MLSTL-WSN: machine learning-based intrusion detection using SMOTETomek in WSNs
International Journal of Information Security(2024)
摘要
In the domain of cyber-physical systems, wireless sensor networks (WSNs) play a pivotal role as infrastructures, encompassing both stationary and mobile sensors. These sensors self-organize and establish multi-hop connections for communication, collectively sensing, gathering, processing, and transmitting data about their surroundings. Despite their significance, WSNs face rapid and detrimental attacks that can disrupt functionality. Existing intrusion detection methods for WSNs encounter challenges such as low detection rates, computational overhead, and false alarms. These issues stem from sensor node resource constraints, data redundancy, and high correlation within the network. To address these challenges, we propose an innovative intrusion detection approach that integrates machine learning (ML) techniques with the Synthetic Minority Oversampling Technique Tomek Link (SMOTE-TomekLink) algorithm. This blend synthesizes minority instances and eliminates Tomek links, resulting in a balanced dataset that significantly enhances detection accuracy in WSNs. Additionally, we incorporate feature scaling through standardization to render input features consistent and scalable, facilitating more precise training and detection. To counteract imbalanced WSN datasets, we employ the SMOTE-Tomek resampling technique, mitigating overfitting and underfitting issues. Our comprehensive evaluation, using the wireless sensor network dataset (WSN-DS) containing 374,661 records, identifies the optimal model for intrusion detection in WSNs. The standout outcome of our research is the remarkable performance of our model. In binary classification scenarios, it achieves an accuracy rate of 99.78
更多查看译文
关键词
Wireless sensor network,Intrusion detection,Machine learning,SMOTE,Sensor nodes
AI 理解论文
溯源树
样例
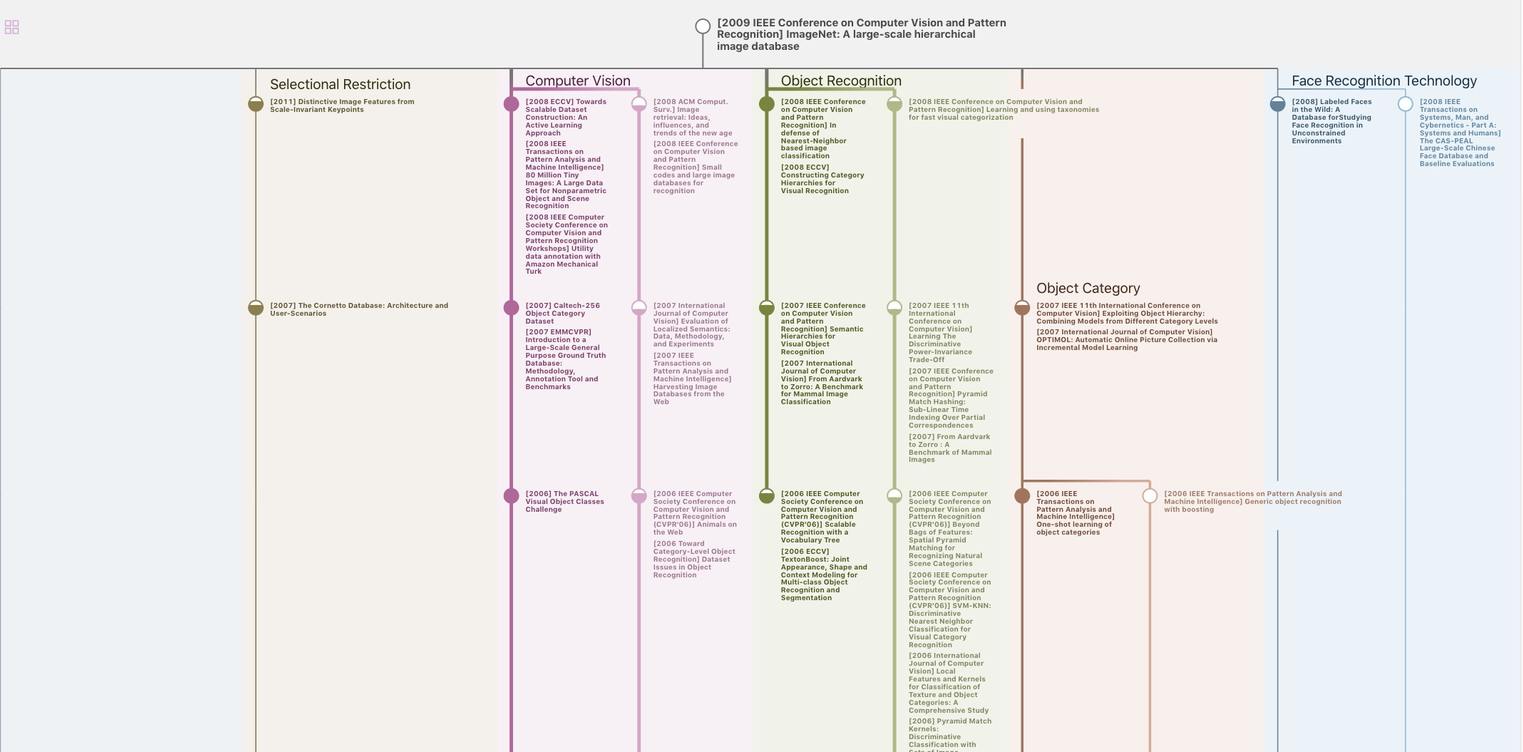
生成溯源树,研究论文发展脉络
Chat Paper
正在生成论文摘要