Neural density estimation for Galactic Binaries in LISA data analysis
arxiv(2024)
摘要
The future space based gravitational wave detector LISA (Laser Interferometer Space Antenna) will observe millions of Galactic binaries constantly present in the data stream. A small fraction of this population (of the order of several thousand) will be individually resolved. One of the challenging tasks from the data analysis point of view will be to estimate the parameters of resolvable galactic binaries while disentangling them from each other and from other gravitational wave sources present in the data. This problem is quite often referred to as a global fit in the field of LISA data analysis. A Bayesian framework is often used to infer the parameters of the sources and their number. The efficiency of the sampling techniques strongly depends on the proposals, especially in the multi-dimensional parameter space. In this paper we demonstrate how we can use neural density estimators, and in particular Normalising flows, in order to build proposals which significantly improve the convergence of sampling. We also demonstrate how these methods could help in building priors based on physical models and provide an alternative way to represent the catalogue of identified gravitational wave sources.
更多查看译文
AI 理解论文
溯源树
样例
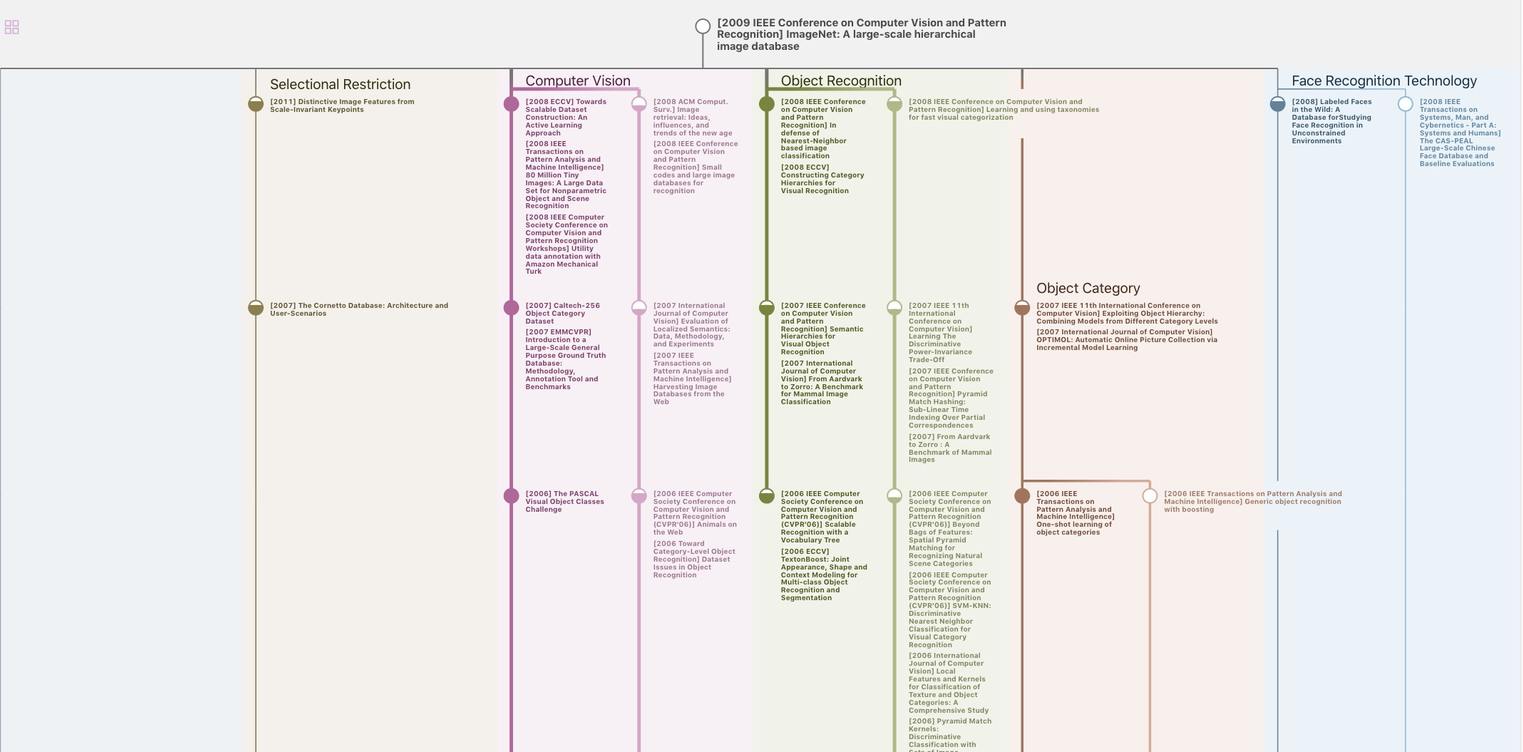
生成溯源树,研究论文发展脉络
Chat Paper
正在生成论文摘要