A Graph Convolutional Network Approach to Qualitative Classification of Hydrocarbon Zones Using Petrophysical Properties in Well Logs
Natural Resources Research(2024)
摘要
The discovery of hydrocarbon reserves has declined significantly in recent years owing to the structural and lithological heterogeneities present in reservoirs. To overcome this decline, it is crucial to incorporate advanced computational methods, such as machine learning (ML) and deep learning (DL). These technologies can facilitate more precise discovery of hydrocarbon reserves, thereby replenishing and increasing the supply of proven reserves. By utilizing ML and DL, likelihood of errors arising from human error or bias during exploration activities can be diminished substantially. This is due to the extensive incorporation of sophisticated statistical techniques within the applications of ML and DL. Well logs provide valuable information about the physical characteristics of subterranean fluids and rocks. In the McKee field in New Zealand, the lithology of three wells was determined using petrophysical parameters, such as porosity, permeability, water saturation, volume shale, and oil saturation that were extracted from the well logs. The unsupervised method K-means clustering (KMC) was used to perform facies classification tasks, utilizing clusters that matched the well’s facies and ranged from 5 to 10 and each well yielded six pairs of outputs. Graph convolutional networks (GCN) are reliable technique for working with graph representations. The best performance is achieved by directly integrating graph convolutions with feature information and related parameters. The petrophysical parameters were combined with the unlabeled KMC outputs to form the GCN dataset. The initial potential zones were classified into five classes based on petrophysical criteria: very high, high, moderate, low, and very low. This GCN approach was used to identify each graph quality in the dataset. The hydrocarbon potential of the three wells was evaluated using the graph dataset and the GCN approach, which produced results with higher accuracy when real labels were used. The findings of this study indicate that the identification of a hydrocarbon-rich region through the utilization of a graph that integrates lithological and petrophysical data requires a comprehensive understanding of the subsurface that goes beyond lithology alone. To achieve this, a novel method for predicting hydrocarbon potential based on GCN is proposed, which combines graph datasets derived from well logs consisting of petrophysical entities and depth values.
更多查看译文
关键词
Graph neural networks,deep learning,hydrocarbon quality estimation,depth classification,facies classification
AI 理解论文
溯源树
样例
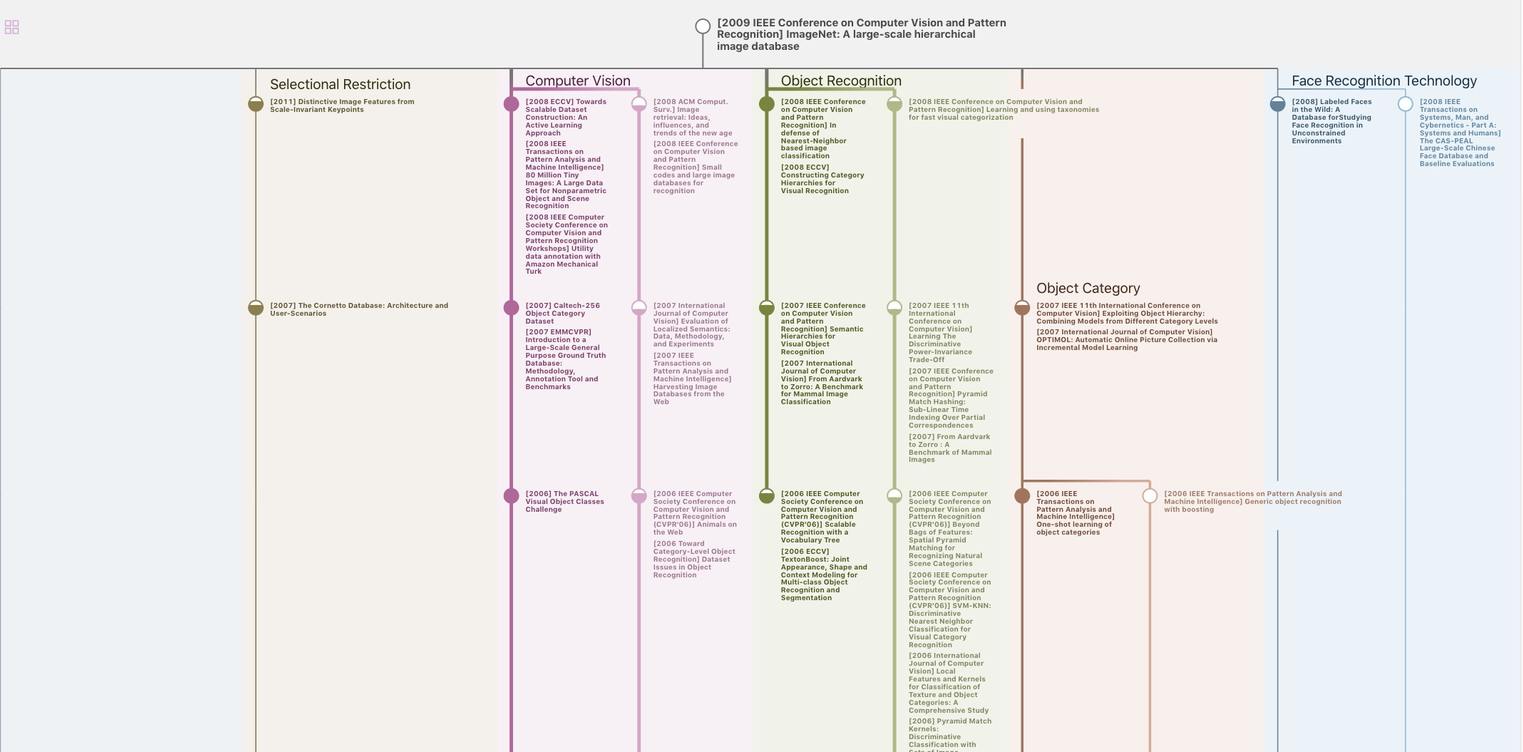
生成溯源树,研究论文发展脉络
Chat Paper
正在生成论文摘要