Learning dynamic representations of the functional connectome in neurobiological networks.
ArXiv(2024)
摘要
The static synaptic connectivity of neuronal circuits stands in direct contrast to the dynamics of their function. As in changing community interactions, different neurons can participate actively in various combinations to effect behaviors at different times. We introduce an unsupervised approach to learn the dynamic affinities between neurons in live, behaving animals, and to reveal which communities form among neurons at different times. The inference occurs in two major steps. First, pairwise non-linear affinities between neuronal traces from brain-wide calcium activity are organized by non-negative tensor factorization (NTF). Each factor specifies which groups of neurons are most likely interacting for an inferred interval in time, and for which animals. Finally, a generative model that allows for weighted community detection is applied to the functional motifs produced by NTF to reveal a dynamic functional connectome. Since time codes the different experimental variables (e.g., application of chemical stimuli), this provides an atlas of neural motifs active during separate stages of an experiment (e.g., stimulus application or spontaneous behaviors). Results from our analysis are experimentally validated, confirming that our method is able to robustly predict causal interactions between neurons to generate behavior.
更多查看译文
关键词
neural networks,non-negative tensor factorization,dynamic community detection,functional connectome
AI 理解论文
溯源树
样例
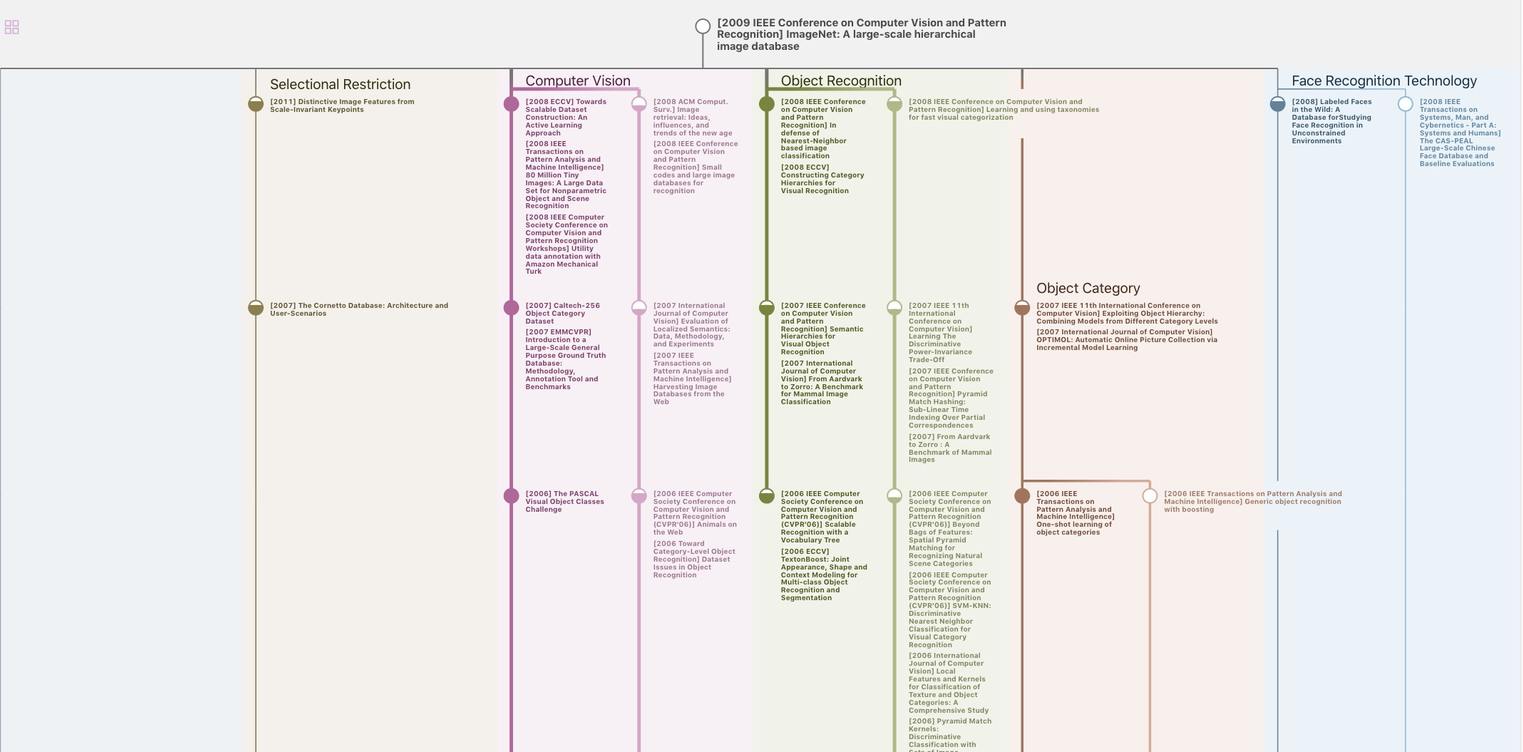
生成溯源树,研究论文发展脉络
Chat Paper
正在生成论文摘要