Localization in Underwater Acoustic IoT Networks: Dealing With Perturbed Anchors and Stratification
IEEE Internet of Things Journal(2024)
摘要
Underwater acoustic Internet of Things Networks (UAIoTNs) play a crucial role in oceanographic and environmental monitoring, necessitating precise localization for optimal functionality. However, the underwater setting introduces significant challenges, encompassing the stratification effect arising from underwater heterogeneity, uncertainty in anchor positions due to currents, and variations in the signal transmission environment. These factors collectively impede the accurate estimation of location. Consequently, this paper addresses these challenges by analyzing and deriving a closed-form solution using a time-of-arrival (TOA)-based technique for 3D localization in UAIoTNs. The investigation establishes an underwater stratified propagation model, drawing inspiration from ray tracing theory and Snell’s law. Employing the Cramér-Rao lower bound (CRLB) framework, we explore scenarios both with and without considering perturbed anchors, utilizing the Banachiewicz-Schur theorem. To quantify the impact of the stratification effect and perturbed anchors on CRLB and mean square error (MSE), we further analyze and derive an MSE expression, employing Taylor-series linearization. Building on our analysis of the detrimental effects of stratification and inaccurate anchors, we introduce a multiple-weighted least squares (MWLS) algorithm to alleviate potential performance losses. This approach integrates a matrix operator in the update step, eliminating variable dependencies and resulting in a closed-form solution that circumvents the need for iterative processes. Our simulation results validate our analytical findings and demonstrate the effectiveness of the proposed method, showcasing improved localization accuracy across various scenarios when compared to state-of-the-art approaches.
更多查看译文
关键词
Underwater acoustic Internet of Things Networks (UAIoTNs),localization,Cramér-Rao Lower Bound (CRLB),stratification effect,perturbed anchors
AI 理解论文
溯源树
样例
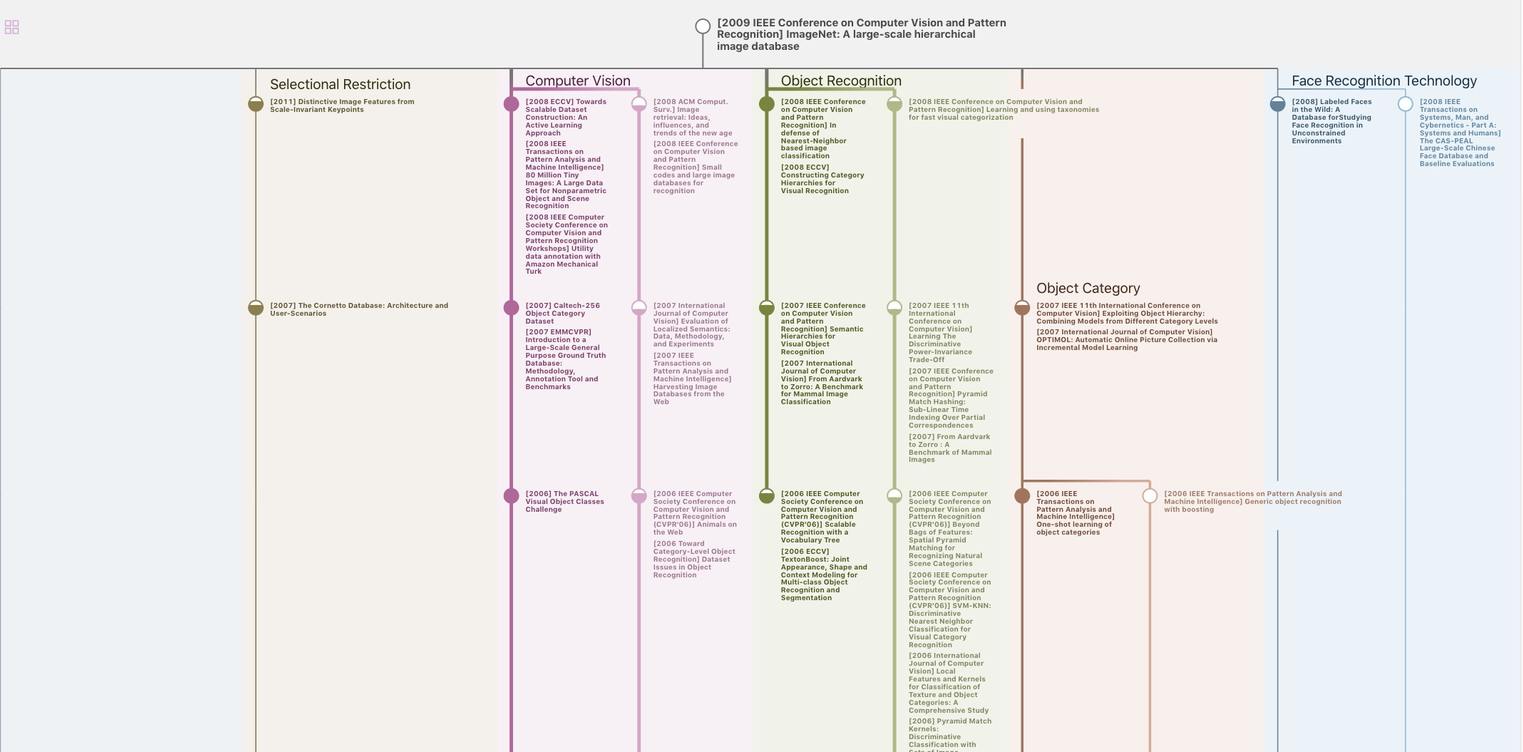
生成溯源树,研究论文发展脉络
Chat Paper
正在生成论文摘要