Improving IoT Security With Explainable AI: Quantitative Evaluation of Explainability for IoT Botnet Detection
IEEE Internet of Things Journal(2024)
摘要
Detecting botnets is an essential task to ensure the security of IoT systems. Machine learning-based approaches have been widely used for this purpose, but the lack of interpretability and transparency of the models often limits their effectiveness. In this research paper, our aim is to improve the transparency and interpretability of high-performance machine learning models for IoT botnet detection by selecting higher-quality explanations using explainable artificial intelligence (XAI) techniques. We used three datasets to induce binary and multiclass classification models for IoT botnet detection, with Sequential Backward Selection employed as the feature selection technique. We then use two post hoc XAI techniques such as LIME and SHAP, to explain the behaviour of the models. To evaluate the quality of explanations generated by XAI methods, we employed faithfulness, monotonicity, complexity, and sensitivity metrics. ML models employed in this work achieve very high detection rates with a limited number of features. Our findings demonstrate the effectiveness of XAI methods in improving the interpretability and transparency of machine learning-based IoT botnet detection models. Specifically, explanations generated by applying LIME and SHAP to the XGBoost model yield high faithfulness, high Consistency, low complexity, and low sensitivity. Furthermore, SHAP outperforms LIME by achieving better results in these metrics.
更多查看译文
关键词
XAI,Feature Importance,LIME,SHAP,Faithfulness,Complexity,Robustness,Consistency,IoT,Botnet,Posthoc XAI
AI 理解论文
溯源树
样例
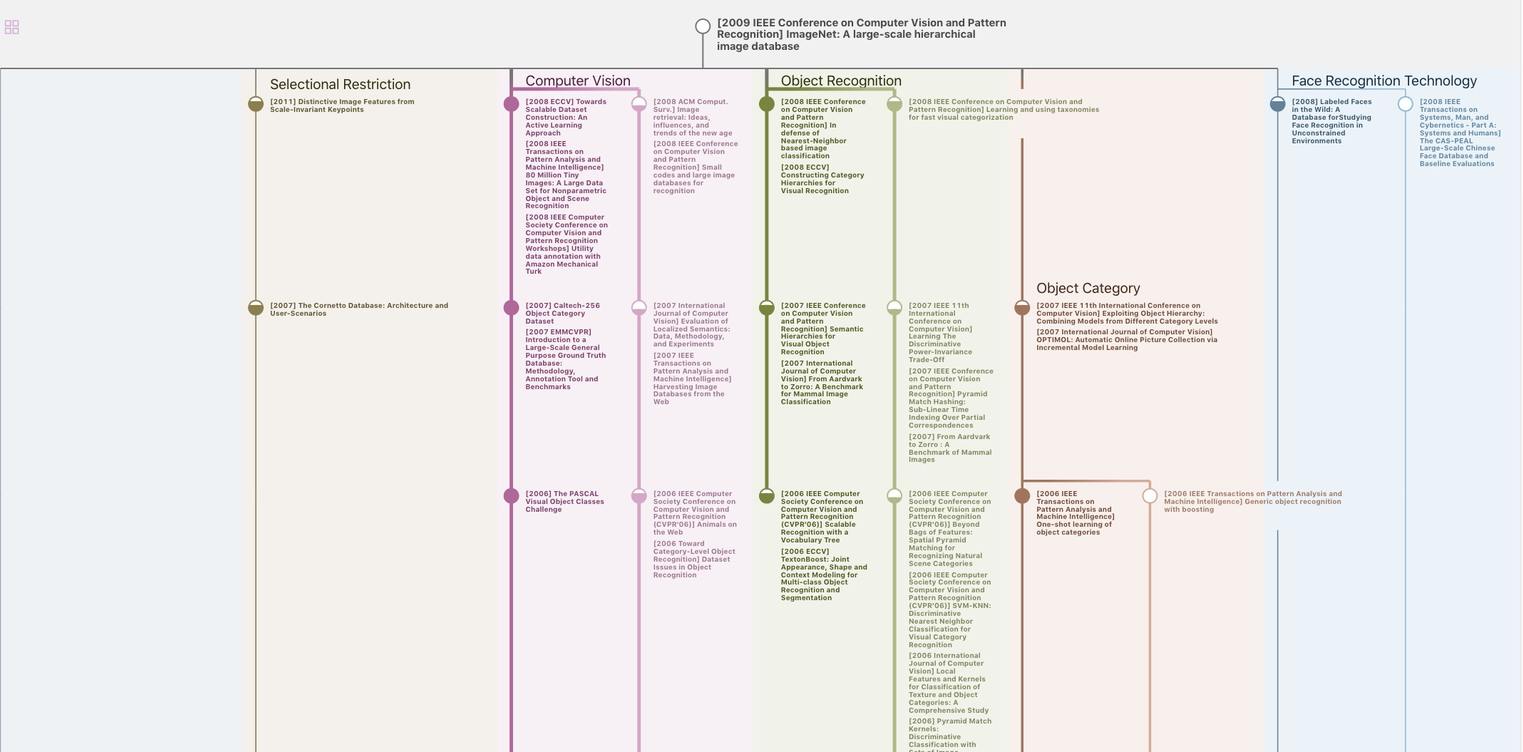
生成溯源树,研究论文发展脉络
Chat Paper
正在生成论文摘要