Tree-Planted Transformers: Large Language Models with Implicit Syntactic Supervision
CoRR(2024)
摘要
Large Language Models (LLMs) have achieved remarkable success thanks to
scalability on large text corpora, but have some drawback in training
efficiency. In contrast, Syntactic Language Models (SLMs) can be trained
efficiently to reach relatively high performance thanks to syntactic
supervision, but have trouble with scalability. Thus, given these complementary
advantages of LLMs and SLMs, it is necessary to develop an architecture that
integrates the scalability of LLMs with the training efficiency of SLMs, namely
Syntactic Large Language Models (SLLM). In this paper, we propose a novel
method dubbed tree-planting: implicitly "plant" trees into attention weights of
Transformer LMs to reflect syntactic structures of natural language.
Specifically, Transformer LMs trained with tree-planting will be called
Tree-Planted Transformers (TPT), which learn syntax on small treebanks via
tree-planting and then scale on large text corpora via continual learning with
syntactic scaffolding. Targeted syntactic evaluations on the SyntaxGym
benchmark demonstrated that TPTs, despite the lack of explicit syntactic
supervision, significantly outperformed various SLMs with explicit syntactic
supervision that generate hundreds of syntactic structures in parallel,
suggesting that tree-planting and TPTs are the promising foundation for SLLMs.
更多查看译文
AI 理解论文
溯源树
样例
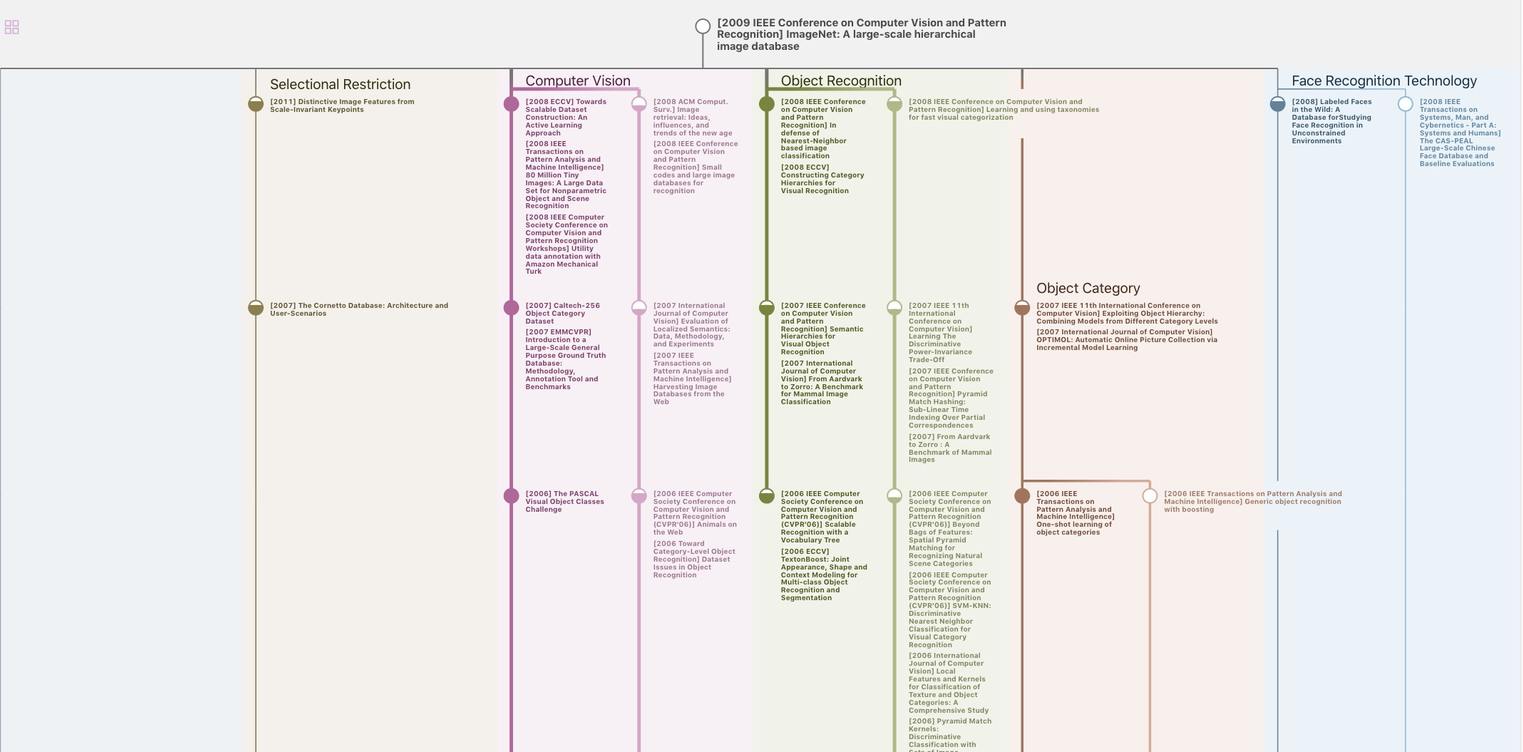
生成溯源树,研究论文发展脉络
Chat Paper
正在生成论文摘要