Multi-Agent Reinforcement Learning for Wireless Networks Against Adversarial Communications.
Global Communications Conference(2023)
摘要
Based on the efficient and reliable exchange of learning messages containing both the policy selection experiences such as the learning parameters and observations among the learning agents, multi -agent reinforcement learning (RL) has to address adversarial communications that send fake learning messages to learning agents with the goal of decreasing the RL rewards or even failing the learning tasks. In this paper, we propose a multi-agent RL (MARL) communication framework for wireless networks against adversarial communications, in which each learning agent chooses the cooperative agents to share learning messages based on the agent reputation that indicates the probability to send fake learning messages. By comparing with the learning history, each learning agent authenticates the received learning messages before integrating them in the RL task state formulation and the learning parameter update for robust task learning. The learning factor that increases with the correlation between the local and the shared observation is calculated to update the task Q-values and neural network weights based on the shared learning parameters. As a case study, the multi-agent deep Q-network within our proposed MARL communication framework is implemented in the UAV swarm video transmission system and the performance gain over the benchmark is provided in the simulation results based on 5-UAV swarm against an attacker that sends fake observations and neural network weights.
更多查看译文
关键词
Multi-agent reinforcement learning,communications,reputation,adversarial communications,wireless networks
AI 理解论文
溯源树
样例
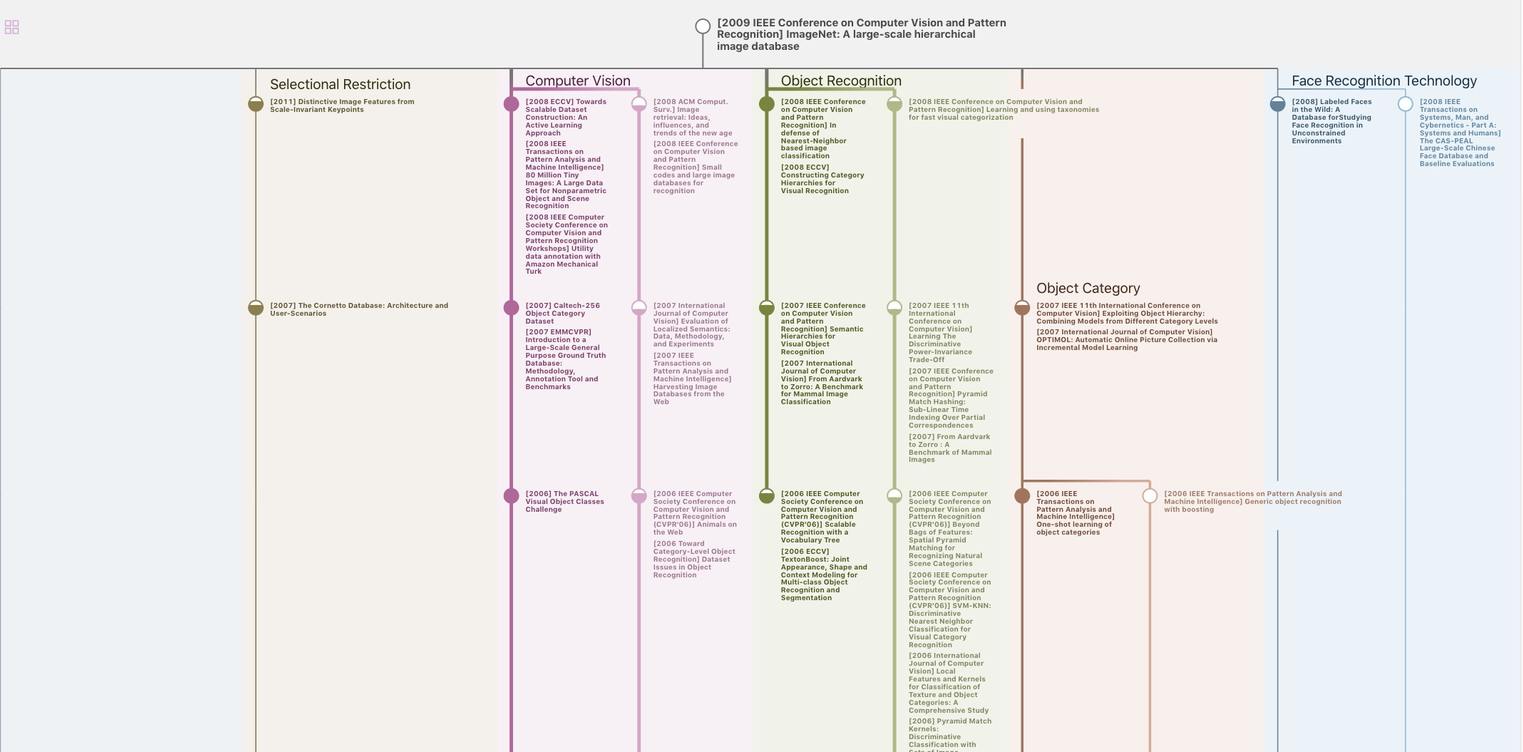
生成溯源树,研究论文发展脉络
Chat Paper
正在生成论文摘要