PCTDepth: Exploiting Parallel CNNs and Transformer via Dual Attention for Monocular Depth Estimation
Neural Processing Letters(2024)
Abstract
Monocular depth estimation (MDE) has made great progress with the development of convolutional neural networks (CNNs). However, these approaches suffer from essential shortsightedness due to the utilization of insufficient feature-based reasoning. To this end, we propose an effective parallel CNNs and Transformer model for MDE via dual attention (PCTDepth). Specifically, we use two stream backbones to extract features, where ResNet and Swin Transformer are utilized to obtain local detail features and global long-range dependencies, respectively. Furthermore, a hierarchical fusion module (HFM) is designed to actively exchange beneficial information for the complementation of each representation during the intermediate fusion. Finally, a dual attention module is incorporated for each fused feature in the decoder stage to improve the accuracy of the model by enhancing inter-channel correlations and focusing on relevant spatial locations. Comprehensive experiments on the KITTI dataset demonstrate that the proposed model consistently outperforms the other state-of-the-art methods.
MoreTranslated text
Key words
Monocular depth estimation,Hierarchical interaction fusion,Transformer,CNNs,Attention
AI Read Science
Must-Reading Tree
Example
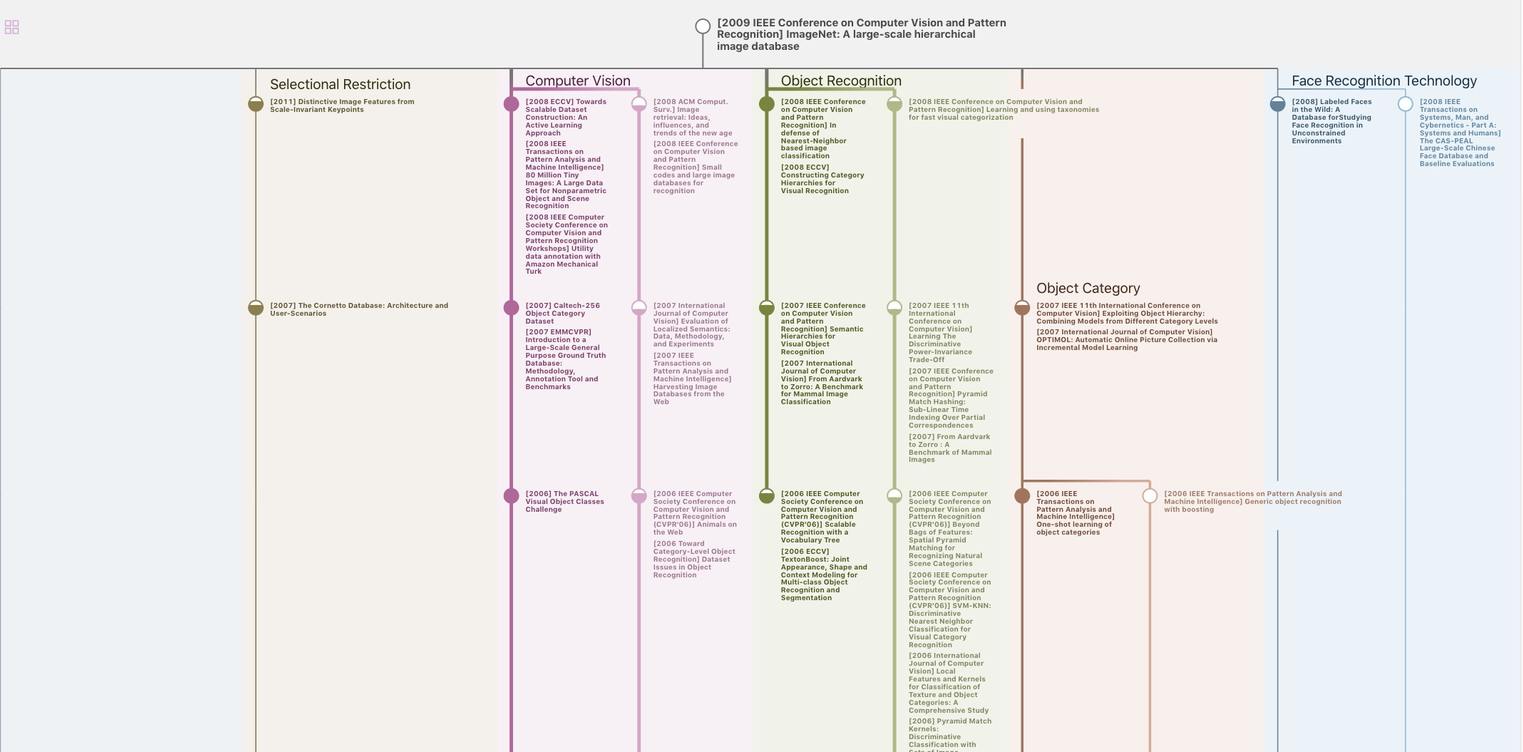
Generate MRT to find the research sequence of this paper
Chat Paper
Summary is being generated by the instructions you defined