Underwater image enhancement using lightweight vision transformer
Multimedia Tools and Applications(2024)
摘要
Deep learning-based models have recently shown a strong potential in Underwater Image Enhancement (UIE) that are satisfying and have the right colors and details, but these methods significantly increase the parameters and complexity of the image processing models and therefore cannot be deployed directly to the edge devices. Vision Transformers (ViT) based architectures have recently produced amazing results in many vision tasks such as image classification, super-resolution, and image restoration. In this study, we introduced a lightweight Context-Aware Vision Transformer (CAViT), based on the Mean Head tokenization strategy and uses a self-attention mechanism in a single branch module that is effective at simulating long-distance dependencies and global features. To further improve the image quality we proposed an efficient variant of our model which derived results by applying White Balancing and Gamma Correction methods. We evaluated our model on two standard datasets, i.e., Large-Scale Underwater Image (LSUI) and Underwater Image Enhancement Benchmark Dataset (UIEB), which subsequently contributed towards more generalized results. Overall findings indicate that our real-time UIE model outperforms other Deep Learning based models by reducing the model complexity and improving the image quality (i.e., 0.6 dB PSNR improvement while using only 0.3
更多查看译文
关键词
Tokenization,Feature extraction,Image enhancement,Vision transformers
AI 理解论文
溯源树
样例
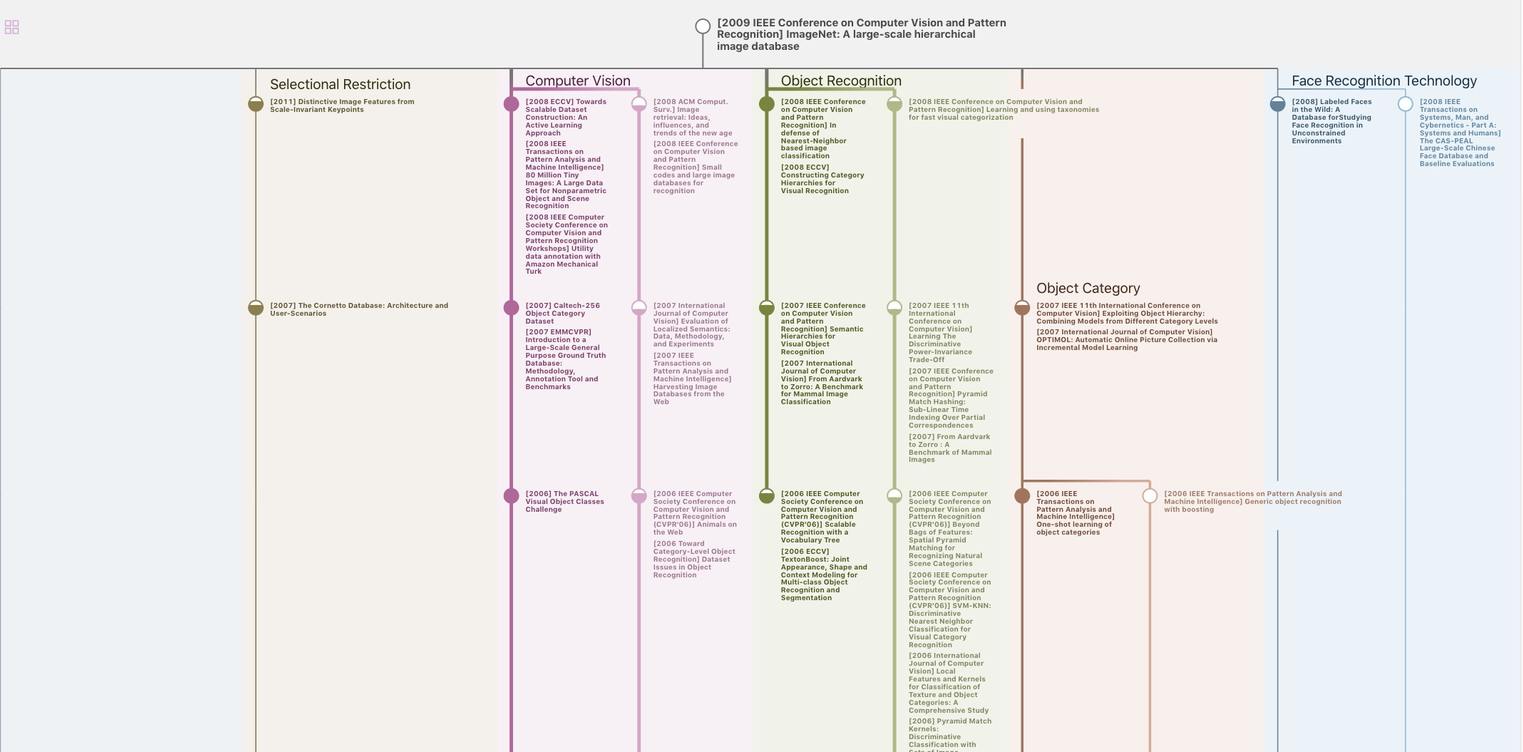
生成溯源树,研究论文发展脉络
Chat Paper
正在生成论文摘要