A general variation-driven network for medical image synthesis
Applied Intelligence(2024)
摘要
The significance of medical image synthesis has exponentially grown due to constrained medical resources, making it a critical component in numerous clinical applications. This process facilitates the generation of high-quality, multi-modal medical images, ultimately enhancing medical image diagnostics. Currently, prevailing medical image synthesis methodologies primarily rely on voxel-based or GAN-based strategies to address substantial challenges arising from disparities in various imaging principles and significant noise. However, these methodologies rarely consider the intensity distribution difference among multi-modal or multi-parameters medical images, which generates unstable and unexplainable results. In response to these limitations, we propose a novel approach-a general variation-driven neural network for medical image synthesis that considers explicit data distribution. Within this method, we introduce the concept of a variation-based distance metric, providing a quantitative framework for capturing distribution disparities between medical images originating from both the source and target domains. Subsequently, guided by this variation-based distance metric, we introduce a robust end-to-end neural network architecture carefully designed to synthesize target medical images. Our proposed method has undergone extensive experimentation across various medical image synthesis tasks, including cross-modality transformations between CT and MRI, high-dose CT synthesis from low-dose CT, and the conversion of multi-parameters in MRI, including T1, T2, T1ce, and Flair sequences. In comparative assessments against existing methods, our approach consistently outperforms them across three publicly available datasets: Gold Atlas, LDCT, and BraTS2018. Additionally, we have successfully applied our model to generate high-quality Micro-CT images in dental clinics from CBCT data, significantly enhancing diagnostic capabilities in this clinical setting.
更多查看译文
关键词
Medical image synthesis,Variation-based distance,Deep learning
AI 理解论文
溯源树
样例
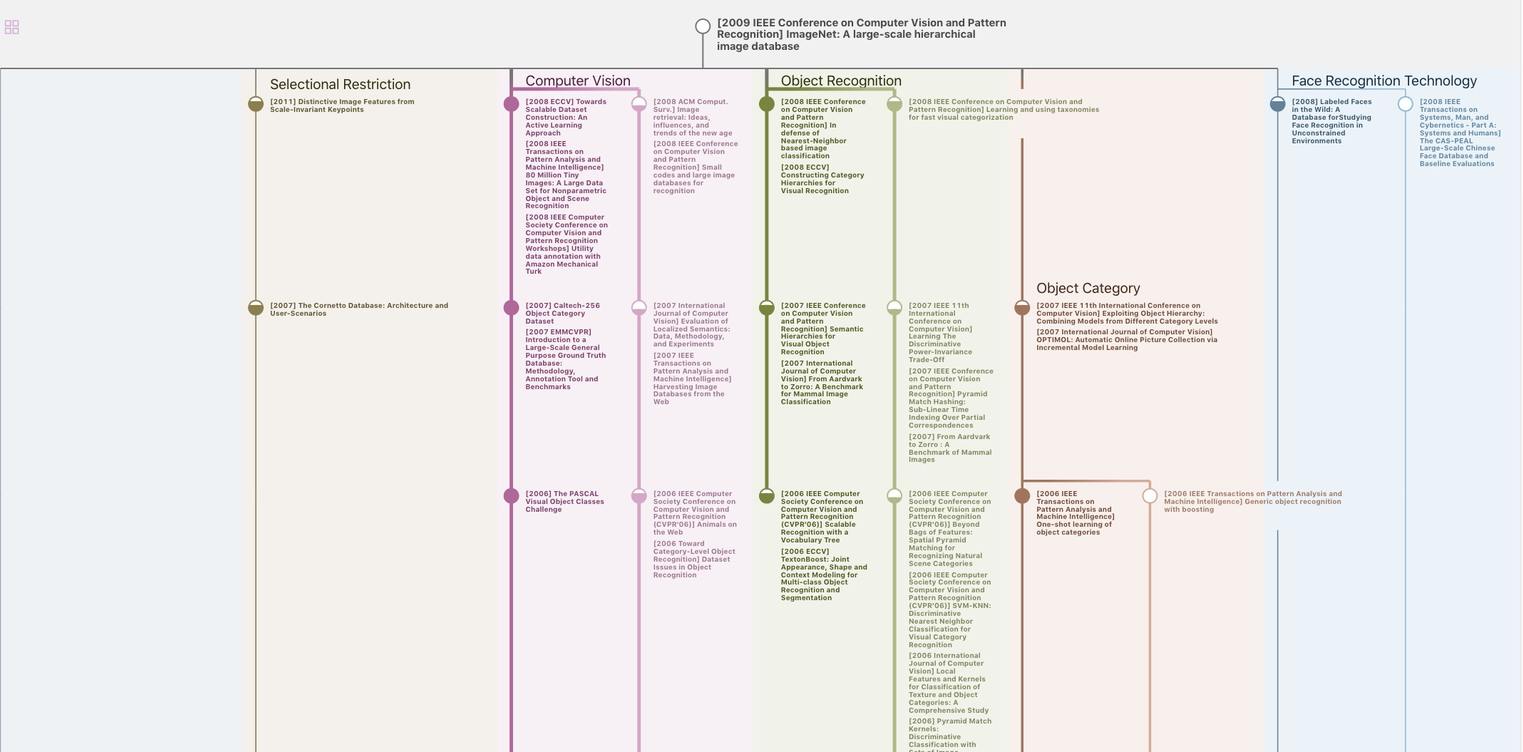
生成溯源树,研究论文发展脉络
Chat Paper
正在生成论文摘要