Domain generalization for video anomaly detection considering diverse anomaly types
Signal, Image and Video Processing(2024)
摘要
In intelligent video surveillance, anomaly detection is conducted to identify the occurrence of abnormal events by monitoring the video captured by vision sensors, and it has important application value in public safety, industrial production process monitoring, and other fields. However, the study of video anomaly detection (VAD) models that generalize into uncharted territory remains challenging. The current approach is to use more abnormal samples to enhance the training and improve the generalization ability of the model, but this requires a large number of auxiliary datasets to fully describe the abnormal events. In addition, the ambiguity of the abnormality definition makes it impossible to effectively cover all abnormal videos. To solve this problem, we divide abnormalities into three different types based on object and behavior type: normal object and abnormal behavior (NOAB), abnormal object and normal behavior (AONB), and abnormal object and abnormal behavior (AOAB). We find that the traditional prediction-based model shows better generalization ability for NOAB. However, under the domain generalization setting, the generalization effect of AONB and AOAB decreases significantly. To solve this problem, a new spatiotemporal generalization (STG) model is proposed specifically for the detection of AONB and AOAB events involving anomalous objects. The STG model integrates contrast learning and adaptive data augmentation techniques to realize domain expansion. In addition, combining the STG model with the traditional prediction-based model, an anomaly video monitoring framework is proposed to comprehensively detect anomalies without target domain adaptation, which can improve the generalization ability of VAD models without auxiliary datasets. Extensive evaluations show that the proposed method achieves excellent performance on the benchmark datasets under the domain generalization setting.
更多查看译文
关键词
Video anomaly detection,Domain generalization,Unsupervised learning,Domain expansion
AI 理解论文
溯源树
样例
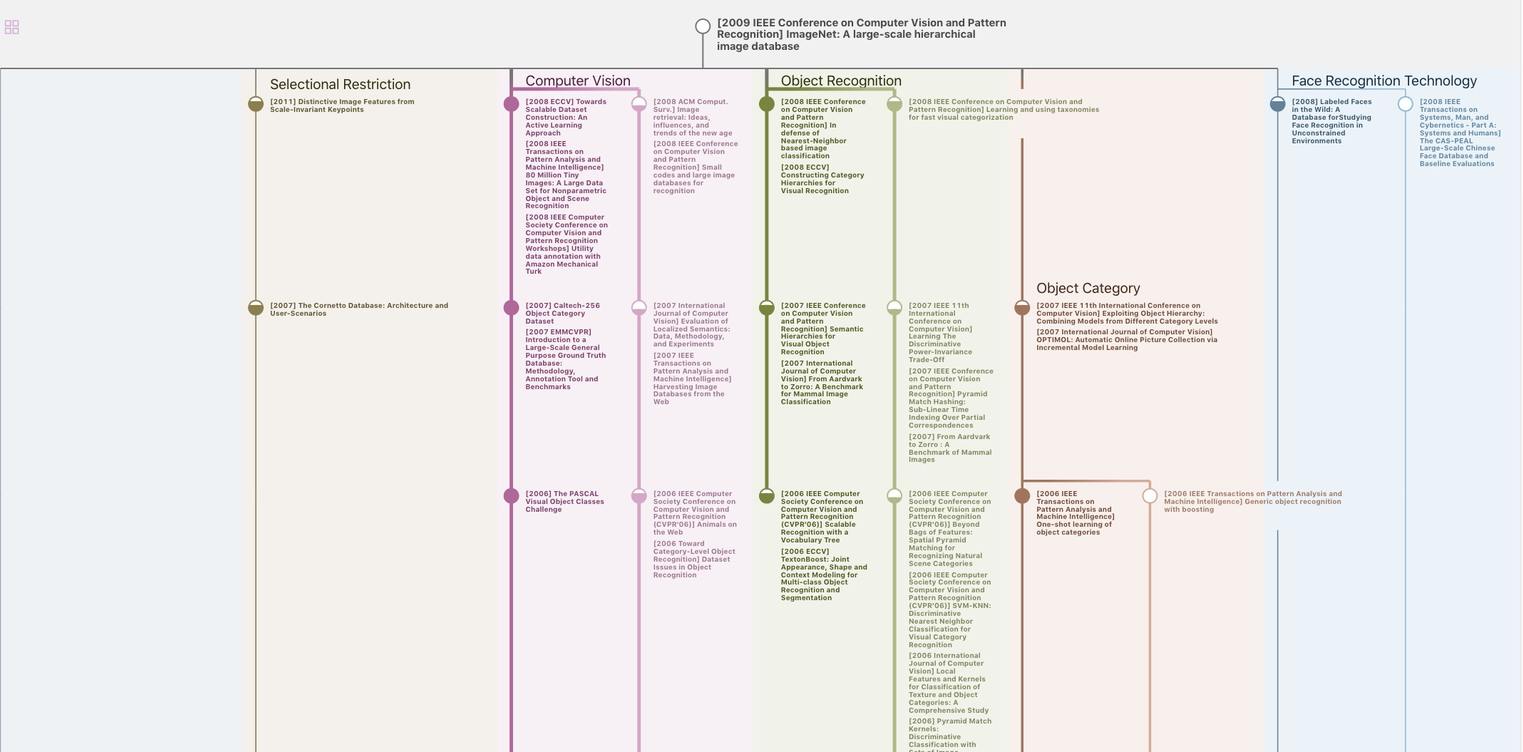
生成溯源树,研究论文发展脉络
Chat Paper
正在生成论文摘要