CSINet: A Cross-Scale Interaction Network for Lightweight Image Super-Resolution
SENSORS(2024)
摘要
In recent years, advancements in deep Convolutional Neural Networks (CNNs) have brought about a paradigm shift in the realm of image super-resolution (SR). While augmenting the depth and breadth of CNNs can indeed enhance network performance, it often comes at the expense of heightened computational demands and greater memory usage, which can restrict practical deployment. To mitigate this challenge, we have incorporated a technique called factorized convolution and introduced the efficient Cross-Scale Interaction Block (CSIB). CSIB employs a dual-branch structure, with one branch extracting local features and the other capturing global features. Interaction operations take place in the middle of this dual-branch structure, facilitating the integration of cross-scale contextual information. To further refine the aggregated contextual information, we designed an Efficient Large Kernel Attention (ELKA) using large convolutional kernels and a gating mechanism. By stacking CSIBs, we have created a lightweight cross-scale interaction network for image super-resolution named "CSINet". This innovative approach significantly reduces computational costs while maintaining performance, providing an efficient solution for practical applications. The experimental results convincingly demonstrate that our CSINet surpasses the majority of the state-of-the-art lightweight super-resolution techniques used on widely recognized benchmark datasets. Moreover, our smaller model, CSINet-S, shows an excellent performance record on lightweight super-resolution benchmarks with extremely low parameters and Multi-Adds (e.g., 33.82 dB@Set14 x 2 with only 248 K parameters).
更多查看译文
关键词
super-resolution,cross-scale interaction,factorized convolution,efficient large convolutional kernel attention
AI 理解论文
溯源树
样例
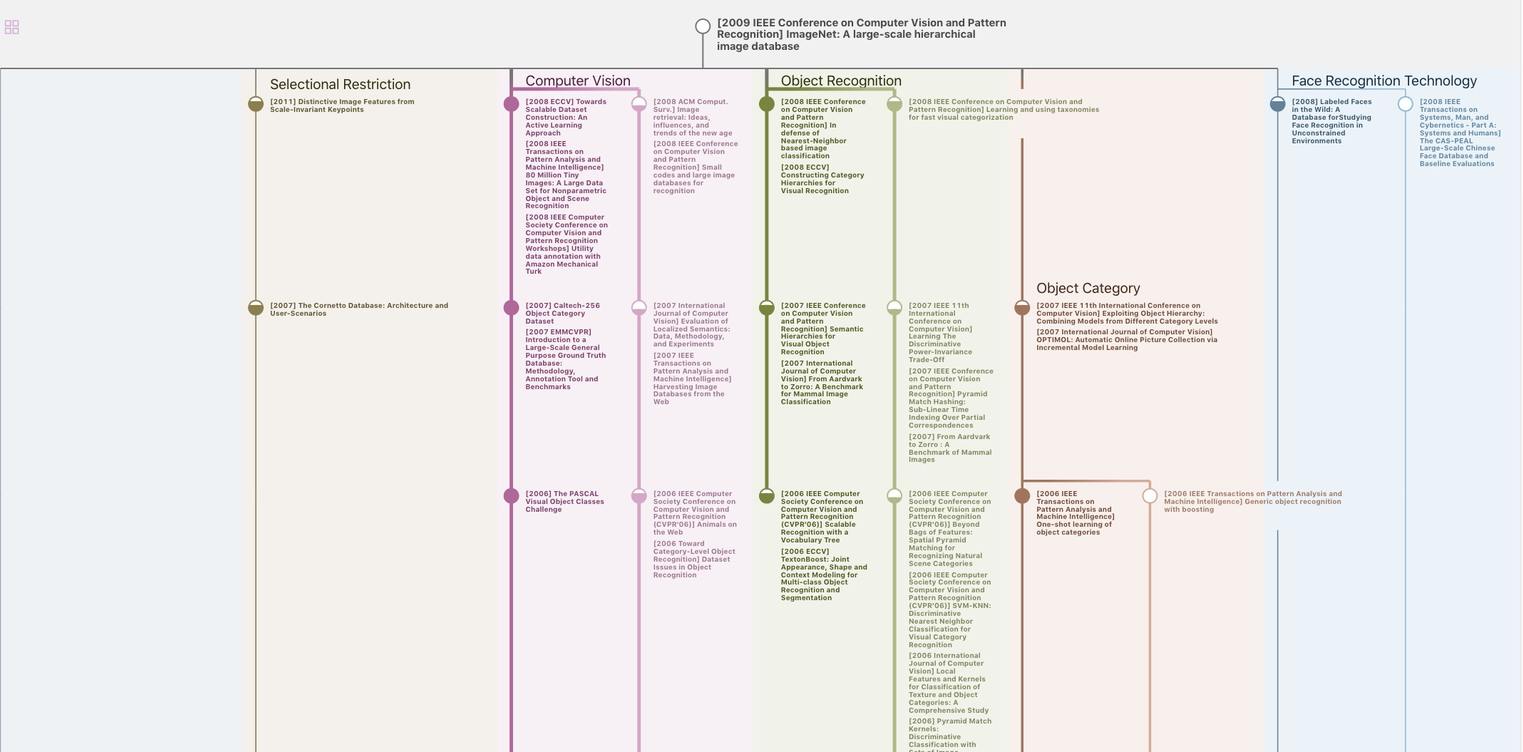
生成溯源树,研究论文发展脉络
Chat Paper
正在生成论文摘要