Lightweight Multilevel Feature-Fusion Network for Built-Up Area Mapping from Gaofen-2 Satellite Images
REMOTE SENSING(2024)
摘要
The timely, accurate acquisition of geographic spatial information such as the location, scope, and distribution of built-up areas is of great importance for urban planning, management, and decision-making. Due to the diversity of target features and the complexity of spatial layouts, the large-scale mapping of urban built-up areas using high-resolution (HR) satellite imagery still faces considerable challenges. To address this issue, this study adopted a block-based processing strategy and constructed a lightweight multilevel feature-fusion (FF) convolutional neural network for the feature representation and discrimination of built-up areas in HR images. The proposed network consists of three feature extraction modules composed of lightweight convolutions to extract features at different levels, which are further fused sequentially through two attention-based FF modules. Furthermore, to improve the problem of incorrect discrimination and severe jagged boundaries caused by block-based processing, a majority voting method based on a grid offset is adopted to achieve a refined extraction of built-up areas. The effectiveness of this method is evaluated using Gaofen-2 satellite image data covering Shenzhen, China. Compared with several state-of-the-art algorithms for detecting built-up areas, the proposed method achieves a higher detection accuracy and preserves better shape integrity and boundary smoothness in the extracted results.
更多查看译文
关键词
built-up area,high resolution,satellite image,CNN
AI 理解论文
溯源树
样例
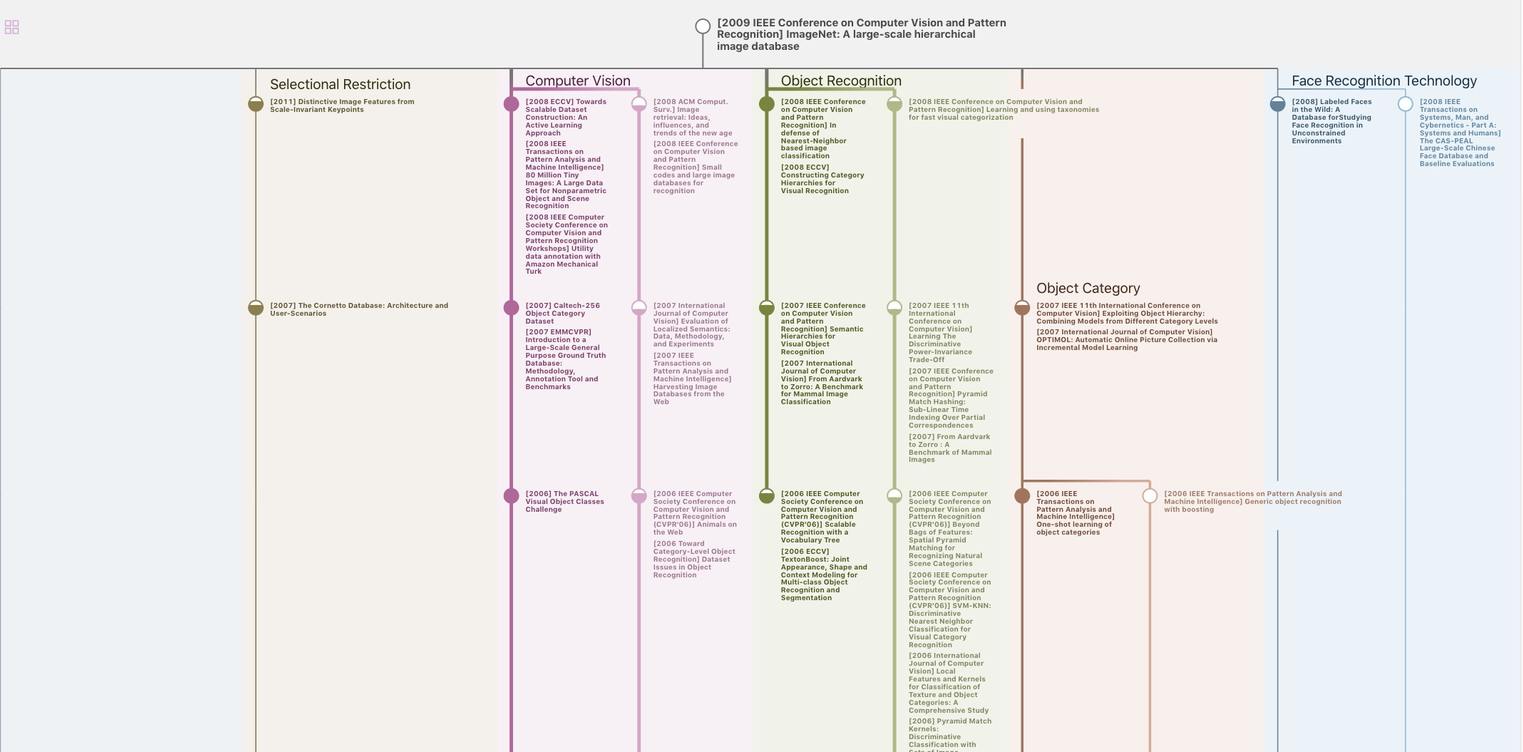
生成溯源树,研究论文发展脉络
Chat Paper
正在生成论文摘要