Investigating the impact of inflammatory response-related genes on renal fibrosis diagnosis: a machine learning-based study with experimental validation
JOURNAL OF BIOMOLECULAR STRUCTURE & DYNAMICS(2024)
摘要
Renal fibrosis plays a crucial role in the progression of renal diseases, yet the lack of effective diagnostic markers poses challenges in scientific and clinical practices. In this study, we employed machine learning techniques to identify potential biomarkers for renal fibrosis. Utilizing two datasets from the GEO database, we applied LASSO, SVM-RFE and RF algorithms to screen for differentially expressed genes related to inflammatory responses between the renal fibrosis group and the control group. As a result, we identified four genes (CCL5, IFITM1, RIPK2, and TNFAIP6) as promising diagnostic indicators for renal fibrosis. These genes were further validated through in vivo experiments and immunohistochemistry, demonstrating their utility as reliable markers for assessing renal fibrosis. Additionally, we conducted a comprehensive analysis to explore the relationship between these candidate biomarkers, immunity, and drug sensitivity. Integrating these findings, we developed a nomogram with a high discriminative ability, achieving a concordance index of 0.933, enabling the prediction of disease risk in patients with renal fibrosis. Overall, our study presents a predictive model for renal fibrosis and highlights the significance of four potential biomarkers, facilitating clinical diagnosis and personalized treatment. This finding presents valuable insights for advancing precision medicine approaches in the management of renal fibrosis.Communicated by Ramaswamy H. Sarma
更多查看译文
关键词
Renal fibrosis,inflammatory response,immune cell infiltration,diagnostic markers,machine learning
AI 理解论文
溯源树
样例
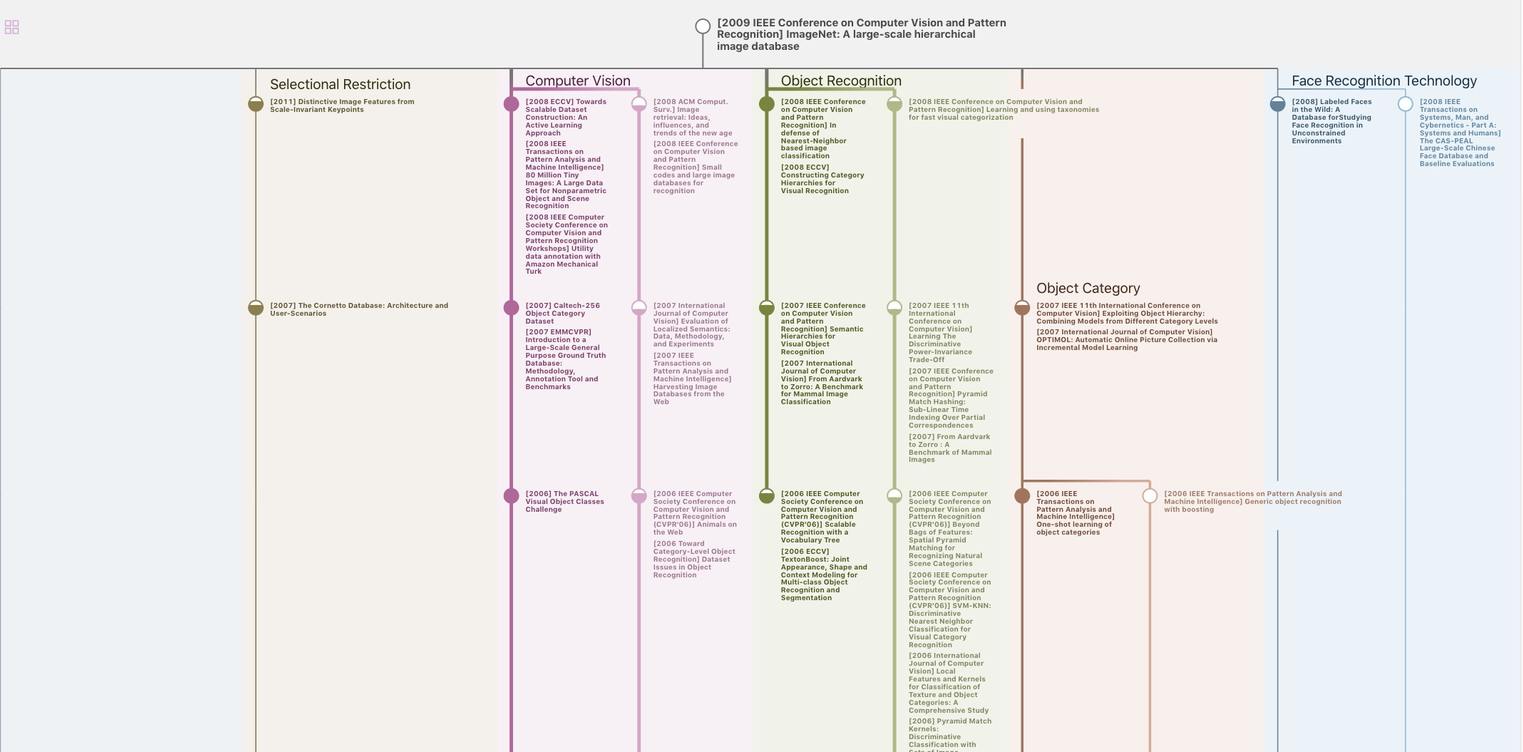
生成溯源树,研究论文发展脉络
Chat Paper
正在生成论文摘要