Multiscale Feature Extraction U-Net for Infrared Dim- and Small-Target Detection
REMOTE SENSING(2024)
摘要
The technology of infrared dim- and small-target detection is irreplaceable in many fields, such as those of missile early warning systems and forest fire prevention, among others. However, numerous components interfere with infrared imaging, presenting challenges for achieving successful detection of infrared dim and small targets with a low rate of false alarms. Hence, we propose a new infrared dim- and small-target detection network, Multiscale Feature Extraction U-Net for Infrared Dim- and Small-Target Detection (MFEU-Net), which can accurately detect targets in complex backgrounds. It uses the U-Net structure, and the encoders and decoders consist of ReSidual U-block and Inception, allowing rich multiscale feature information to be extracted. Thus, the effectiveness of algorithms in detecting very small-sized targets can be improved. In addition, through the multidimensional channel and spatial attention mechanism, the model can be adjusted to focus more on the target area in the image, improving its extraction of target information and detection performance in different scenarios. The experimental results show that our proposed algorithm outperforms other advanced algorithms in detection performance. On the MFIRST, SIRST, and IRSTD-1k datasets, we achieved detection rates of 0.864, 0.962, and 0.965; IoU values of 0.514, 0.671, and 0.630; and false alarm rates of 3.08 x 10-5, 2.61 x 10-6, and 1.81 x 10-5, respectively.
更多查看译文
关键词
convolutional neural network,multiscale features,infrared image,small-target detection
AI 理解论文
溯源树
样例
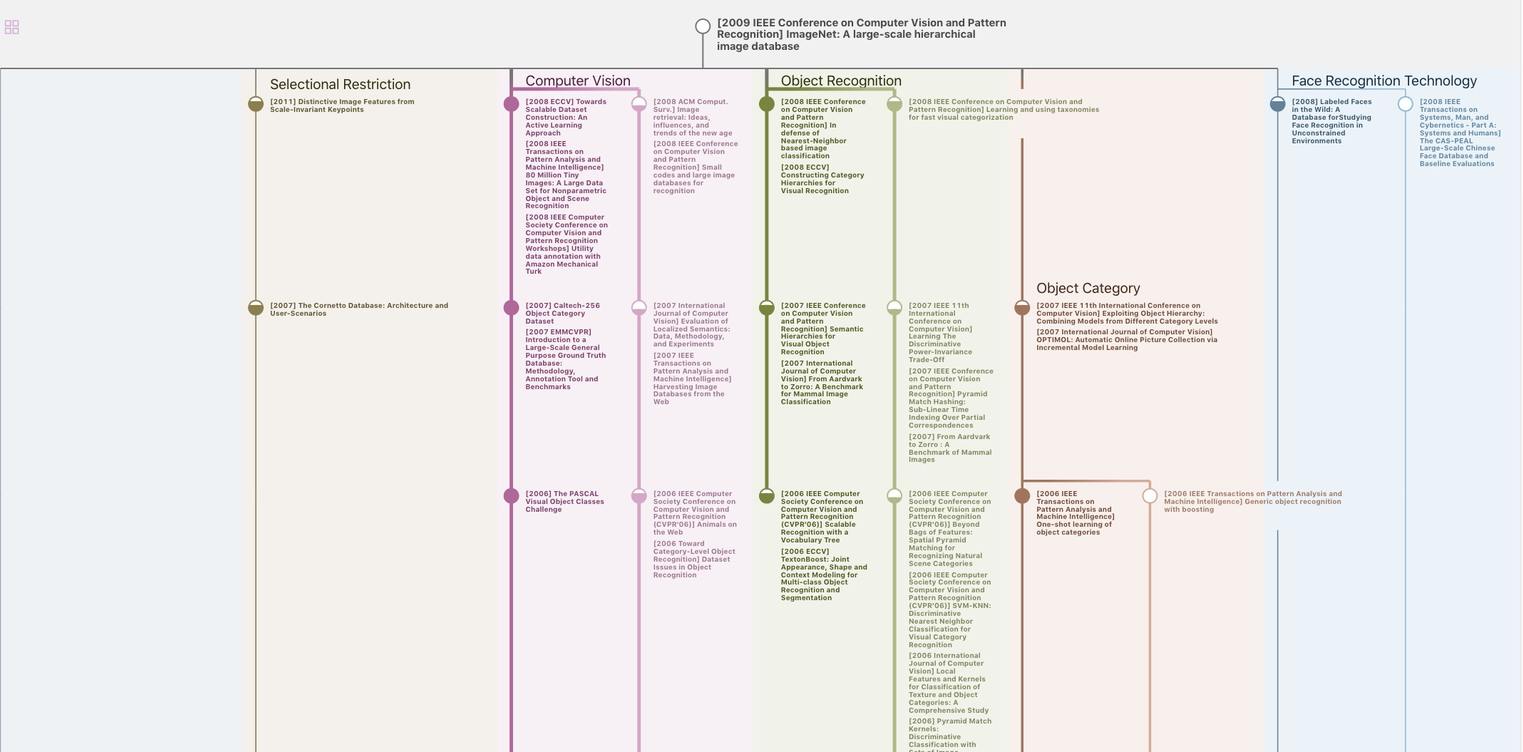
生成溯源树,研究论文发展脉络
Chat Paper
正在生成论文摘要