Federated Optimal Framework with Low-bitwidth Quantization for Distribution System.
Global Communications Conference(2023)
摘要
Federated learning is an attractive solution for efficient data processing and optimization in distributed networks. However, communication bottlenecks often result in sluggish optimization and increased resource consumption, leading to reduced system efficiency. To address these challenges, this paper proposes a novel approach based on Federated Low-bitwidth Quantization (FLQ) framework, which optimizes resource utilization in distributed communications. FLQ quantizes network parameters and gradients, significantly reducing computational and communication costs related to parameter broadcasting and gradient uploading. By utilizing an 8-bit low-bitwidth training and binary vector compression technique, our algorithm greatly enhances network convergence and is well-suited to the energy consumption characteristics of servers and end devices. Moreover, to enhance overall device coordination, we develop a dynamic resource allocation scheme that adapts to changing requirements of individual nodes. Results from extensive experiments demonstrate that our approach leads to faster convergence, lower computational and communication costs and optimized joint network deployment in Internet of Things scenarios.
更多查看译文
关键词
Federated learning,Resource optimization,Low-bitwidth quantization,Gradient compression,Internet of things
AI 理解论文
溯源树
样例
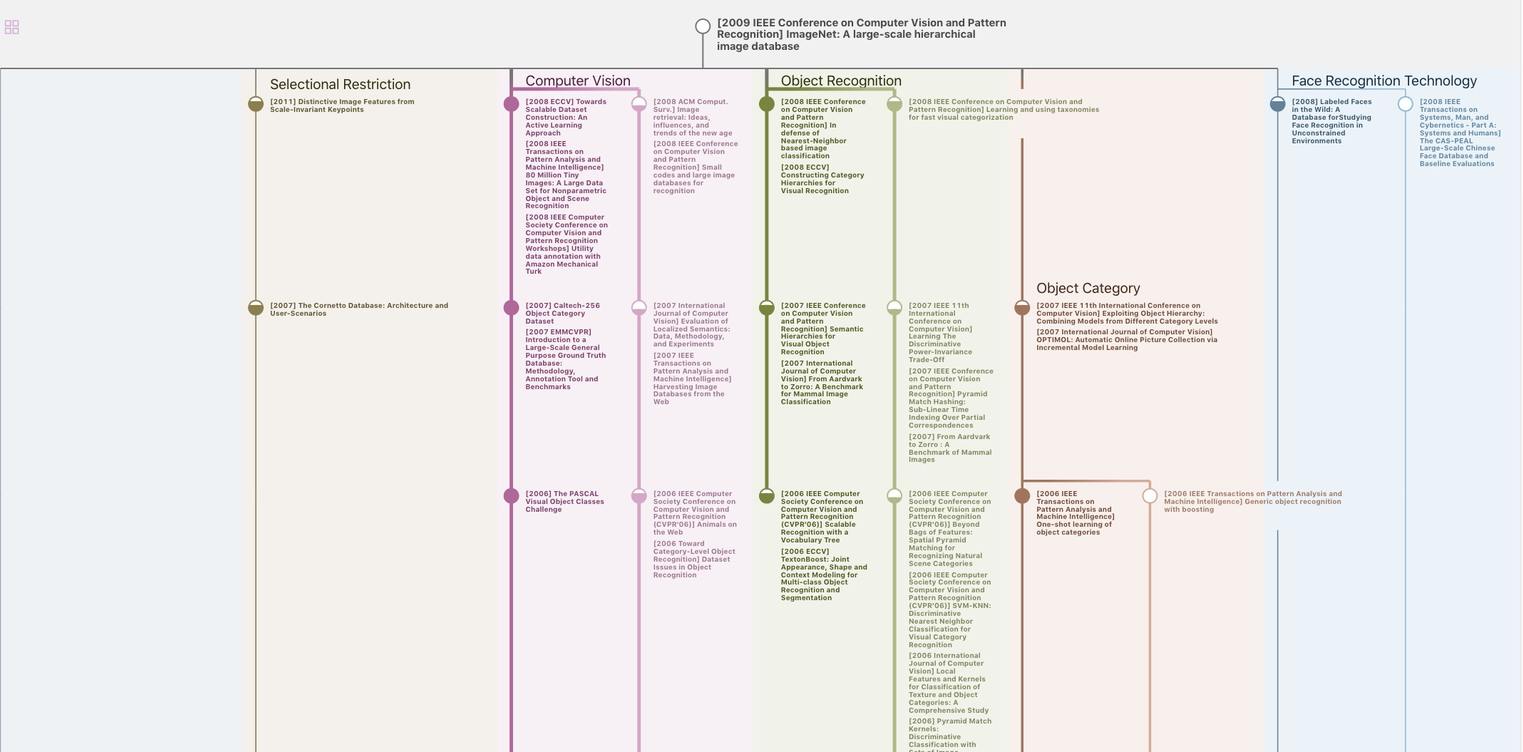
生成溯源树,研究论文发展脉络
Chat Paper
正在生成论文摘要