Deep image matting with cross-layer contextual information propagation
Neural Computing and Applications(2024)
摘要
Natural image matting focuses on accurately estimating the opacity of the foreground object in an arbitrary background. Recently, deep learning-based approaches made significant progress in the matting task benefit from their powerful learning ability for semantic features. However, artifacts, blurry structures, and miscalculated pixels still often appear in some difficult regions with background interference and complex details. To address the above issues, we propose a cross-layer contextual information propagation mechanism (CCIP) that can explicitly model the long-range correlations between global and unknown regions. Specifically, we first calculate region affinity at high-level features with rich structure and semantic information; then reconstruct the adjacent low-level features by propagating information from the global region to the unknown region under the guidance of the affinity matrix; finally, transfer the reconstructed information to the corresponding decoder stage to further improve the feature distinctiveness. In addition, we design a simple and effective supervision strategy in a deep-to-shallow manner to gradually optimize the edges and details of the foreground object. We conducted extensive experiments on the common dataset Composition-1k, the alphamatting.com benchmark, and some real-world images. Compared with previous methods, the proposed method achieves competitive performance on the Composition-1k dataset (30.3 on SAD, 6.8 on MSE, 13.3 on Grad, and 26.7 on Con) and alphamatting.com benchmark (17 on average SAD rank and 16.8 on average Grad rank), while simultaneously yielding high-quality matting results on real-world images.
更多查看译文
关键词
Natural image matting,Contextual information propagation,Long-range correlation,Supervision strategy
AI 理解论文
溯源树
样例
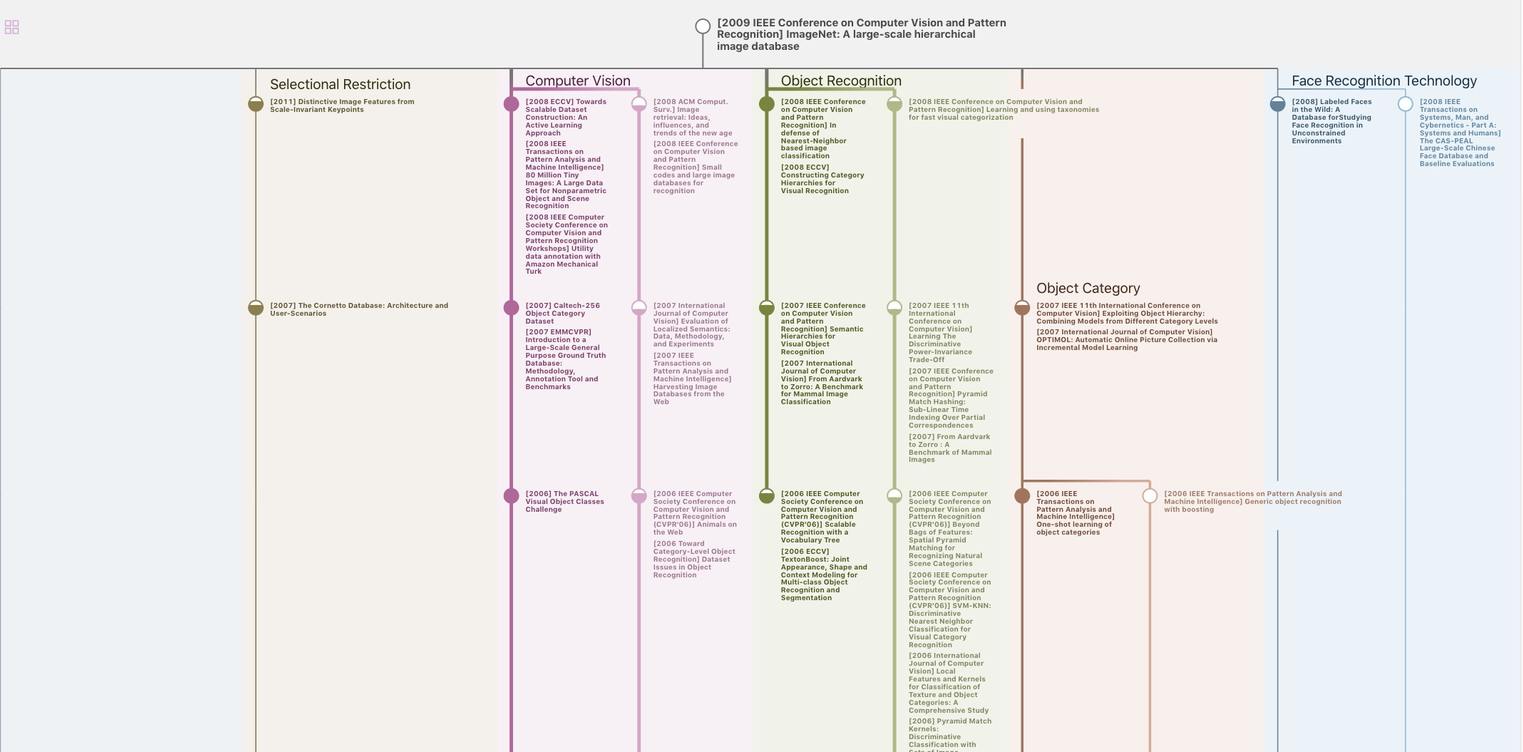
生成溯源树,研究论文发展脉络
Chat Paper
正在生成论文摘要