Shapley value-based approaches to explain the quality of predictions by classifiers
IEEE Transactions on Artificial Intelligence(2024)
摘要
The use of algorithm-agnostic approaches for explainable machine learning is an emerging area of research. When explaining the contribution of features towards the predicted outcome, traditionally, the focus remains on explaining the prediction itself, however a little has been done on explaining the quality of prediction of these models, where the quality can be assessed by the algorithm performance when changing the thresholds for classification. In this paper, we propose the use of Shapley values to explain the contribution of features towards the overall algorithm performance, measured in terms of Receiver-operating Characteristics (ROC) curve and the Area under the ROC curve (AUC). With the help of an illustrative example, we demonstrate the proposed idea of explaining the ROC curve, and visualising the uncertainties in these curves. For imbalanced datasets, the use of Precision-Recall Curve (PRC) is considered more appropriate, therefore we also demonstrate how to explain the PRCs with the help of Shapley values. The explanation of the model performance can help analysts in a number of ways, for example, in feature selection by identifying the irrelevant features that can be removed to reduce the computational complexity. It can also help in identifying the features having critical contributions towards the overall algorithm performance.
更多查看译文
关键词
explainable artificial intelligence,machine learning,business analytics
AI 理解论文
溯源树
样例
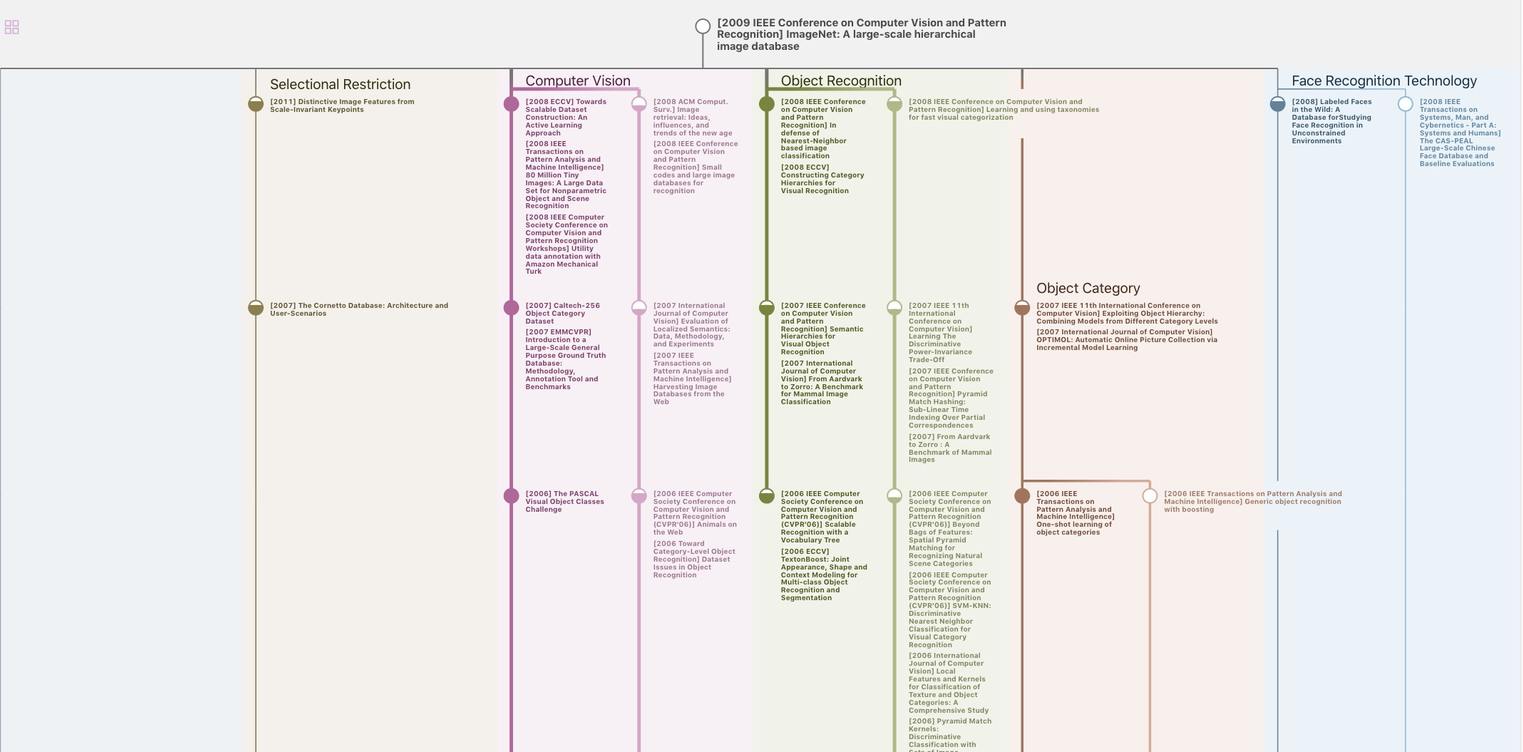
生成溯源树,研究论文发展脉络
Chat Paper
正在生成论文摘要