Follow My Instruction and Spill the Beans: Scalable Data Extraction from Retrieval-Augmented Generation Systems
CoRR(2024)
摘要
Retrieval-Augmented Generation (RAG) improves pre-trained models by
incorporating external knowledge at test time to enable customized adaptation.
We study the risk of datastore leakage in Retrieval-In-Context RAG Language
Models (LMs). We show that an adversary can exploit LMs' instruction-following
capabilities to easily extract text data verbatim from the datastore of RAG
systems built with instruction-tuned LMs via prompt injection. The
vulnerability exists for a wide range of modern LMs that span Llama2,
Mistral/Mixtral, Vicuna, SOLAR, WizardLM, Qwen1.5, and Platypus2, and the
exploitability exacerbates as the model size scales up. Extending our study to
production RAG models GPTs, we design an attack that can cause datastore
leakage with a 100
at most 2 queries, and we extract text data verbatim at a rate of 41
book of 77,000 words and 3
GPTs with only 100 queries generated by themselves.
更多查看译文
AI 理解论文
溯源树
样例
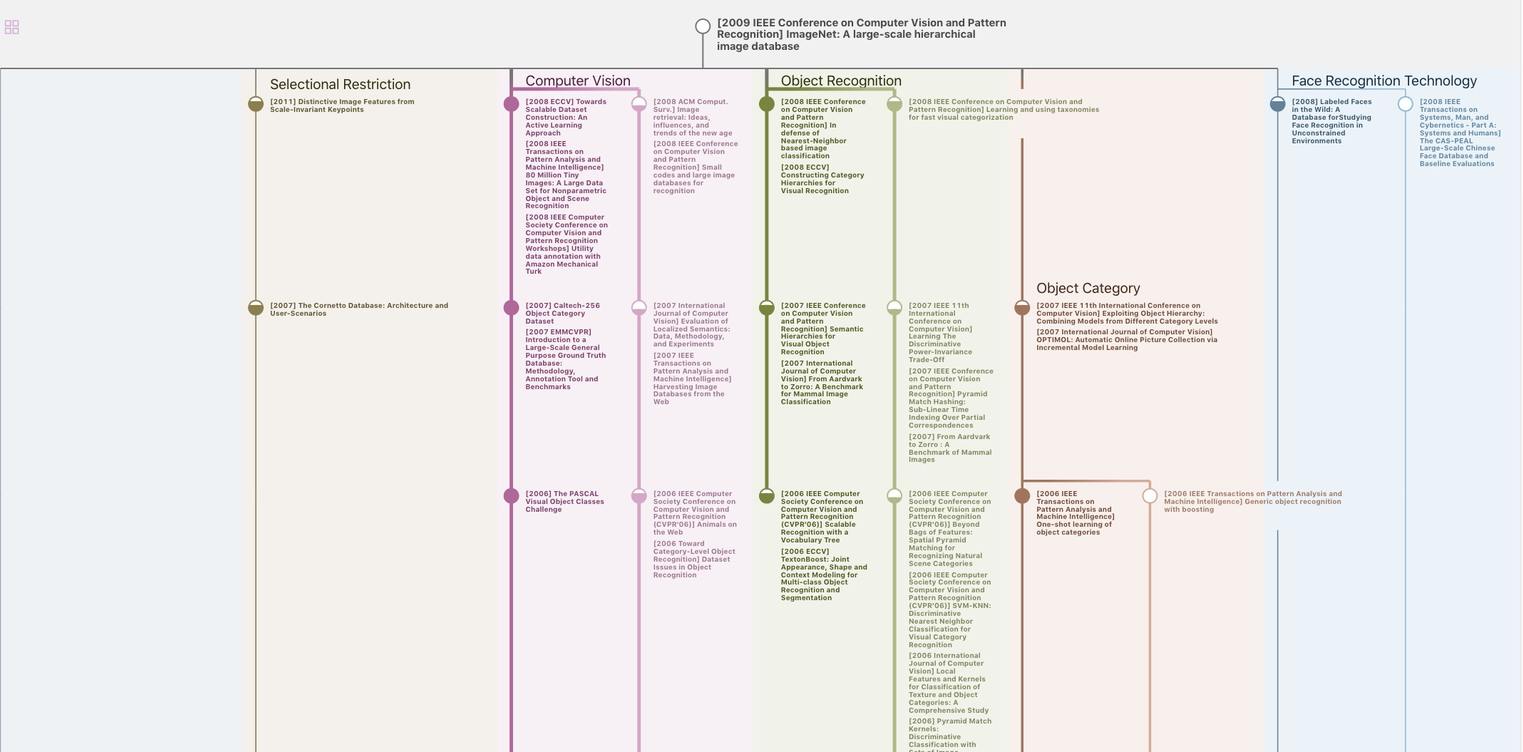
生成溯源树,研究论文发展脉络
Chat Paper
正在生成论文摘要