An Interpretable Framework for Predicting Type 2 Diabetes using ML and Explainable AI
2023 26th International Conference on Computer and Information Technology (ICCIT)(2023)
摘要
Diabetes Mellitus is an incurable disease and stands as a major universe cause of destruction. With the universal prevalence of diabetes increasing rapidly, accurate detection and identification of the disease have become crucial. In this paper, we present an explainable AI-based diabetes indicative methodology that is carefully constructed, effective, and, most importantly, interpretable. Using two real-world diabetes datasets, the three most well-known machine learning classifiers in the literature—Random Forest (RF), Decision Tree (DT), and XGBoost—were subjected with quantitative assessments. After training and assessment of all classification models, the suggested method achieved the best results in the XGBoost classifier for the Sylhet dataset with 99.4% accuracy and the Pima Indian dataset with 92.59% accuracy. The datasets were normalized across observations to provide standardized values for improved analytical applicability and comparability. Explainable AI has been implemented for the XGBoost machine learning model by generating both global and local explanations using Shapley additive explanations (SHAP) and Local interpretable model-agnostic explanations (LIME). The elements that contribute to diabetes are explained and shown in graphs to help medical professionals make decisions about clinical diagnosis and treatment options.
更多查看译文
关键词
Diabetes,explainable AI,diabetes diagnosis,interpretable,Random Forest (RF),Decision Tree (DT),XGBoost,Sylhet dataset,Pima Indian dataset,LIME,SHAP
AI 理解论文
溯源树
样例
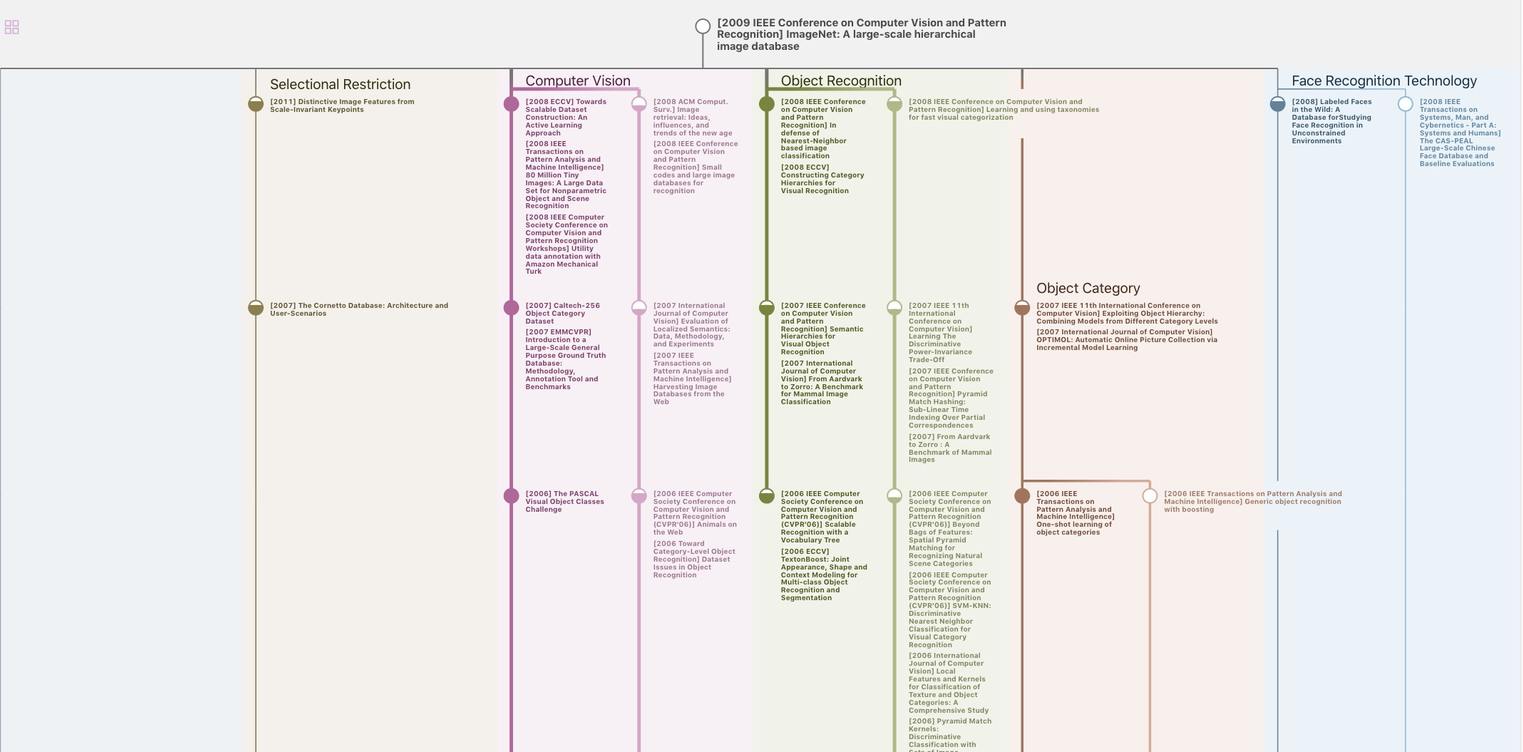
生成溯源树,研究论文发展脉络
Chat Paper
正在生成论文摘要