Enhancing explainability in predicting mental health disorders using human–machine interaction
Multimedia Tools and Applications(2024)
摘要
Mental health measures an individual's emotional, psychological, and social well-being. It influences how a person thinks, feels, and responds to events. Mental illness has wreaked havoc on society in today's globe and has come to the forefront as a serious concern. People with mental disorders, including bipolar disorder, schizoaffective disorder, sadness, anxiety, and others, rarely recognize their condition as the world's most serious problem. In mental illness, there are a variety of emotional and physical symptoms. Anxiety attacks, sweating, palpitations, grief, worry, overthinking, delusions, and illusions are all symptoms of mental illness, and each symptom indicates the kind of mental disorder. Our study outlined the standardized approach for diagnostic depression, including data extraction, pre-processing, ML classifier training, identification classification, and performance assessment that enhances human–machine interaction. This study utilized five machine learning methods: k-nearest neighbor, linear regression, gaussian classifier, random forest, decision tree, and logistic regression. The accuracy, precision, recall, and F1-score metrics are used to evaluate the efficacy of machine learning models. The algorithms are categorised according to their accuracy, and explainability shows that the Gaussian classifier (Minmax scaler), which reaches 91 per cent accuracy, is the most accurate. Furthermore, given that the characteristics are predicated on potential indications of depression, the approach is capable of producing substantial justifications for the determination via machine learning models employing the SHapley Additive Explanations (SHAP) and Local Interpretable Model-Agnostic Explanations (LIME) algorithms of explainable Artificial Intelligence (XAI). Thus, the approach to predicting depression can aid in the advancement of intelligent chatbots and other technologies that improve mental health treatment.
更多查看译文
关键词
Mental health,Machine learning,Predictive models,Gaussian classifier,SHAP,LIME
AI 理解论文
溯源树
样例
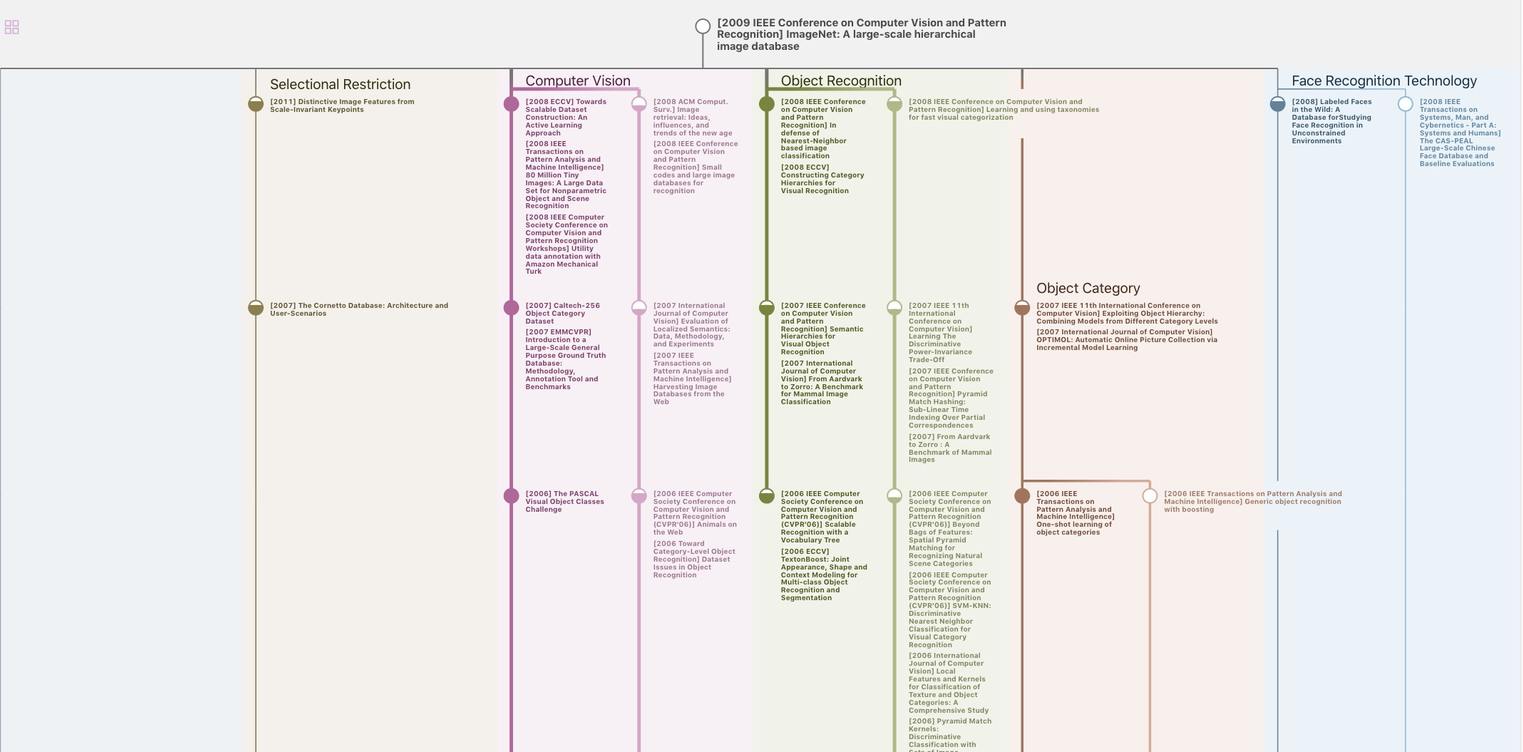
生成溯源树,研究论文发展脉络
Chat Paper
正在生成论文摘要