DelvMap: Completing Residential Roads in Maps Based on Couriers' Trajectories and Satellite Imagery.
IEEE Trans. Geosci. Remote. Sens.(2024)
摘要
The updated residential-level fine-grained digital map is essential for last-mile delivery. However, many of those low-level roads are not recorded in maps due to the high mapping costs. With the digitization of the logistics industry, couriers’ trajectories become a promising data source to complete missing roads in maps. Existing trajectory-based map updating work rely on heavy parameter tuning to overcome the positioning error issue due to their unsupervised nature, and are not able to handle issues of unreliable road indicators and skewed data distributions. To tackle those challenges, in this article, we propose a framework DelvMap to complete missing roads in maps based on couriers’ trajectories and satellite images. DelvMap first leverages a dual signal fusion network (DSFNet) to extract an inferred map from both data modalities, which fully exploits the positive and negative signals in the satellite images to fuse with roads indicated from trajectories, then employs a map completion algorithm to complete the existing map with the inferred map, the connection strategy of which is adaptive to the number of traversing trajectories. Experiments show DelvMap outperforms baselines by at least 11.0% in TOPO F1 on the real-world dataset. Finally, we demonstrate a multimodal map updating system based on DelvMap.
更多查看译文
关键词
Delivery data mining,map updating,multimodal learning
AI 理解论文
溯源树
样例
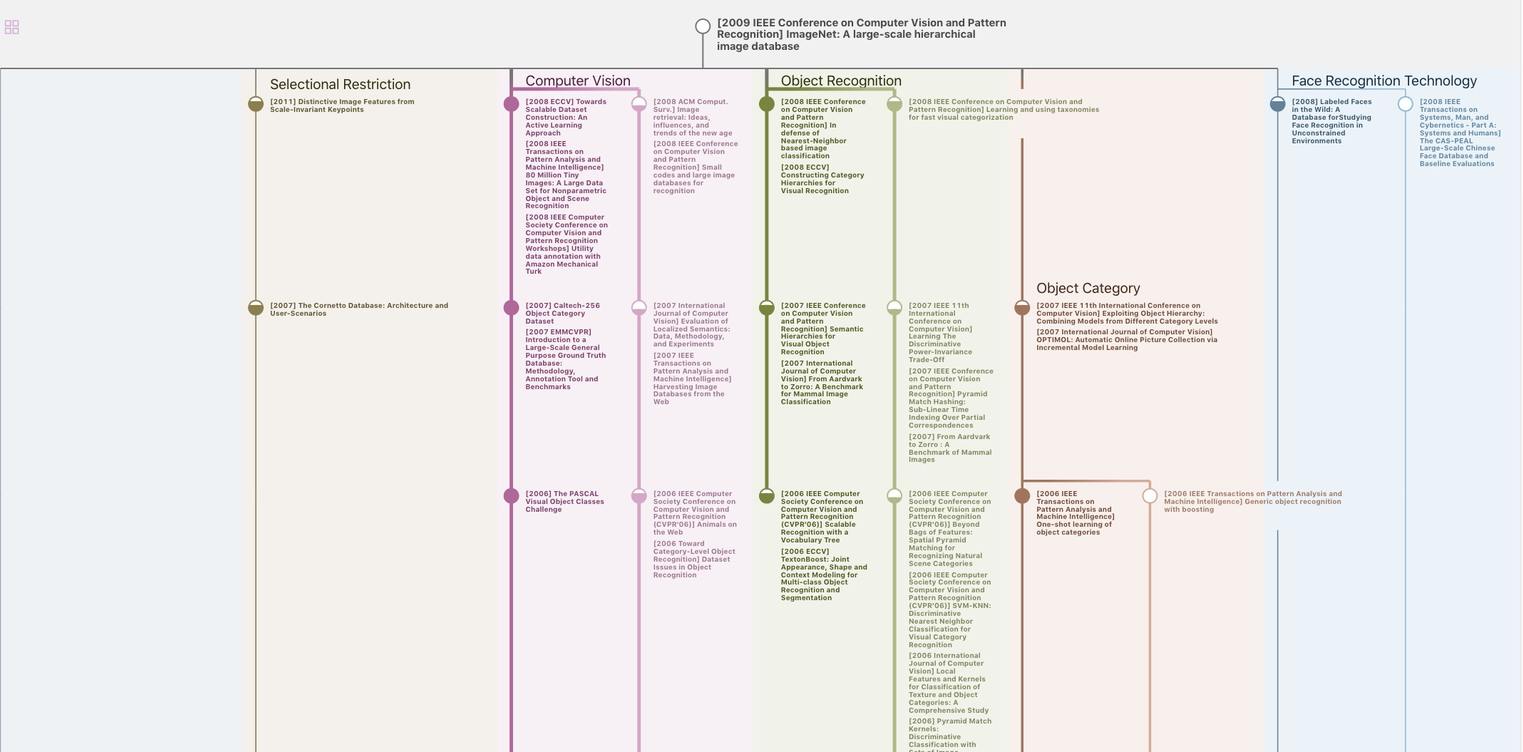
生成溯源树,研究论文发展脉络
Chat Paper
正在生成论文摘要