SEMI Net: Seismic-Electromagnetic Joint Inversion Network.
IEEE Trans. Geosci. Remote. Sens.(2024)
摘要
Inversion of seismic data, particularly full waveform inversion (FWI), allows for high-resolution subsurface velocity estimation. However, the inversion of subsurface velocities using only seismic data typically involves severe nonuniqueness. Electromagnetic exploration, due to its broad detection range and low cost, can effectively complement seismic exploration. Although electromagnetic data give a lower resolution resistivity information, they are sensitive to subsurface anomalies. Hence, the joint inversion of electromagnetic and seismic data effectively integrates the complementary information in both datasets to reduce the inversion nonuniqueness to improve accuracy and reliability of the inversion results. Nevertheless, current joint inversion techniques are confronted with issues such as the complexity of objective function design, challenges in achieving convergence, and insufficient coupling between seismic and electromagnetic data. To address these challenges, we propose a seismic-electromagnetic joint inversion network (SEMI Net) based on joint learning. Our approach leverages the powerful nonlinear fitting capabilities of neural networks for efficient multiobjective optimization. Moreover, we establish coupling between seismic and electromagnetic data across multiple sampling scales. Harnessing the frequency band complementarity of seismic and electromagnetic data, i.e., the low-frequency of the electromagnetic data and the mid-to-high frequency of the seismic data, we obtain high-resolution resistivity and velocity models by SEMI Net. Results on synthetic data and the Overthrust model demonstrate the effectiveness of our approach.
更多查看译文
关键词
Deep learning,electromagnetic inversion,full waveform inversion (FWI),joint inversion
AI 理解论文
溯源树
样例
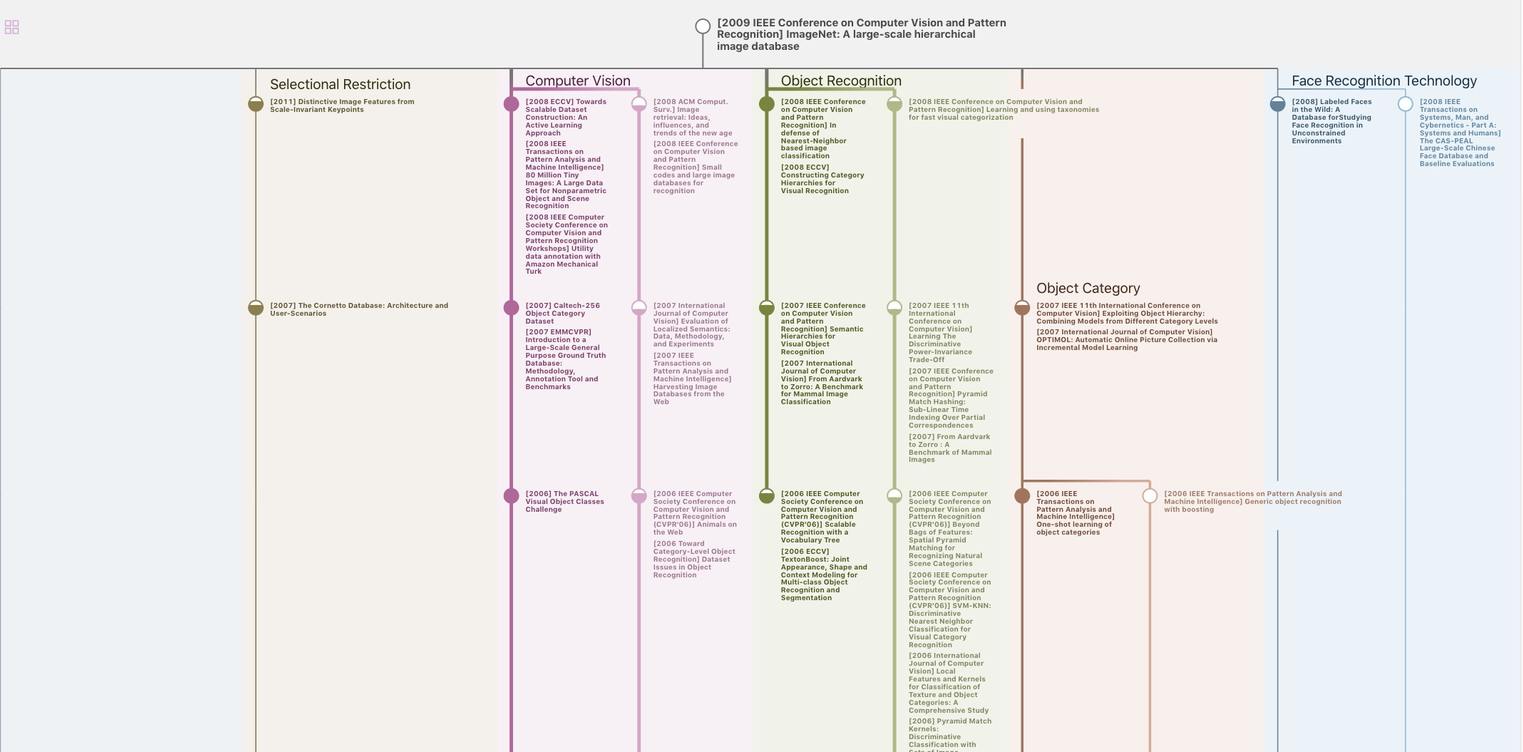
生成溯源树,研究论文发展脉络
Chat Paper
正在生成论文摘要