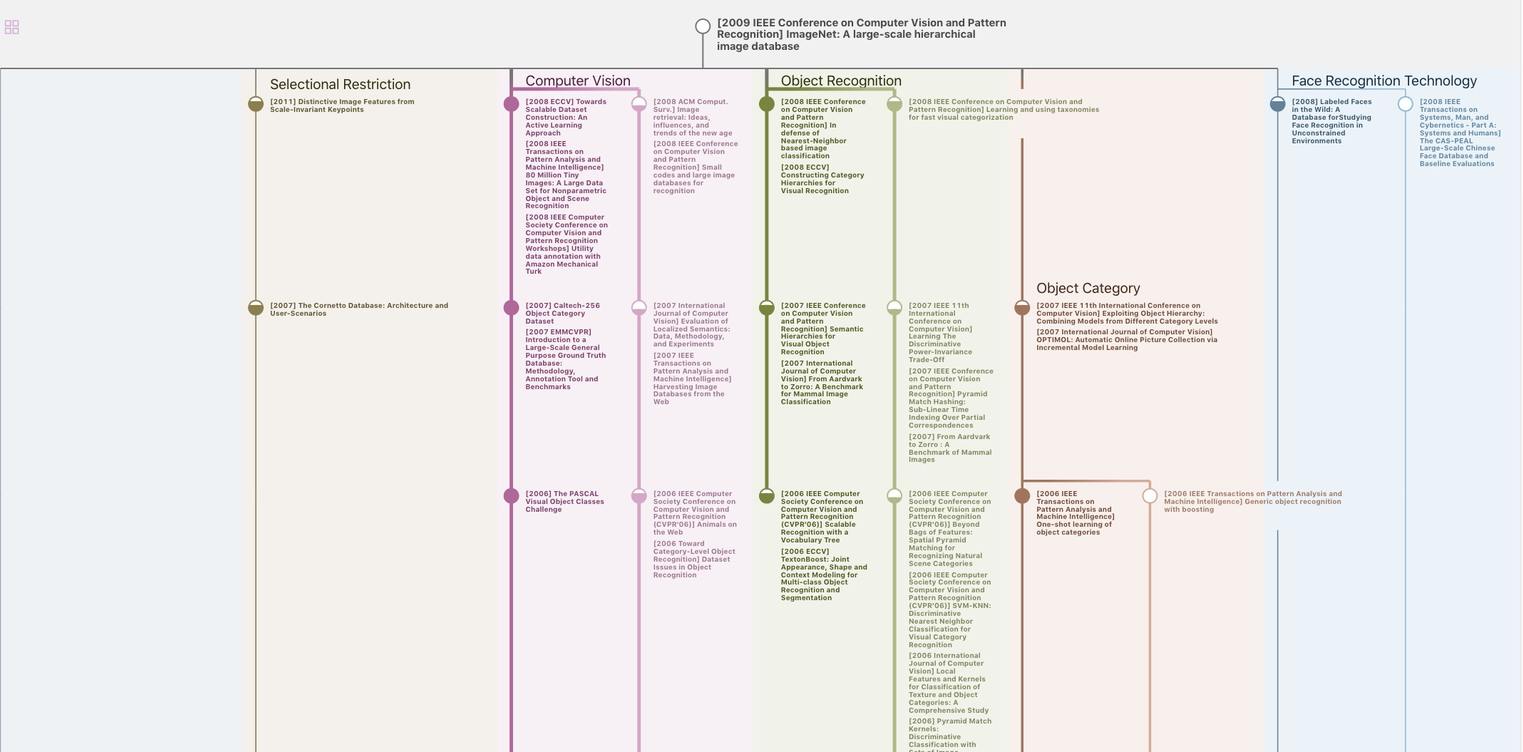
生成溯源树,研究论文发展脉络
Chat Paper
正在生成论文摘要