An Adversarial Robustness Benchmark for Enterprise Network Intrusion Detection
CoRR(2024)
摘要
As cyber-attacks become more sophisticated, improving the robustness of
Machine Learning (ML) models must be a priority for enterprises of all sizes.
To reliably compare the robustness of different ML models for cyber-attack
detection in enterprise computer networks, they must be evaluated in
standardized conditions. This work presents a methodical adversarial robustness
benchmark of multiple decision tree ensembles with constrained adversarial
examples generated from standard datasets. The robustness of regularly and
adversarially trained RF, XGB, LGBM, and EBM models was evaluated on the
original CICIDS2017 dataset, a corrected version of it designated as NewCICIDS,
and the HIKARI dataset, which contains more recent network traffic. NewCICIDS
led to models with a better performance, especially XGB and EBM, but RF and
LGBM were less robust against the more recent cyber-attacks of HIKARI. Overall,
the robustness of the models to adversarial cyber-attack examples was improved
without their generalization to regular traffic being affected, enabling a
reliable detection of suspicious activity without costly increases of false
alarms.
更多查看译文
AI 理解论文
溯源树
样例
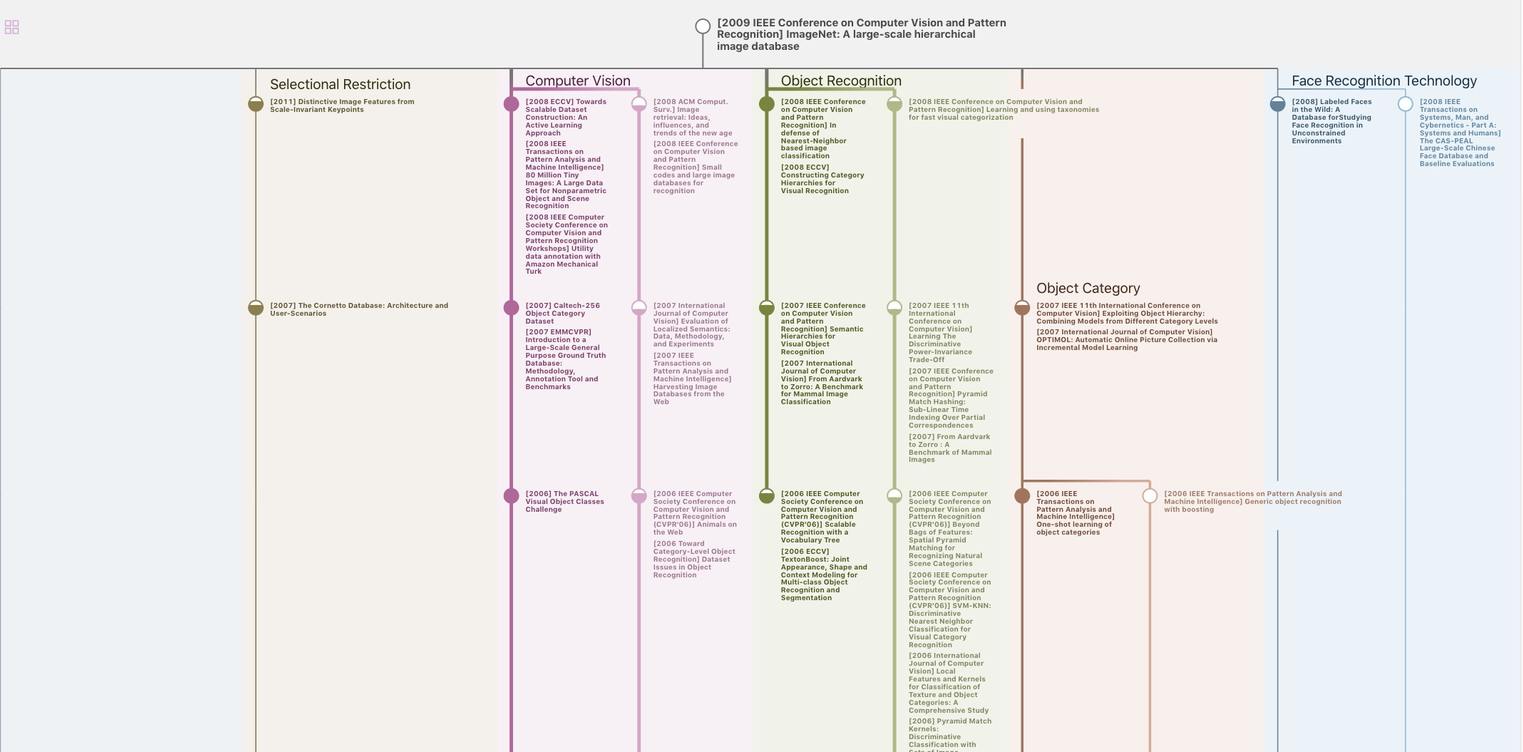
生成溯源树,研究论文发展脉络
Chat Paper
正在生成论文摘要